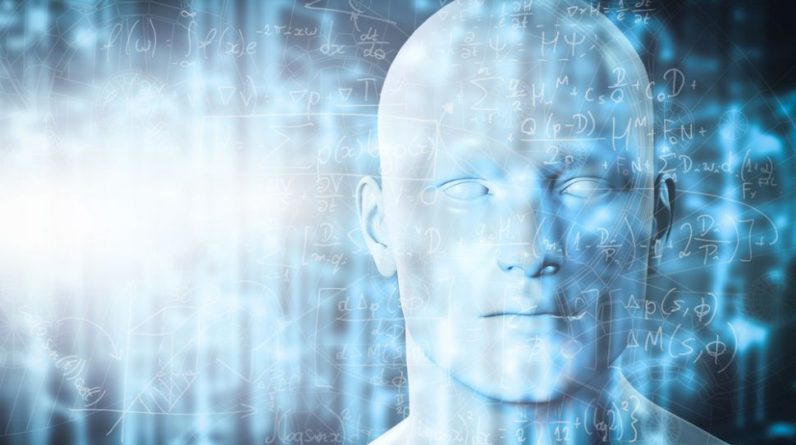
Companies are rushing to address IT and business challenges by implementing AI strategies. But their roadmaps are leading in dozens of directions amid a barrage of AI hype and hope. We’re fast realizing that putting AI to work, including machine learning and generative AI, in an effective and cost-efficient way requires accurately diagnosing deep-seated enterprise problems.
While the depth of problems can vary from organization to organization, enterprises experience considerable friction due to cloud migration complications and constantly evolving core technologies, platforms, and ITSM tools. Diving deeper, cross-enterprise challenges also include a broad lack of maturity in automation adoption, digital labor, systems-driven operational models, and AI-enabled tools themselves. All of this is often wrapped up in failures to understand core process issues that have stalled digital transformation and acceleration initiatives.
Enterprises can become frustrated with little measurable success, despite major investments of time and money. The future of IT service management depends on the accurate examination of this impasse — and fine-tuning AI, guided by human oversight and evaluation, to navigate processes better and achieve higher quality results faster than we currently do.
Deepesh YK
Social Links Navigation
Head of Intelligent-Infra Services, SLK Software.
Challenges multiply when enterprises fail to understand process issues
When organizations fail to fully identify and understand issues with their business and technical processes, they also waste time and resources trying to identify and implement the right solutions for the organization, at the right time. While executives and management often have a solid awareness of the impact of technology adoption and process problems on the business, they typically lack visibility into the root causes of, say, skyrocketing cloud spend, poor data management, or inefficient workflows and tooling.
A lack of process and procedural “hygiene” is responsible for much of the inefficiency and noise. That bad hygiene can manifest as a lack of discipline in organizations to log tickets for every issue handled, to capture proper issue-related details in the tickets, and to capture proper work notes and resolution notes. These seemingly small details can wreak havoc on the health of enterprise systems and larger business objectives. This is a manifestation of the “watermelon effect” at work: everything may look green on the outside, but the issues surface in red when you cut deep. Intentionally or unintentionally, a healthy assessment might be conveyed to higher tiers of management and to the public, while rot is taking hold deep in the system.
What’s more, many organizations focus on operational-level KPIs rather than business-level KPIs, which means the needle may not be moving toward better business performance. Organizations can also suffer due to inadequate technical expertise, domain expertise, and staffing relative to capacity. Undocumented and “tribal” knowledge locked within incumbents inside the organization contributes to siloed IT approaches and solutions that detract from achieving business objectives. Tribal knowledge may also, at times, be locked in archaic approaches and technologies which further hinder modernization.
Other factors that can drag enterprises down include inefficiencies in hybrid or work-from-home models, the ongoing tightening of compliance and regulatory standards, and a lack of unity and clarity across the organization in long-term growth and modernization strategy.
