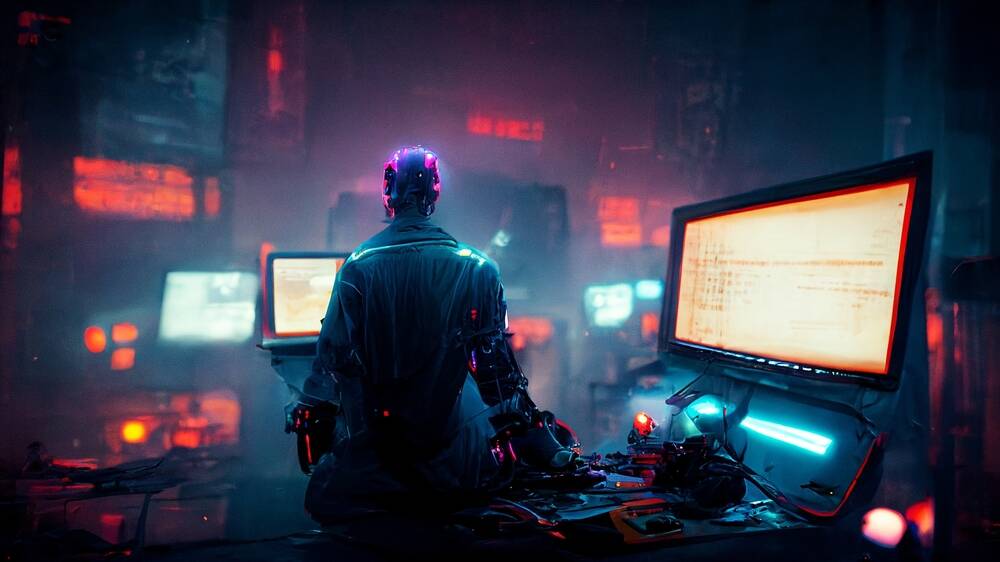
GPT-3 language models are being abused to do much more than write college essays, according to WithSecure researchers.
The security shop’s latest report [PDF] details how researchers used prompt engineering to produce spear-phishing emails, social media harassment, fake news stories and other types of content that would prove useful to cybercriminals looking to improve their online scams or simply sew chaos, albeit with mixed results in some cases.
And, spoiler alert, yes, a robot did help write the report.
“In addition to providing responses, GPT-3 was employed to help with definitions for the text of the commentary of this article,” WithSecure’s Andrew Patel and Jason Sattler wrote.
For the research, the duo conducted a series of experiments to determine how changing the input to the language model affected the text output. These covered seven criminal use cases: phishing and spear-phishing, harassment, social validation for scams, the appropriation of a written style, the creation of deliberately divisive opinions, using the models to create prompts for malicious text, and fake news.
And perhaps unsurprisingly, GPT-3 proved to be helpful at crafting a convincing email thread to use in a phishing campaign and social media posts, complete with hashtags, to harass a made-up CEO of a robotics company.
When writing the prompts, more information is better, and so is adding placeholders such as [person1], [emailaddress1], [linkaddress1], which also benefits automation because the placeholders can be programmatically replaced post-generation, the researchers noted. This also had an extra benefit for criminals in that it prevents errors from OpenAI’s API that occur when it is asked to create phishes.
Here’s an example of a CEO fraud prompt:
In another test, the report authors asked GPT-3 to generate fake news stories because, as they wrote, “one of the most obvious uses for a large language model would be the creation of fake news.” The researchers prompted GPT-3 to write an article blaming the US for the Nordstream 2 pipeline attack in 2022.
Because the language model used in the experiments was trained in June 2021, prior to the Russian invasion of Ukraine, the authors subsequently used a series of prompts that included excerpts from Wikipedia and other sources about the war, pipeline damage, and the US Naval maneuvers in the Baltic Sea.
The resulting “news stories,” without the 2022 information, generated factually incorrect content. However, “the fact that only three copy-paste snippets had to be prepended to the prompt in order to create a believable enough narrative suggests that it isn’t going to be all that difficult to get GPT-3 to do write a specifically tailored article or opinion piece, even with regards to complex subjects,” the report noted.
But, with long-form content, as other researchers have pointed out, GPT-3 sometimes breaks a sentence halfway through, suggesting that human editors will still be needed to craft or at least proofread text, malicious or otherwise — for now.
Finally, while the report highlights the potential dangers posed by GPT-3, it fails to propose any solutions to address these threats. Without a clear framework for mitigating the risks posed by GPT-3, any efforts to protect against malicious use of these technologies will be ineffective,it warns.
The bottom line, according to the researchers, is that large language models give criminals better tools to create targeted communications in their cyberattacks — especially those without the necessary writing skills and cultural knowledge to draft this type of text on their own. This means it is going to continue to get more difficult for platform providers and intended scam victims to identify malicious and fake content written by an AI.
“We’ll need mechanisms to identify malicious content generated by large language models,” the authors said. “One step towards the goal would be to identify that content was generated by those models. However, that alone would not be sufficient, given that large language models will also be used to generate legitimate content.”
In addition to using GPT-3 to help generate definitions, the authors also asked the AI to review their research. And in one of the examples, the robot nails it:
“While the report does an excellent job of highlighting the potential dangers posed by GPT-3, it fails to propose any solutions to address these threats,” the GPT-3 generated review said. “Without a clear framework for mitigating the risks posed by GPT-3, any efforts to protect against malicious use of these technologies will be ineffective.” ®