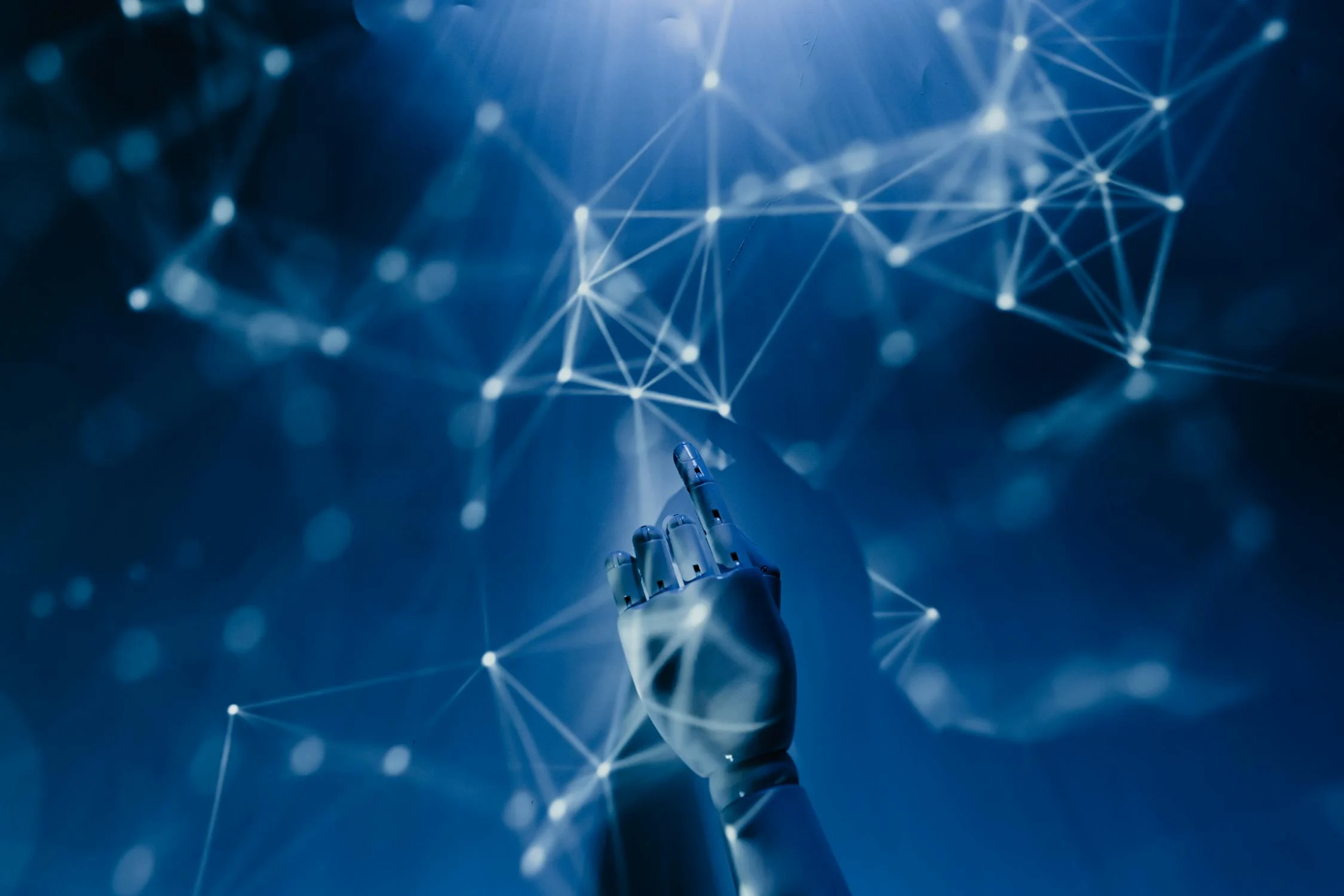
AI is one of the most transformative and valuable scientific tools ever developed. By harnessing vast amounts of data and computational power, AI systems can uncover patterns, generate insights, and make predictions that were previously unattainable.
Rick Stevens
Credit: Argonne National Laboratory
As we find ourselves on the cusp of an AI revolution, scientists are beginning to question how this technology can be best put to use in their research endeavors. More specifically, the Department of Energy (DoE) is investigating how best to use its vast array of computational resources to make AI a central tool in scientific research.
