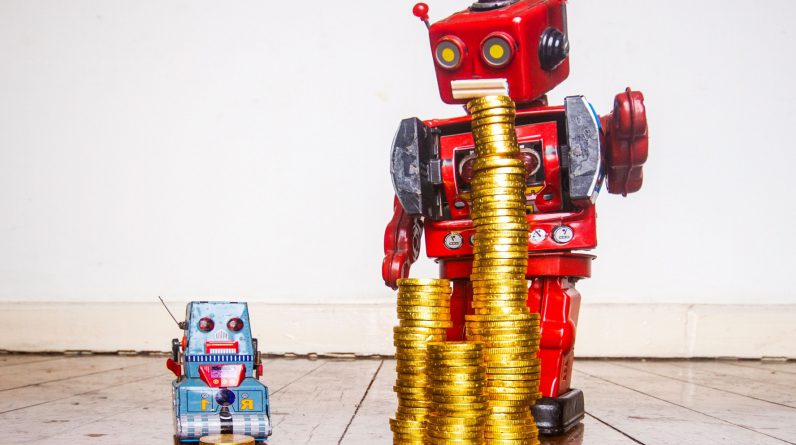
According to one survey, about half of Americans think that the increased use of AI will lead to greater income inequality and a more polarized society. Roughly two thirds think the government should take action to prevent the loss of jobs due to AI, and 46% of young Americans think that it is at least somewhat likely that AI will replace their job in the next five years.
While economists have generally been less concerned than the public about drastic scenarios of AI-driven job loss in the near-term, they often do share concerns around AI’s potential to exacerbate income inequality. To empirically estimate this possibility, researchers have recently taken to studying the impacts of new AI systems on worker productivity—a key determinant of wages. Interestingly, several studies have now found that within certain occupation groups—including lawyers, software engineers, customer service agents, management consultants, and workers performing professional writing tasks—the lowest skilled or least experienced workers derive much greater productivity gains from AI than their higher skilled, more experienced counterparts.
Some leading economists, such as MIT’s David Autor, have praised these results as supporting the hypothesis that AI could boost middle class wages and help reduce inequality. This would be a welcome course correction for technology’s impact on wages in the U.S. over the last 40 years, during which about 50-70% of the increase in wage inequality has been attributed to the introduction of new automation technologies. In an eloquent Noema magazine piece in February, Autor points to the task-level studies of AI’s impact on programming, writing-intensive work, and customer service work as evidence that AI can help enhance the capabilities of novice workers in these jobs, empowering them in a way that could help diminish inequality.
While this positive vision is a useful target for policymakers, this task-level evidence within occupations can be misleading when attempting to predict the economy-wide impacts of AI on inequality. By relying on it, we risk overlooking broader implications of AI on inequality both now—while AI is mainly used to boost worker productivity on tasks—and in the future, when new systems will become more reliable at fully automating more complex tasks. This commentary argues that there are at least two likely mechanisms through which AI could increase inequality in the U.S.:
- In the near-term, AI-driven productivity boosts could be skewed towards high-income workers, leaving lower-wage workers behind
- In the slightly longer term, AI-driven labor automation could increase the share of income going to capital at the expense of the labor share
High-income workers appear most likely to benefit from AI-driven productivity boosts in the near term
The first mechanism through which AI could increase inequality is by giving a stronger productivity boost to already highly-paid knowledge workers, while leaving many lower-skilled workers in in-person service and manual labor jobs behind. Indeed, most of the evidence mentioned earlier, which indicates larger productivity gains for lower-skilled workers within a specific job, comes from studies of workers who are already in relatively high-paying professions. The exception is customer service agents, whose income is around the 20th percentile in the U.S.
Recent research has zoomed out to assess the likelihood of AI-driven boosts to labor productivity across the entire economy, rather than within specific jobs. In a recently published study that I worked on with my co-authors Tyna Eloundou, Daniel Rock, and Pamela Mishkin, we found evidence to suggest that higher-income workers are in fact more likely to experience productivity boosts from AI. To illustrate this, the graph below shows expected exposure to the productivity gains from a GPT-4-like system (the y-axis shows the percent of worker tasks within an occupation where it could be technologically feasible to double worker productivity by using GPTs) plotted against annual wages for all occupations in the U.S. (x-axis). As you can see, exposure to productivity gains from AI are expected to be concentrated at the higher end of the income distribution, peaking at around $90,000 per year and remaining high for those who make six-figure salaries. Other research by Rob Seamans, Edward Felten, and Manav Raj found similar results when examining exposure to AI across all jobs in the economy, as opposed to focusing only on differences across workers within the same occupation.
It is important to note, however, that these studies are predictions based on secondary data. Rather than observing the impact of AI on worker productivity, they each estimate potential productivity impacts (or a broader general measure of exposure to AI) based on the level of overlap between descriptions of worker tasks and the capabilities of AI systems.
Despite this methodological limitation, if one considers how workers are accessing frontier AI systems today, this theoretical relationship between wages and productivity gains makes intuitive sense. Today’s most advanced large language models (LLMs) and multimodal models are primarily accessed in one of three ways: via chatbots, through application programming interfaces (APIs) that allow programmers to prompt models at a greater scale, or indirectly through software built on top of LLMs (for example, a legal research assistant or coding assistant). As such, the people best positioned to use and benefit from these systems are those who can easily interact with software as part of their existing workflows; namely, those who do much of their work at a computer. Those who work in agriculture, in the skilled trades, in-person service work, and in other professions composed mostly of physical labor have a much less direct access point within their existing workflows to the scaled productivity benefits these systems can provide.
