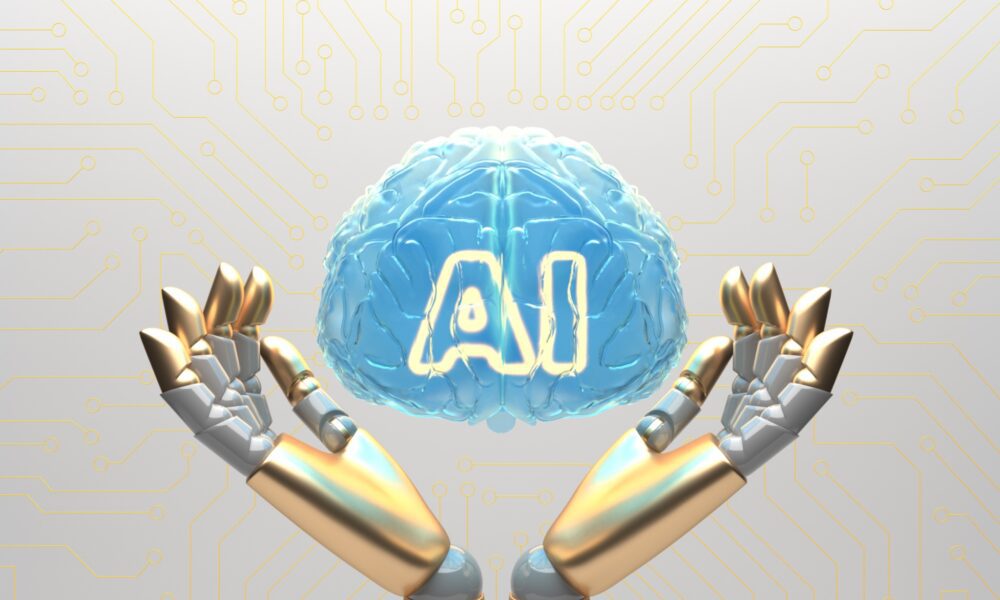
From opening your phone with face ID to scrolling your social media feeds, inquiring about the weather, commuting to work, or watching Netflix, today, there are a wide variety of examples of how we use artificial intelligence (AI) in our everyday lives.
Although cutting-edge technology is the talk of the town today, it has been around for over 70 years. AI has evolved with time, and today, almost every industry is leveraging its benefits to provide customized solutions, enhance customer experience, make smarter decisions, and a lot more.
In fact, 82% of business leaders believe that AI enhances job satisfaction and performance. Moreover, 94% of business leaders believe AI is critical to success over the next five years.
As you start looking into how to use AI, it’s important to know that not all AI applications are the same.
As artificial intelligence ushers in new technology programs and ethical concerns, various concepts and vocabulary have come about to understand it.
To get a full grasp on how AI operates and for what purpose, one should understand the difference between conversational AI and generative AI. While these two branches of AI work hand in hand, each has distinct functions and abilities.
Here, we’ll discuss the differences between conversational and generative AI, as well as how they work together. Let’s get started!
Understanding Traditional Artificial Intelligence (AI)
A Brief Overview
Traditional AI, often called narrow or weak AI, refers to the technology that helps machines engage with humans in a more natural way. It’s best used for tasks related to customer service or virtual assistants.
These systems use predefined algorithms and rules derived from structured data to perform preset tasks. These applications are specifically designed to excel in a single activity or a limited set of functions, whether it’s playing computer chess, diagnosing diseases, or translating languages.
Think of customer service chatbots or virtual assistants like Siri, messaging apps, and Alexa. These technologies are known for their conversational abilities, assisting people through back-and-forth communication.
Traditional AI Applications
Traditional AI is like the brain behind helpful tools we use every day. Think of customer service chatbots and virtual assistants like Siri – they’re good at talking with us and helping out. Also, when you get recommendations on Spotify or Netflix, it’s thanks to traditional AI. It looks at what you like and suggests more things you might enjoy.
Google’s search bar is another example. When you type something, it uses traditional AI to find the best results for you. AI in healthcare assists physicians by analyzing patient data and making recommendations for potential diseases or therapies. In terms of finance, it safeguards your funds by identifying anomalous transactions that could be fraudulent.
Traditional AI is like a super inspector in companies, ensuring products are top-notch by checking for any problems during manufacturing. So, whether it’s making our music playlists better or keeping our money secure, traditional AI is all around us, making things work smoother and smarter.
Limitations and Challenges of Traditional AI
With so many pros of traditional AI, it also faces several limitations and challenges. One major issue is the reliance on predefined rules, making it less adaptive to new, unforeseen scenarios.
Additionally, traditional AI often struggles with understanding context and lacks the ability to learn continuously. The scalability of these systems is another challenge, as they may not effectively handle large datasets or complex problems.
