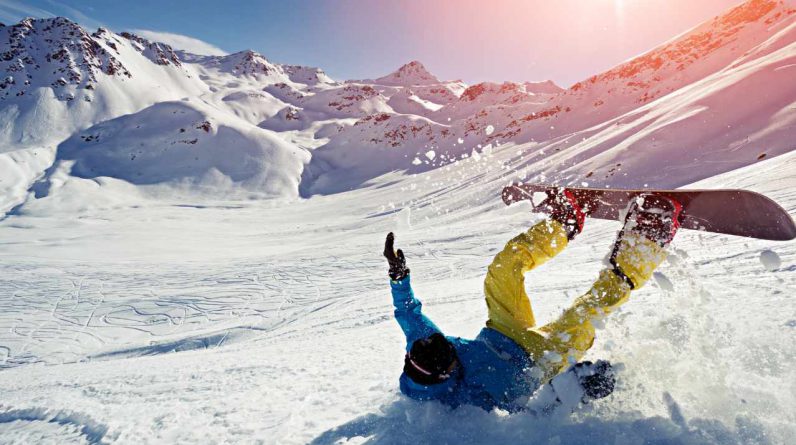
Lead story – How to survive the looming AI avalanche of enterprise automation
Phil’s converging threads of team collaboration and agentic automation are worth a close look. I found his opening statement interesting: “AI is making it dramatically easier for people to automate the work they do.” At first read, I’m not sure I agree with that, so what is Phil’s position?
Whereas in the past, building an automation meant mapping out every possible instruction and carefully linking each of them to potential actions, generative AI cuts out a lot of that manual effort. It is able to understand everyday language and automatically figure out how to work with data and functions it finds in the underlying system to deliver what the user is asking for.
Makes sense – though I’d file some of this in the co-pilot/assistant bucket, rather than building true automated processes. I believe that deeper enterprise automations require a level of governance – and auditable reliability – generative can’t provide on its own (but can usefully trigger). Phil continues:
This means that developers can build automations far more quickly than before, and in many cases non-developers can use no-code tooling to build automations themselves without even having to involve developers. It sounds like a huge step forward, but is a rapid roll-out of new automation necessarily going to be a good thing?
I hear mixed reviews from developers on how much generative AI helps them, but I do think ultimately most developers will find a use for these tools. But is it a huge step forward for enterprise-grade code, where gen AI code output tends to require quality/security review? I suspect on some projects, it will be. In other cases, the necessary scale for that kind of impact might not be there. On the low-code/no-code automation side, I like the concept of business users creating workflows with gen AI, and handing them to IT for discussion/implementation, but I wonder how fully automated that will be anytime soon. Phil raises a different caution here:
These are automations that always were needed, and could have been delivered earlier if they’d been prioritized, but were denied the resources, budget or motivation. AI simply lowered the bar to getting them done. The ROI comes not because of AI per se, but as a result of the automation of previously manual processes. Nevertheless, AI swoops in at the last moment and gets all the credit.
Phil also cautions against automating sub-optimal processes, without taking the chance to rethink them. You think tech debt is a problem? Add ‘process debt’ to that list:
With everyone suddenly able to automate their own processes, there will soon be multiple automations across the enterprise, each doing essentially the same thing but in very different ways — the vast majority of which will be hugely inefficient. Developers often talk about the concept of technical debt, where successive enhancements and modifications are layered on top of each other and over time lead to inefficiencies and potential conflicts. The same pattern exists in process debt.
If can take this forward, orchestration will be a must. Perhaps an “automation library”, where users can invoke approved transactional automations as they see fit, via their own co-pilots.
Example: users generate their own emails or code, to a varying degree based on their skills, client know-how etc. I see most of these as partially automated, but they can still add value, e.g. including a ‘set up a demo call’ button in an email triggers a series of agentic bot interactions to schedule the demo, and automate any contractual follow up steps (e.g. Docusign on steroids).
I don’t think agentic AI can make up for companies that haven’t already done some heavy lifting around metadata, systems integration, process automation and even RPA-type workflows. Agenetic AI can leverage all of those things and make them more accessible to users, but it can’t suddenly replace them, or compensate for their absence.