Given this likely concentration of productivity gains among higher-income workers, policymakers should prioritize investments in AI literacy and access for a broader population of workers. In the short term, investing in education and training programs that help workers develop the skills needed to interact with and leverage AI systems can pay off by enabling a wider range of individuals to harness the productivity gains offered by these technologies. This could include training on how to use AI-powered software tools and fostering a more widespread understanding of AI capabilities and limitations. By equipping workers across the income spectrum with the knowledge and skills needed to work effectively with AI, policymakers can help ensure that the benefits of this technology are more evenly distributed.
Continued AI advancement could lead to automation that shifts economic returns from labor to capital
Differential productivity gains are not the only mechanism through which AI could lead to exacerbated income inequality. A near-term focus on observed productivity gains in task-level studies risks underestimating what seems to be a likely future: Continued AI improvement in terms of general capabilities, reliability, and the ability to do longer sequences of tasks independent of human instruction. If the pace of AI advancement on each of these dimensions holds, it seems plausible that leading AI systems could start to eat up a larger share of production in occupations where they are deployed. That means that where we see productivity gains now, there could be even larger growth in the future. In sectors where AI automation significantly reduces production costs, businesses may choose to reduce their workforce if consumer demand for their products or services doesn’t increase enough to offset the productivity gains. This could lead to job losses and lower wages in affected industries.
In such a scenario, firms adopting the most capable AI systems will need to make choices about how to allocate tasks and organize work across human labor and machines. In particular, as the task composition of work shifts towards working more with machines and overseeing their output, firms will need to decide whether to elevate their least experienced workers into positions that are more managerial in nature or to keep incumbent managers and experienced workers in place. This will be a multi-faceted decision, but it seems unlikely that the less-experienced workers within affected occupations would be the default beneficiaries of this transition.
Concretely, imagine a firm such as the one studied by Brynjolfsson, Li, and Raymond. In this study, a Fortune 500 enterprise software company that employs a variety of chat-based, technical customer support agents experimentally varied the integration of one of OpenAI’s GPT models into its internal customer support software. Across customer support agents, access to GPT-enhanced software increased productivity on average by 14%, though the gains were much larger for novice and less-skilled agents.
This is an exciting finding, but a key determinant of downstream impacts on wage inequality will be how the distribution of productivity benefits evolves as the technology improves over time. As these systems improve, customer service work could very well shift from fielding customer inquiries to supervising a small number of edge cases and areas where the AI systems can’t fully resolve an issue. This shift could reduce the absolute amount of human labor needed to produce the same output and shift economic returns towards capital if increased demand for customer service doesn’t outpace the productivity gains from AI. Furthermore, since advances in AI capabilities make equivalent human skills less scarce, the wage premium for those skills should be expected to decrease as AI improves. By default, this won’t necessarily provide boosts to lower-skilled workers or high-skilled workers but could exacerbate the shift from labor towards capital.
We may already be seeing this dynamic unfold. Recently, Klarna, a Swedish fintech company, reported that their AI system was performing the work of 700 customer service agents about a year after they laid off 700 of their employees. In situations like this, it seems probable that low-skilled agents won’t be the most likely candidates to be elevated into the remaining supervisory and AI-complementing roles; they could be the ones most at-risk of being automated out of a job. If that holds true at a larger scale, then we could put ourselves back into a position of hollowing out middle-wage jobs.
As the technology matures, the relatively larger productivity gains we’re currently observing for lower-skilled workers in occupations exposed to AI would, in this scenario, end up transitioning into greater risk of job displacement for those same workers. Here, policymakers again have an important role to play. Investments in training and transition assistance can be helpful, while strengthening safety nets for displaced workers with other vulnerabilities can provide support to workers who face a greater risk of harm from AI-driven job loss.
This time might actually be different
Finally, let’s zoom out once again, this time to consider the oft-repeated claim that there will always be new jobs. This has been true since the Industrial Revolution, but whether or not it continues to be true has major implications for inequality in the future.
Perhaps AI leaders prophesying about superintelligent systems are just engaging in hype. However, the world’s leading machine learning experts are increasingly convinced that human-level systems are on the horizon, and investors are putting billions of dollars on the line betting on significant advances. OpenAI—a leading AI research and development company—has the stated mission of developing systems that “outperform humans at most economically valuable work.” OpenAI’s CEO, Sam Altman, has predicted that the demand for many kinds of labor will fall towards zero once sufficiently powerful AI is deployed into the economy. A 2023 survey of thousands of leading AI researchers from academia and industry put the probability of all human occupations being fully automatable at 10% by 2037 and over 50% within the next century.
They may or may not be right, but in case they are, policymakers would be wise to recognize that technology-driven job automation may not always be accompanied by plentiful job creation. If leading AI labs achieve the goals they publicly aspire to, the economic impacts of these systems will have a fundamentally different set of societal ramifications relative to past technologies. These ramifications stem from a technology that, for the first time in history, is being developed with the intent to not only perform current human tasks but also to execute all possible cognitive work—including newly invented tasks—more effectively than humans can and at a lower cost. Success in this technological endeavor surely isn’t guaranteed, but the possibility of success poses many challenges to policymakers. How will society be organized, income distributed, human agency preserved, and prosperity equitably shared in a world where machines outperform us in every dimension, even those that we haven’t yet conceived of? Addressing these challenges will be crucial to ensuring that the transformative potential of AI benefits all members of society and does not harmfully exacerbate inequalities.