Overcoming these limitations is essential for advancing AI technology and addressing real-world complexities.
Understanding Generative Artificial Intelligence (AI)
Generative AI, on the other hand, enables users to create new content, whether it be text, images, animations, or sounds. It is the next generation of AI that relies on machine learning algorithms and the data they’re trained on and comes in handy for writing and other content creation tasks.
Some famous examples of generative AI include Bing, Google Bard, ChatGPT, and Copy.AI.
Meanwhile, generative AI uses neural networks to identify patterns in its training data. By identifying these patterns and taking note of human responses and feedback, generative AI programs learn to create more accurate content.
Here are some significant differences between the two:
Learning Approach
Traditional AI: Relies on rule-based approaches, following explicit instructions and predefined rules set by human experts. It operates within the confines of programmed directives, lacking the ability to adapt beyond predefined boundaries.
Generative AI: Embraces a data-driven approach, learning patterns and structures from extensive datasets through techniques like deep neural networks. It eschews explicit rules in favor of learning from the data, allowing it to generate novel content by discerning underlying patterns.
Learning Paradigm
Traditional AI: Predominantly utilizes supervised learning, relying on labeled data with provided inputs and corresponding outputs. Human annotations are vital for accurate learning and predictions.
Generative AI: Demonstrates proficiency in unsupervised learning scenarios, where the model excels in training on unlabeled data. It autonomously identifies underlying patterns and structures without explicit human guidance, showcasing adaptability in handling diverse datasets.
Model Type
Traditional AI: Leverages discriminative models, focused on distinguishing between different classes or categories of data. It excels in tasks like classifying images into specific categories based on predefined features.
Generative AI: Utilizes generative models, understanding the inherent probability distribution of the data. This enables it to create entirely new samples resembling the original data, such as generating realistic images through techniques like Generative Adversarial Networks (GANs).
Creativity and Adaptability
Traditional AI: Tailored for specific tasks, lacking creativity and adaptability beyond its programmed directives. It adheres to predefined rules without the capacity to generate new content or adapt to novel situations independently.
Generative AI: Exhibits creativity and adaptability by generating novel content. Whether it’s crafting images, texts, or music, Generative AI offers unique and creative outputs. Its ability to adapt to diverse data distributions enables it to align with new patterns or changes in input data, showcasing a dynamic and innovative approach.
While these two branches of AI are different, they are not mutually exclusive. In fact, AI programs like ChatGPT involve both conversational since it’s a chatbot, yet it’s also generative since it provides users with written content in response to prompts.
Key characteristics and capabilities of Generative AI
While ChatGPT and chatbots have quickly risen in popularity, other generative AI applications of this advanced technology are widely being implemented today.
One of the most common use cases of generative AI is text creation and summarization from user prompts. In addition to text creation, AI can also be used to generate a realistic image from text, called text-to-image conversions.Besides, code generation & completion and automated testing have quickly become one of the most prominent applications of generative AI.
Other sought-after applications of generative AI are video creation, audio generation, organization collaboration, ECM solutions, assisting with repetitive tasks, and checking customer contracts for compliance.
Future Trends and Developments in AI
In the upcoming years, we anticipate some exciting developments in Artificial Intelligence (AI) that will have a significant impact on various industries and our society.
A key trend to watch is Generative AI, where AI systems independently create things like art or text, bringing in a new era of creativity and personalization for different products and content.
Another noteworthy trend is the increase in Multimodal AI models. These models enable machines to understand and utilize different types of information such as text, images, and sound, promising more accurate and human-like interactions with AI systems.
Moreover, Workplace AI is on the horizon, focusing on automating tasks and aiding in decision-making to enhance efficiency in professional settings. The concept of Personalization at scale involves AI tailoring products and services based on individual preferences, offering more customized and enjoyable experiences.
The emergence of Digital humans and Digital Twinning introduces virtual counterparts created by AI, transforming simulations and interactions in various applications.
Finally, the growing emphasis on Ethics and Regulation highlights the need for establishing guidelines to ensure responsible AI use, prioritizing fairness, safety, and protection for individuals and society at large. It’s essential to stay vigilant about these trends as AI continues to shape our future.
Conclusion
Both technologies are integral to shaping the future and have found widespread acceptance in today’s society.
Conversational AI, with its roots in rule-based approaches, excels in customer service applications and virtual assistants, while Generative AI, employing a data-driven approach, empowers users to create new content, from text to images.
Recognizing these two branches of AI’s unique capabilities allows you to fully harness their potential as we navigate this exciting journey of innovation. Embracing AI is not just a technological leap but a strategic move toward enhancing efficiency, customer satisfaction, and overall business success.
In this era of technological transformation, businesses that embrace and integrate AI technologies stand to unlock new levels of innovation and success.