This effort has culminated in the creation of the Frontier AI for Science, Security and Technology (FASST) initiative. FASST will operate as a research and infrastructure development initiative with the goal of developing and deploying high-value AI systems for science.
Rick Stevens – the Associate Laboratory Director for Computing, Environment, and Life Sciences (CELS) and an Argonne Distinguished Fellow – discussed this push in a talk at the ISC2024 conference. During this discussion, he laid out the future of AI in science as well as some of the challenges that we’ll face along the way.
Flexible AI Will Accelerate Science
Stevens began by pointing out that the DoE is properly positioned to lead the charge in AI for science. The department has the massive machines necessary to do AI work and the human resources required to keep these systems functional.
He went on to describe a set of workshops that the DoE organized in the summer of 2022 to discuss how the department and its researchers should think about the AI revolution. The workshops eventually decided on six areas to represent important scientific endeavors to consolidate efforts in AI development:
- AI for advanced properties inference and inverse design: Energy storage, Proteins, Polymers, Stockpile modernization
- AI and robotics for autonomous discovery: Materials, chemistry, biology, light sources, neutrons
- AI-based surrogates for HPC: Climate ensembles, Exascale apps with surrogates, 1000x faster è zettascale now
- AI for software engineering and programming: Code Translation, Optimization, quantum compilation, QAIgs
- AI for prediction and control of complex engineered systems: Accelerators, Buildings, Cities, Reactors, Power Grid, Networks
- Foundation, Assured AI for scientific knowledge: Hypothesis formation, Math theory, and modeling synthesis
Stevens was quick to point out that the scientific community needs to start thinking about creating flexible models that can perform many functions.
“You could think of each one of these six areas as the conceptual target for something like a frontier foundational model,” Stevens said. “Not many models, not tiny models, not one model for every data set. The idea that for advanced property inference and inverse design is one model that spans all these other areas in the same way that ChatGPT is one model.”
This echoes some of the sentiments that came out of the DoE’s announcement of the creation of FASST at the AI Expo for National Competitiveness in Washington. Specifically, the DoE is hoping for the creation of flexible foundational models that can solve a variety of functions within a similar scientific field.
“Imagine we had a basic science AI foundational model like ChatGPT for English – but it speaks physics and chemistry,” Deputing Energy Secretary David Turk said while announcing the initiative.
In his talk, Stevens discussed that this flexibility will be absolutely necessary considering the fact that AI model parameter sizes are exploding. Argonne is already preparing for trillion parameter models, which demand an enormous amount of computing power.
Stevens stated that if a scientist wanted to train a trillion-parameter model on 20 trillion tokens of data using a 10-exaflop mixed-precision machine, it would take several months to complete. That’s a huge barrier that most organizations won’t be able to overcome, and as such scientists are already working to improve efficiency. Stevens mentioned pushing toward smaller models with high quality data as a solution, as well as lower complexity.
However, a truly interesting innovation that he brought up in his talk is the advent of AI assistants.
The Potential of AI Assistants
Stevens and other DoE scientists are working hard to develop AI assistants to get the most out of AI tools for scientific research. The idea is that researchers would build these assistants tailored to the specific kind of scientific research that they are working on.
“It works with you 24/7,” Stevens said. “You can email it, text it, video it, yell at it. It takes high-level instructions and works toward concrete goals, intuiting what you want. It checks in as needed, but just keeps working. And we’re trying to scope out how this might work.”
With such an exciting and revolutionary idea, the question remains as to how far away this technology is from becoming a reality. While Stevens mentioned a project called Astral that is working on this problem, he made a point to mention issues with trustworthiness when it comes to developing AI assistants.
Stevens showed an example where he asked Chat-GPT4 to write a Python program to numerically solve for some Drift-Diffusion Equations, which model charges and semiconductors – work that is essential for designing future computers.
Chat-GPT4 took these instructions and spit out a Python code that runs and gives you answers. But Stevens asked a very important question – who can check that? How many humans would we need to actually verify that Chat-GPT gave the correct answer?
AI assistants like those described in Stevens’ talk would be a complete game-changer for science. However, these tools are absolutely useless if we cannot trust the information we receive from them. Thankfully, initiatives like FASST are working hard to solve AI trust problems and hopefully make AI assistants a reality.