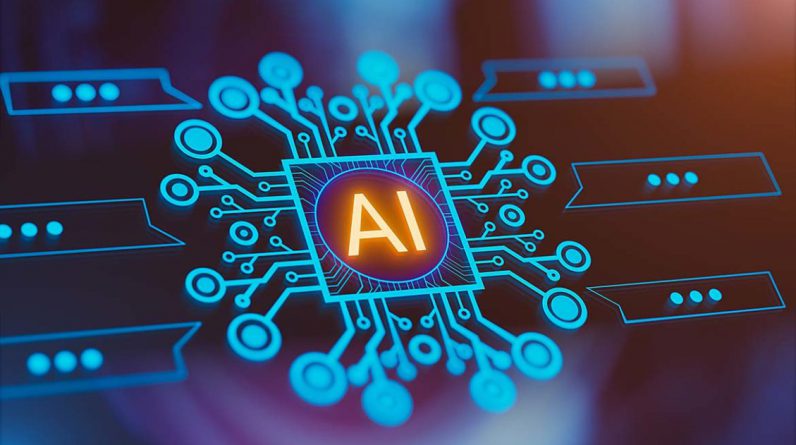
In the ever-evolving landscape of manufacturing and automation, the quest for efficiency, quality, and flexibility remains paramount. However, achieving these goals has become increasingly complex due to a myriad of challenges faced by modern manufacturing facilities. Fortunately, advancements in artificial intelligence (AI) and machine learning technologies offer a beacon of hope, promising to revolutionize industrial automation and address these challenges head-on.
Challenges Driving Interest in AI and Machine Learning
Manufacturers today grapple with the pressing need to predict manufacturing performance with unparalleled precision. Rising operating costs, including energy and software license expenses, coupled with the escalating costs of quality errors such as product recalls, underscore the urgency for solutions that optimize process efficiency. This imperative for efficiency gains drives the heightened interest in AI and machine learning technologies.
Generative AI and machine learning tools are particularly appealing as they offer insights into the underlying relationships within manufacturing processes. By demystifying these relationships, algorithms empower teams to unlock previously underutilized assets and enhance overall operational efficiency. Ultimately, the central question guiding manufacturing endeavors is: “How can we do more with less?”
Current Applications of AI in Industrial Automation
While AI adoption in manufacturing is still in its nascent stages, pioneering facilities have begun integrating AI into their operations. These early adopters, equipped with robust data infrastructure and a culture of continuous improvement, leverage AI for anomaly detection and predictive maintenance. By analyzing real-time data streams, AI algorithms can detect deviations from the ideal state and enact proactive measures to maintain process integrity.
Using data of stable processes to confidently address the limitations of a production line. This benefit can manifest itself in efficiency improvements, such as predictive maintenance rather than reactive repairs. Furthermore, it can increase quality by finding the relationships between raw material batches from specific upstream vendors and desired production metrics. As well as increase flexibility by empowering automation to both read and write data for production lot sizes of one. Where the verification of tasks that adhere to pre-planned work instructions can ensure that the entire data for the lot is complete before a product leaves a specific work cell. This flexibility can further manifest itself by challenging the sequential dependencies of the specific tasks, allowing each lot size of one to each be completed in the most efficient manner. Which maximizes output regardless of the mix of product to allow facilities to consistently meet production quotas.
However, widespread AI deployment in industrial automation faces hurdles, including the lack of standardized data aggregation frameworks and the absence of scalable deployment networks. Bridging these gaps is essential to unlock AI’s full potential in manufacturing.
Implementations of AI in Manufacturing Processes
When outlining the deployment of AI, regardless of the AI being generative and trained in an unsupervised manner or the AI being traditional and developed through data mining, it can be helpful to organize the machine learning system into three sections.
The first section is all about the data. A data first architecture enables the data to be aggregated holistically and with substantial granularity. Granularity preserves the context that the data was generated in. All without compromising the performance of the automation on the factory floor. The second section is the algorithm itself. Whether the algorithm is hosted on edge or in the cloud, this is the actual problem-solving operation. The third section is the neuro network that can deploy the mediation based on the prediction from the data aggregation and the algorithm in real time.
Of course, with the huge leaps forward we have seen in large language models in the consumer space, all the attention is on the second section. The algorithm is often the catalyst for an AI conversion regarding a potential machine learning pilot program.
Major challenges still reside in the first and third sections. Without an automation architecture which can aggregate data with a high degree of resolution and transport the data securely in the format which the algorithm requires, then a valuable algorithm cannot be built through data mining nor through reinforced learning. Without a neuro network to deploy a mediation or an avenue to collaborate with the tribal knowledge on the factory floor, then the process cannot benefit from the great leaps forward in algorithm development. Currently, we are seeing gaps in the first and third sections which need to be addressed before algorithm development can start.
Addressing Challenges and Ensuring Integration
When addressing these challenges, it begins with a mindset of unifying the automation on the factory floor. A good way to start down that path is to put data first. By looking at data holistically, teams can identify silos within their automation, then work towards a single connection and a single control unit. However, being data first does not mean being blind to the costs of short-sighted data aggregation. Technologies that are incompatible with the current automation architecture, require additional software licenses, compromise machine performance, or introduce additional cyber vulnerabilities should all be scrutinized.
To address these challenges and ensure successful integration of AI technologies into their automation systems, teams have looked to globally open industrial protocols. EtherNet/IP™, EtherCAT®, and IO Link can all be leveraged to start to reduce complexity on the factory floor while aligning with currently used protocols in native automation systems. When integrating or even updating automation to address these challenges, teams should start with a section of the plant floor at a time. Where upgrading a section of the plant floor at a time minimizes the risk to overall production by reducing the vulnerability of plant wide downtime through proper production planning. Starting small also creates an increased reservoir of spare parts for consumption elsewhere in the plant. This extends the transition period, allowing for more time to train maintenance and production teams.
The Future of AI-Driven Automation
Looking ahead, the future of AI-driven automation holds immense promise for manufacturers. AI technologies will continue to evolve, enabling algorithms to discern intricate relationships within manufacturing processes and optimize resource allocation. As AI algorithms become more specialized and adept at identifying analogies and patterns, manufacturers can expect unparalleled efficiency gains and competitive advantages.
In conclusion, AI and machine learning technologies represent a paradigm shift in industrial automation, offering manufacturers unprecedented opportunities to enhance efficiency, quality, and flexibility. By embracing AI-driven automation solutions and overcoming integration challenges, manufacturers can unlock the full potential of AI to propel their operations into the future.