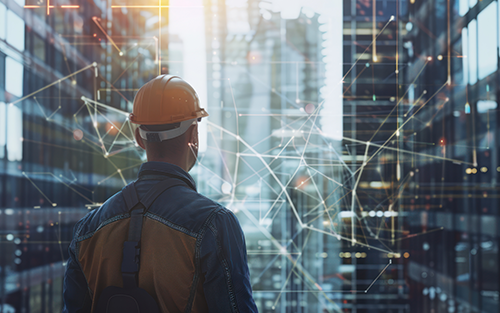
Summary
The most competitive facilities will have fine-tuned their relationships with AI to boost data integrity.
How to Improve AI Quality Control in Manufacturing
Artificial intelligence (AI) is a powerful resource for manufacturing companies, but it could be more reliable. The most competitive facilities will have fine-tuned their relationships with AI to boost data integrity. What techniques and tools should automation and industry workers use to improve AI quality control?
Data quality and quantity improvement
If robots have computer vision to identify defects on the production line, they must have clear pictures of every problem. Sparse or incomplete datasets compromise quality control, primarily when relaying information to employees. The data must also contain more than descriptions of visual concerns. It should contextualize them by explaining material, environmental and equipment factors.
Data enrichment is the best way to battle this common hurdle. It’s achievable by assigning more categories, expanding metadata and entering more into the sample size.
Integration of multimodal sensing technologies
A drone, cobot or Internet of Things (IoT) device should be equally capable of using multiple senses. It should detect changes in temperature, visuals and sound. These abilities are critical when numerous goods are altered by environmental conditions or signal quality issues when their characteristics change.
Assessing quality from multiple vectors boosts the chances of accuracy. In one study, this strategy effectively balanced the macroscopic and microstructural aspects of food samples. The AI could notice these factors in a nondestructive way, maintaining food preservation throughout processing. The analyzed samples could still go to customers without generating waste.
Continuous learning and adaptation
Manufacturers must know AI training is a never-ending job. There is no endpoint when the dataset is full enough. The workforce must constantly feed it so it can use its machine and deep learning algorithms to learn more. Enhancing an AI’s ability to see quality control issues relies on the staff’s willingness to learn and translate what they observe into the AI continually.
Continuous learning also refers to the manufacturer’s ability to cleanse and govern the dataset. Regular audits will reveal redundancies, variances and integrity problems that could skew results. Data scientists may use feature engineering and model accuracy to explain questionable decision-making. Without this accountability, the AI will not adapt to the organization’s ever-changing needs.
It creates a positive feedback loop, encouraging workers to pursue knowledge and correct oversights in the AI. Continuous learning and adaptation become fulfilling if the information provided and gathered optimizes the manufacturing process, which influences the supply chain, procurement and production.
For example, employees can refine an AI’s ability to execute forecasting by analyzing product trends and demand. Eventually, the AI will categorize items based on their likelihood of causing a bottleneck or suffering demand fluctuations during manufacturing.
Advanced anomaly detection techniques
Cameras and sensors may notice a discoloration or packaging dent with cameras. These tools will reveal only some problems a faulty production line could generate. Eventually, AI memory will expand further to see more anomalies than manufacturers typically do.
Consider how an AI could review the material composition of a toy’s plastic packaging to determine its safety for children. It could also find the smallest instabilities in an electronic device, like a printed circuit board, and run simulations to determine whether it will sustain damage during transit.
These are concerns technicians may not be aware of upon a quick glance. AI could reduce harder-to-find anomalies to save money and protect customers.
User-friendly interfaces and collaboration tools
Many quality control errors with AI come from the model itself. However, some come from employees. Making user interfaces (UI) as easy to control as possible is critical for consistent training and accessible on-the-floor operations. Personnel must understand what data visualizations represent and how to report quality metrics to superiors or compliance auditors.
Manufacturers can help by expanding educational opportunities for staff. There should be introductory training with subsequent seminars to deepen the employees’ knowledge of the AI tools. This approach will help with collaborative environments, where many people enter information or comment on AI behavior in a centralized digital space.
Manufacturers can also implement plug-and-play AI models that run autonomously and have a short time to value because they require minimal training.
Boosting AI-driven quality control
Manufacturers need better AI to shorten lead times, increase productivity and promise higher quality control. These strategies address the most common oversights in industrial AI, so stakeholders must invest equally in their development, research and implementation. Doing so will ensure expertly crafted products reach customers safely and swiftly.
About The Author
Zac Amos is the features editor at ReHack, where he covers trending tech news in cybersecurity and artificial intelligence. For more of his work, follow him on Twitter or LinkedIn.
Did you enjoy this great article?
Check out our free e-newsletters to read more great articles..
Subscribe