To some extent this is all uncharted territory, in a good way. You can expect plenty of diginomica use cases on these topics in 2025.
diginomica picks – my top stories on diginomica this week
Vendor analysis, diginomica style – earning reports of note:
Event season finally winds down:
- Hallelujah! The importance of being practical – generative AI pragmatism from Amazon CEO Andy Jassy – the last major enterprise show, re:Invent, is in the books. One highlight? Jassy on Amazon’s internal gen AI use across 1,000 apps. Stuart quotes Jassy’s advice, some of the more candid I’ve seen from the mega-vendors: “You need a good model, but it’s not just the model. In addition to the model, you have to have the right guardrails, and you have to have the right fluency of the messaging, and you have to have the right UI, and you have to have the right latency or it’s a really slow, laggy experience, and you have to have the right cost structure. I think a lot of times, what happens is you build these apps, you use a great model, you do a little bit of work, and you think, ‘I have a great generative AI app’, and it turns out that you’re really only about 70% of the way there. And the reality is customers don’t take kindly to apps that have 30% wonkiness.”
- First Rail pursues ‘clean core’ SAP S/4HANA implementation – Derek was on the ground for UKISUG Connect, and filed this instructive S/4HANA migration story: “Fortune explained that his team worked with finance users across ‘fit to standard’ workshops, so that the organization could be honest with itself about whether or not it needed uniqueness across its processes.” Also see: highlights from my pre-event discussion with new UKISUG Chair Conor Riordan: UKISUG Connect – discussing the hot topics of RISE, ERP migrations and AI with new UKISUG Chair Conor Riordan.
- ASUG Tech Connect brings clarity to LLM accuracy – and shows how SAP’s GenAI Hub can bring AI to all customers – This user event brought the LLM research/use cases I’ve pursued this fall to a head: “ASUG Tech Connect provoked a different AI conversation than expected. The show demonstrated how SAP customers can get access to SAP AI services now, via BTP and the GenAI Hub. Here’s what I learned on LLM accuracy from SAP’s Walter Sun, and SAP partner sovanta AG.”
A few more vendor picks, without the quotables:
Jon’s grab bag – Cath surfaced a nifty tech for good story in Using technology to support neurodivergent passengers during train travel. Sarah examined a destination web site conundrum in As organic search declines, personalize to convert the visitors you still get, says Webflow CEO. Yes, better conversion rates are always good, but you can’t stop there. Brands that haven’t figured out how to cultivate opt-in communities are in for a web analytics cold shower…
Barb managed to knock a chip off my shoulder again (well done), this time with Can AI really drive the success of email marketing? I’m still waiting to get an effective, ‘AI personalized’ email in my inbox. Brands still seem fine about risking the collateral damage of digital spray-and-pray, as long as a certain percentage engage. If AI can free our inboxes from that, I’ll personally sign up to serve our robot overlords. Speaking of, George turned his own experience with algorithmic-false-positives to good use in Why hallucinating parking AI needs stronger governance. (Musing here: given the difficulty of getting any kind of algorithmic screw up overturned, I wonder if agentic gen AI could lend us all a hand here, fighting it out with the bot that grieved us).
Best of the enterprise web
My top seven
- AWS CEO Garman Q&A: Model choices, competition and AI’s future – Constellation’s Larry Dignan rounds up the re:Invent news, along with some Q/A highlights. One story to watch: how successful will customers be with “build your own” AI models?
- UnitedHealthcare spotlight reveals pivotal AI failure – This is not a happy story, but are there lessons here on putting bad/unethical algorithms into production?
- Who’s the Bigger Villain? Data Debt vs. Technical Debt – A provocative assertion: “Although data debt and tech debt are closely connected, there is a key distinction between them: you can declare bankruptcy on tech debt and start over, but doing the same with data debt is rarely an option.” I’m not sure that’s true – try telling airlines they can get rid of archaic crew scheduling systems, etc., but it makes for an interesting premise.
- AI-driven software testing gains more champions but worries persist – Joe McKendrick quotes a recent study: “The debate on which quality engineering and testing activities will benefit most from Gen AI remains unresolved.” If gen AI can change software testing, McKendrick writes, organizational resistance will need to be overcome.
- What McKinsey learned while creating its generative AI platform – We need more of these internal project stories: “Yes, you need a data architecture. You need a data framework for thinking about how to tag and label things. You need a bit of a curation process.”
- It’s Time to Kill the Term ‘Citizen Developer –
- #shifthappens Podcast – Moving Beyond AI Hype to Responsible Implementation –
Whiffs
This went well:
Booking.com took this privacy glitch in stride:
Good to keep in mind – we are always the product, and “free” and “open” are music to the ears of AI scrapers and trolls:
If you find an #ensw piece that qualifies for hits and misses – in a good or bad way – let me know in the comments as Clive (almost) always does. Most Enterprise hits and misses articles are selected from my curated @jonerpnewsfeed.