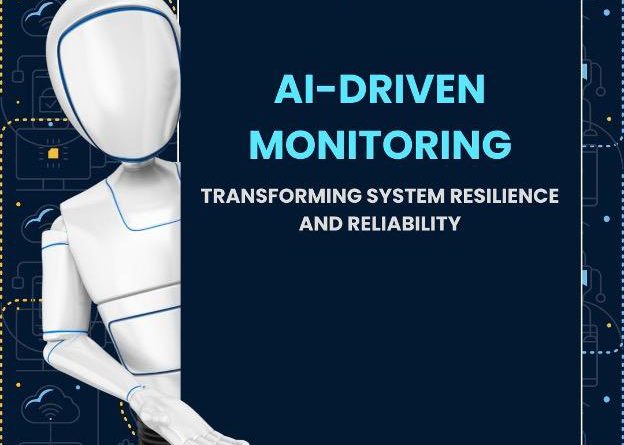
In a world increasingly reliant on real-time operations, the adoption of AI-driven monitoring has emerged as a significant breakthrough. Anil Kumar Thimmapuram’s research highlights innovations in advanced system monitoring solutions, focusing on enhancing resilience and reducing recovery times. By merging AI analytics with human expertise, his work underscores the transformative potential of intelligent automation in operational management.
Redefining System Oversight through Intelligent Monitoring
The reliance on middleware systems for seamless operations has historically been plagued by delays in detecting and resolving failures. Traditional monitoring mechanisms often react to incidents rather than predicting them. AI-driven monitoring, however, marks a departure from conventional approaches. By leveraging AI tools capable of processing vast operational data, the system identifies anomalies, triggers proactive interventions, and minimizes disruptions. This shift from reactive to predictive oversight ensures better resilience, which is critical in industries with interconnected services.
Optimizing Fault Detection and Recovery
One of the core strengths of intelligent monitoring systems lies in their ability to optimize fault detection and recovery. Automated tools pinpoint failures and predict potential disruptions before they escalate. Self-healing architectures further accelerate recovery times, achieving a 25% reduction in Mean Time to Resolution (MTTR). These enhancements demonstrate how AI bridges gaps in existing processes, ensuring faster and more reliable system recovery.
Human-AI Collaboration for Superior Outcomes
Despite the advancements in automation, AI-driven monitoring does not replace human expertise. Instead, it complements human capabilities, creating a synergy that enhances decision-making. The combination of human insight and AI’s computational efficiency significantly improves anomaly detection accuracy. The research illustrates a 93% success rate in collaborative fault resolution scenarios—far surpassing outcomes from isolated human or AI-only efforts. This collaboration not only accelerates incident responses but also fosters trust in automation among operational teams.
Reducing Noise and Enhancing Accuracy
Alert fatigue has long been a challenge for operations personnel, particularly in complex environments with multiple interdependent systems. AI-driven monitoring addresses this issue by reducing false positives and refining anomaly detection. With a reported false-positive rate of just 3%, the system ensures that alerts are both actionable and accurate. Advanced algorithms analyze contextual patterns, recognizing subtle deviations that traditional systems often overlook. By filtering noise and presenting prioritized issues, these solutions allow human operators to focus on critical incidents.
Self-Healing Architectures for Uninterrupted Operations
AI-driven systems enhance system resilience by integrating self-healing mechanisms and optimizing resource allocation. Leveraging container-based deployments and service mesh integrations, these systems monitor real-time performance, autonomously resolve failures, and dynamically allocate resources to minimize downtime. By analyzing usage patterns and predicting workloads, AI ensures efficient resource distribution, reducing manual interventions by 40% and operational costs by 28%. These advancements establish a sustainable model for uninterrupted operations, balancing system demands with optimal performance and reliability.
Benchmarking Resilience and Scalability
Performance benchmarks such as Mean Time to Resolution (MTTR), anomaly detection accuracy, and automated recovery rates highlight the effectiveness of AI-driven monitoring systems. Achieving 89% accuracy in identifying anomalies and improving recovery rates by 37%, these solutions set new standards for operational resilience. Their modular architecture ensures adaptability and scalability, enabling incremental expansion without performance degradation. By balancing scalability with stability, intelligent monitoring systems redefine reliability and remain future-proof amidst growing operational demands and evolving technological challenges.
In conclusion, Anil Kumar Thimmapuram’s research demonstrates how AI-driven monitoring systems revolutionize fault detection, recovery, and operational efficiency. By integrating advanced analytics with human expertise, these solutions offer unprecedented improvements in system resilience. The research serves as a testament to the transformative impact of AI, establishing a benchmark for intelligent, automated oversight in complex operational environments. As industries continue to embrace AI, innovations such as these pave the way for smarter, more reliable systems.