AI: Extracting intelligence and solving problems for optimized services
Generative AI, machine learning, natural language processing (NLP) and other forms of AI can be of great help in revealing, simplifying, and solving these pain points. AI can aid in extracting intelligence that can be used to eliminate challenges with process issues, technology adoption, meeting business-level KPIs, and increasing team productivity.
Specifically, with ITSM, generative AI can drive positive results by addressing historic contexts as well as issues in real time. It can help humans identify trends and patterns, formulate insights, determine better processual steps, and even generate summaries of incidents and events with respect to both infrastructure needs and customer needs. AI can quickly summarize triage across the incidents and generate overall resolutions implemented by directly fetching inputs from mail chains if needed. Humans can then review those AI-generated summaries and take corrective action that saves the organization time and money. These comprehensive insights across tickets and systems issues can provide clarity on the nature, impact, and volume of challenges the organization faces. That data helps in prioritizing automation and resolution efforts.
Importantly, AI-summarized triage notes and resolution notes across tech teams and tech stacks offer a more holistic view of events flow across teams, which helps provide an end-to-end picture of the ITSM environment and can reveal any process inefficiencies. Also useful is AI’s ability to generate concise management reports, while enabling more thorough and comprehensive documentation for future reference.
Better configuration, proactive prediction, and a reduced skills gap
Additionally, predictive AI, platform AI, NLP, and AI in Site Reliability Engineering (SRE) can be used to assist in a wide variety of ITSM work to define the processes and methodology of operations. For example, by consuming metrics, logs, and traces, AI can enhance its predictive capabilities, helping an organization prevent incidents before they occur. With data unique to an organization, AI can be trained to differentiate between positives, false positives, and negatives. It can help in event correlation among historic incidents and unearth patterns, volumes, and impact metrics — which will later be matched up with real-time metrics to proactively predict incidents.
AI-driven infrastructure-as-code (IaC) and configuration-as-code (CasC) can help in streamlining and standardizing infrastructure provisioning and maintenance, reducing the burden on humans. AI can also help predict the impact of configuration changes and automate configuration optimization, which helps reduce management efforts. And AI tools can aggregate the best reference material and provide it to the right teams — reducing the skill gaps where that’s most needed.
Not least, AI can help to team up individuals working on the same issue across different organizational silos. It can unearth process-related issues in those silos and across the teams. It can “artificially” merge siloed services across teams for a more comprehensive view of the overall health of IT services and systems, while helping reduce costs by eliminating idle resources, duplicated work, and over-provisioning.
Looking forward
In ITSM, AI will have a profound, long-term impact. It will help to bridge talent gaps in the global workforce, and in the next five to ten years, we will see increasing use of digital labor and a meaningful shift in how human labor is levied.
As AI matures, it will become capable of solving increasingly complex problems, and even more comprehensively and independently identify root causes of technical and process issues across enterprises. Predictive analytics will facilitate proactive and preemptive systems maintenance. Industry-specific AI engines, trained for particular domains, will increasingly have the ability to quickly or near instantaneously learn and adapt to the needs of the organizations where they are deployed, minimizing the maturity curve. All of this will reduce systems downtime, while improving stability and security across the enterprise IT landscape.
The next generation of IT professionals will also be affected, and in order to bolster their careers, they must prepare for a period of transition. Developing an understanding of the IT landscape, as well as how to use, confirm, measure, and enhance the effectiveness of AI engines within organizations will become critical. A key part of their jobs will involve training AI engines and accounting for latest enhancements, as they strategize AI-to-business outcomes.
Right now, business leaders seeking to understand the intersection of AI and IT service management must define the most effective strategies and timelines around why and how to implement AI. Having the right AI solutions, knowing how AI engines can be effectively adopted across an organization for best ROI, and securing the right talent and partners to build and manage AI are collectively an uphill task. It’s worth the effort in the long term, due to cost efficiencies and performance improvements that AI can deliver at scale.
We’ve featured the best AI phone.
This article was produced as part of TechRadarPro’s Expert Insights channel where we feature the best and brightest minds in the technology industry today. The views expressed here are those of the author and are not necessarily those of TechRadarPro or Future plc. If you are interested in contributing find out more here: