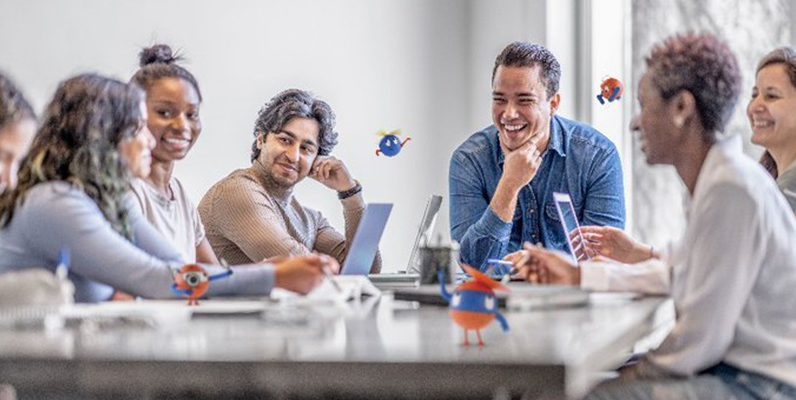
UiPath used its UiPath Forward + TechEd 2024 conference this week to explain where the enterprise automation and AI company that we all know for its Robotic Process Automation (RPA) goes next… and, that place is a ‘planet’ filled with agentic AI workflow agentification.
Founder and CEO of UiPath Daniel Dines kicked off proceedings with his usual ‘upbeat but essentially deep-geek’ approach, a pleasing delivery that is further amplified by the Romanian slant in his accent.
In a keynote entitled “The Future of Business: Robotic and Agentic”, Dines explained how UiPath has always been on a mission to emulate humans at a more intellectual level through automation.
“As we enter a new era of agentic AI, we will have the tools to deliver the autonomous enterprise – an organization that effectively combines RPA with new agentic capabilities. This combination forms dynamic, adaptive workflows that excel with minimal human oversight, heralding a new chapter in business process automation,” noted Dines.
The mission right now – inside UiPath – is to explore how agentic automation is capable of building on traditional RPA to now incorporate a new degree of deep learning alongside new foundational models to autonomously discover, perform and manage the long tail of complex tasks.
But – for all the corporate positivity – we’re at a perplexing point with AI in many senses. UiPath’s Mary Tetlow chief brand officer reminded attendees that market workplace analysis reports suggest that as much as 95%+ of modern jobs have the potential for AI to automate parts (if not all of them), but the rollout of so much of these new tools has still yet to reach its full potential in some areas and industries.
Relax, bots do it
Dines took the stage (walking on to Relax, by Frankie Goes To Hollywood) to tell us that now marks the beginning of UiPath’s second act as a company. Act 1 for UiPath was the company building a technology that was capable of imitating people and that technology was capable of working with structured information and rule-based business processes that were based upon trusted precision models.
But from the UiPath document processing arm evolved an organisation with technology capabilities that would also be able to work with semi-structured data as it became more and more advanced.
We do need to remember that [good, reliable] automation is hard according to Dines i.e. to be able to run thousands of automations in a dependable way across a business is tough, but it is what UiPath has been refining over the last decade. He notes that the company has spent considerable time creating discovery tools that would further automate the use of these technologies and reduce the need for human input in these processes.
Primary AI convergence mechanism
“Agentic automation will rapidly become the primary mechanism to converge AI with rules-based technologies to automate and augment knowledge work,” said Maureen Fleming at IDC. “The combination of generative AI and AI agents represent the first-time knowledge workers will meaningfully gain the benefits of business automation to help them do their jobs, creating the next level of value from automation across enterprises.”
But any use of generative AI can be unpredictable (it is a non-deterministic technology after all) so we wil only want to use it to a certain defined point.
There are questions about how far we give AI agents passwords and credit card details – so we (for now at least) will still keep humans in the loop at this point. As the AI agent starts to learn that one user always opts for a particular brand of purchase for any given product or service, we may soon get to a point where we start to bypass human validation at a certain level (or at a certain monetary value) and this confidence will grow and develop over time.
Now that we are working with generative AI, we stand at a point where we can start to apply automations upon the more unstructured parts of the business operations layer. UiPath CEO says that AI agents are going to become prime citizens, AI agents have an instruction set (in natural language), a memory (which it will build experience with) and a set of prompts and a memory to be able to operations.
Agentified workflows
An AI agent needs to break down complex workflows into their component parts so that we can use agents + LLMs + robots in a unified and orchestrated way as businesses now work to construct what we can now agentified workflows.
As those workflows are now broken down (take a credit risk assessment created by a bank) there are some deep calculations that AI agents will be well suited to handle, but parts of that total workflow may still need to be handled by human beings. But as we know, no business will be able to apply any level of automation to a bad workflow process and expect it to work better, so there is a business element of this whole process that needs to be analysed first.
The future for not only AI agents but augmented AI agents, success in this space comes down to being an ecosystem player i.e. let’s be honest, no business jumps from no AI agents to a mass deployment of agentified workflows overnight, so firms will need to look for strategic first application deployment areas where they can validate proof of concept ideas and then extend and scale going forwards.
As so many specialists in this space remind us, automation is not necessarily about cost savings, it’s about agility and efficiency in the first instance.
A working AI agent example
Looking at a working example, let’s consider a travel agency AI agent.
This AI agent has a prompt that employees can use to find flights and hotels. It will find recommendations (and make sure that they match company policy which it has been given access to) and deliver those recommendations to employees who can go ahead and book flights for the customers who request them.
The agent has another set of tools to be able to search specific airlines and another to aggregate results to be able to find the best deals. We can ask this AI agent if we have a specific trip coming up – in natural language which the AI agent will extract the appropriate intent from – what we need, using Large Language Models to augment the automation with a degree of basic human knowledge that helps the whole automation work in a fluid way.
The feeling from both Dines and his guest speakers at this show is that bots are no longer restricted to working inside the structured part of an organization’s business process.
If the coming year 2025 will teach us anything, it is the what, when, where, why and how of actually applying automations with the injection of LLM power from non-deterministic generative AI functions into more complex business processes.