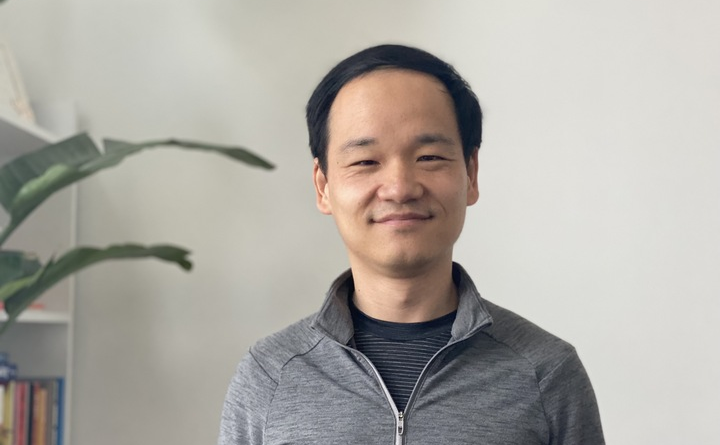
Michael Gao, MD, CEO and co-founder of SmarterDx
Applying artificial intelligence (AI) solutions in hospitals and health systems can be overwhelming for leaders due to AI’s complexity (it’s not one technology, but several) and rapid proliferation. This is in addition to the usual technology hurdles within healthcare that include data quality and accessibility, interoperability, and clinical validation and adoption. Finally, with any new solution, there’s the concern of return on investment: Will implementing the latest solutions pay off for my organization? How will it be measured?
Given these challenges, it’s advisable for organizations to initially apply AI in areas where there are fewer uncertainties and the value can be clearly ascertained. One such area is revenue cycle management (RCM), where the impact of AI can be easily measured, and hospital leaders can gain valuable insights into its potential. This knowledge can then be applied to more intricate and harder-to-measure domains like clinical care. To help RCM leaders build their AI strategy, here are three important steps: goal setting and impact measurement, assessing the true investment, and mitigating financial risk.
Setting Clear Goals and Measuring AI’s Impact
Before investing in any new technology, it’s essential to establish a clear understanding of the value you aim to achieve and how that value will be accurately measured and attributed. New initiatives often can deliver on multiple fronts; for example, efficiency gains, cost savings and revenue capture. However, it is crucial to identify the primary goal from the beginning and ensure the methodology for measurement and communication is clearly defined to organizational leadership.
For instance, many organizations target cost savings through automation, such as reducing full-time equivalents (FTEs); however, employees may be reassigned to different tasks, potentially offsetting the savings achieved through automation. And sometimes automation reconfigures the workflow and shaves minutes off the total time but still requires the same roles to support. For example, if you automate the turning of the wheels in a taxi, you’ve technically automated ~80% of the work, yet you still can’t replace the driver. They still need to spot and interact with passengers, press the brake and gas pedals, and relay the fare. Healthcare leaders should be cautious of introducing automation to workflows that don’t actually realize savings in terms of the total number of positions.
A clear example where value attribution is well-defined is net new revenue. For instance, AI can automate prebill review by scanning charts for additional documentation and coding opportunities after final coding but before billing. With this approach, each dollar can be matched to added documentation and diagnosis codes, allowing hospitals to clearly measure and attribute financial results.
Assessing the Real Investment in AI
When implementing an AI solution, the true cost goes beyond what is paid to the vendor. It is crucial to consider other associated expenses such as staff time, training, system replacement and IT costs. For example, a change in physician workflow that requires education, monitoring and training for a large number of physicians can quickly escalate implementation costs. Leaders need to account for these costs in addition to the vendor fees.
Another often-misjudged cost is IT integration. A common assumption is that costs increase with the volume of data, but the actual scalability is determined by the depth of integration. Sending gigabytes of data periodically as one-way file transfers is typically easier compared to real-time bidirectional integration of even a few data fields. For clinicians using AI tools at the bedside, seconds matter, and thus near-instant patient data transfers and workflow integration are necessary. AI solutions focused on revenue cycle processes, however, can often work with data that’s minutes or even hours old, thus decreasing the requirements for direct integration.
Mitigating Financial Risk
Lastly, RCM leaders should explore contracting options to minimize financial risk when adopting AI solutions. The most prevalent model in the past decade has been Software as a Service (SaaS), which typically involves multi-year lock-ins and upfront costs. New models are emerging that offer alternative structures that meaningfully reduce financial risk. Some solutions operate on a use-based model, where the organization only pays for actual usage. Another option is a contingency model where the vendor is paid a percentage of the cost savings achieved or new revenue generated. By sharing the risks and rewards with the vendor, organizations stand to gain more from their AI solutions.
Laying the Groundwork for Future AI Applications
AI solutions hold tremendous potential in transforming healthcare. RCM leaders who approach AI implementation with a thorough understanding of its benefits and potential challenges are better positioned to strategically utilize these solutions for both short-term and long-term gains. With a focus on RCM processes, organizations can leverage AI’s ability to analyze vast amounts of data quickly and effectively, while also mitigating common risks. By focusing on narrower applications, organizations can take actionable steps to reduce costs, increase revenue and reinvest those gains into optimizing patient care.
About Michael Gao, MD
Michael Gao, MD, is the co-founder and CEO of SmarterDx, a clinical AI company that provides hospitals with a prebill safety net to capture net revenue opportunities and advance care quality. Prior to SmarterDx, he was an Assistant Professor of Medicine at Weill Cornell and Medical Director for Transformation for NewYork-Presbyterian where he led AI and automation projects across clinical, operational, and revenue cycle domains. Dr. Gao completed his BS at the University of California Los Angeles, his MD at the University of Michigan, and his Internal Medicine Residency and Silverman Fellowship for Healthcare Innovation at NewYork-Presbyterian/Weill Cornell.