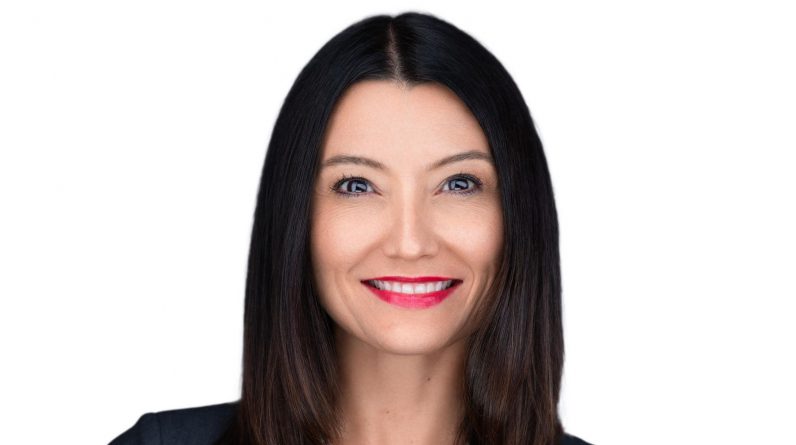
It certainly seems that all the talk today is about AI, and in particular Generative AI. However, much of the quiet work that AI is doing behind the scenes is much more mundane. And many times, the work is being done by less-intelligent forms of automation, based on approaches that leverage more from the world of rules-based low-code programming than from data-based machine learning.
That being said, the worlds of automation and intelligent systems are increasingly being used in tandem to power new levels of productivity and value. Indeed, these systems are powering real-world applications that provide value now, unlike so many of the high-glamor flash in the pan AI experiments people are toying with today that get press attention.
Keying into the differences between Automation and Intelligence
Christina Kucek, CAI
Christina Kucek, CAI
On a recent AI Today podcast, Christina Kucek, Executive Director of intelligent automation and practice lead at public sector-focused firm CAI shared insights into where automation and AI are heading. Christina has been assisting clients for the last five years in their automation journey from building hyperautomation teams for RPA and document extraction to AI-enabled systems and conversational AIs. CAI is a global technology services firm with over 8500 associates worldwide, and yearly revenue of over one billion dollars. One of their interesting differentiators is that they have a focus on recruiting and placing neurodivergent teams in technology roles.
One of the challenges in putting AI into place is focusing on real-world AI projects that provide actual, real-world value quickly. Christina explains, “I hear from a lot of our stakeholders that they want to be able to leverage the power of AI in their organization, but they are not sure how to get started. A lot of CEOs are saying, all right, what is our AI strategy? I need a strategy for 2024. What have you got for me? ‘Get it on my desk’ was the rush at the end of the year.”
However, a common challenge of getting AI into widespread adoption is the fact that AI is heavily data dependent. And data is not as available in the quantity or quality that’s needed. “Some of our clients have grown through acquisition, so their data challenges include some of the typical ones you see around volume, veracity, velocity, variety challenges that can be really overwhelming for them about their data, and just the quantity in all the disparate locations,” Christina explains.
She continues, “… and also a lack of data management strategy. It’s really hard to get your AI solutions implemented, your AI models built when you have so much data in so many different locations globally. So having that data management strategy and the data governance required to secure your data and make sure that permissions are set properly, those are real challenges that we see regularly, and it feels like a monumental task.”
AI may seem shiny on the surface. However, underneath that glitzy exterior is the not as exciting, but super necessary core of fundamental data management, data quality, data governance, and architecture. The key to success, Christina explains, is breaking down these problems into more “manageable pieces, manageable objectives, [so you can focus on the] rewarding and important work to leverage these tools development for business.” Like many other successful AI project managers, Christina is CPMAI certified, a rapidly emerging best practice framework and certification for running and managing AI projects.
As part of the CPMAI methodology, the first phase is developing a Business Understanding that translates to a short term, implementable, real-world AI-relevant project that delivers a well-identified short-term positive return. Christina explains, “What is our AI plan? What is our rollout? How are we using this? Everybody’s talking about the how. How LLMs work, how neural networks work, how cool the technology is, and not talking about the business case where businesses can get real value from implementing these technologies.”
How AI is Changing Automation
There is still a lot of confusion about the difference between automation and intelligence in the industry, especially among C-level executives Christina explains. “I don’t want to say how many times [I’ve had to explain] the difference between robotics, process automation, and something that’s actually intelligent. RPA (Robotic Process Automation) is the hands, not the brains, of the solution. Using AI adds intelligence by providing intelligent document extraction, machine learning models, and more”.
“As a director, as a manager, I needed to ensure our clients that we were able to consistently deliver great AI solutions. I’m not in the business of creating vaporware. My biggest nightmare is to spend months or even a year on a project, and then it’s just not of value to our business stakeholders.”
As AI tools and automation tools both become easier to adopt and use, they’re becoming more incorporated into products used daily. The technology part is becoming more accessible, but it’s the harder things around changing people and processes that often get in the way of project success. People are much harder to change than technology. Processes are some of the most difficult things to change, especially if they’re embedded in the way that things are done.
As a final note, Christina shares one of her most important lessons learned: “Working iteratively is huge. Not trying to boil the ocean. I’ve seen a lot of projects balloon as stakeholders start imagining what they can do with AI. We start the discussion, we start that discovery, and they start imagining what’s possible with their data and AI and the scope just balloons out of control. Just being able to focus on what goal and the minimum viable product, the one thing that we want to accomplish and then really building on that, I would say that is the key need today.”
She continues, “Keep the human in the loop. Feedback can be perilous, and this was a hard pill for my team to swallow, and we kept seeing it over and over. To clarify, if you’re allowing your users to provide feedback, and if they are not consistent and diligent and accurate about their feedback, depending on what data elements you’re capturing in the feedback loop, then your AI solution can degrade really quickly.”
(Disclosure: I’m a co-host of the AI Today podcast and CPMAI lead instructor)