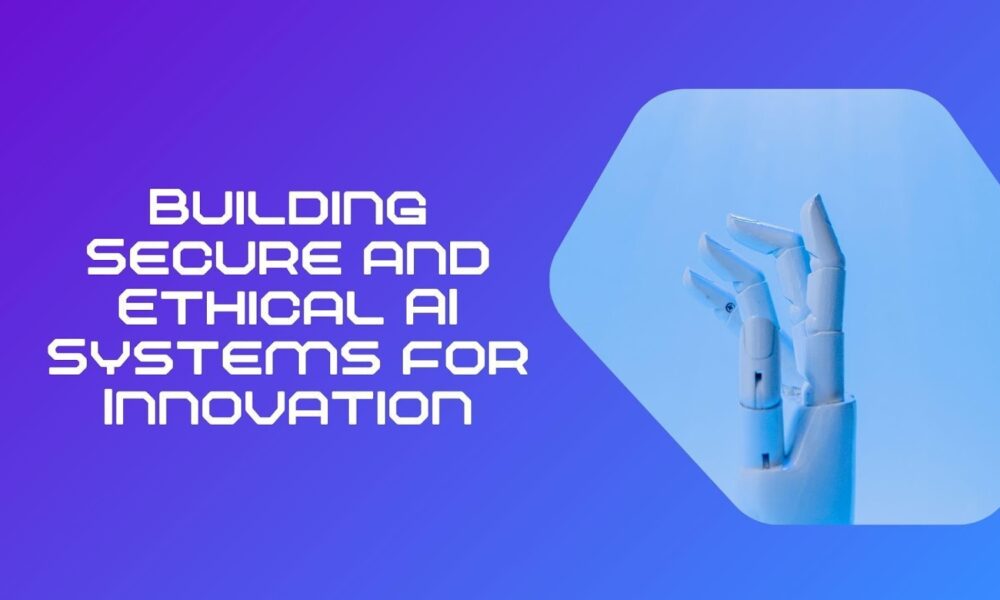
In this modern era, as artificial intelligence (AI) adoption grows, ensuring security and ethical governance has become essential. Rajkumar Sukumar explores secure AI systems, focusing on data security, privacy protection, and ethical AI governance. His research highlights encryption protocols, access control mechanisms, blockchain, and privacy-preserving AI models that protect sensitive data while ensuring regulatory compliance. With AI’s increasing role in decision-making, organizations must integrate strong security frameworks to address bias, transparency, and ethical concerns. Implementing these measures not only strengthens AI reliability but also fosters trust in AI-driven systems across industries.
The Need for Secure and Ethical AI
AI enhances efficiency but introduces risks related to data integrity, privacy, and algorithmic fairness. Research indicates that 72% of organizations identify AI security and ethics as primary challenges. Without proper safeguards, AI systems can be vulnerable to data breaches and biased decision-making, leading to regulatory penalties and reputational damage.
Data Security in AI Systems
Encryption and Access Control
AI security relies on encryption techniques to prevent unauthorized access. Quantum-resistant encryption ensures confidentiality in distributed environments. AI-powered access control models using role-based (RBAC) and attribute-based (ABAC) frameworks have reduced security breaches by 78%, improving automated data protection.
Data Masking and Tokenization
Tokenization and data masking enable AI models to process anonymized data without exposing sensitive information. These techniques reduce breach risks by 76% while maintaining data utility for analysis.
Privacy-Preserving AI Models
Federated Learning for Secure AI Training
Federated learning trains AI models across decentralized data sources, preventing raw data exposure. This approach enhances privacy while maintaining high accuracy, particularly in healthcare and financial applications.
Differential Privacy and Homomorphic Encryption
Differential privacy techniques add statistical noise to datasets to anonymize individual records. Homomorphic encryption allows computations on encrypted data, enhancing security with minimal performance trade-offs.
Ensuring Data Integrity and Authenticity
Blockchain for Secure AI Data Management
Blockchain technology strengthens AI data integrity by preventing unauthorized modifications. Blockchain-integrated AI systems reduce data manipulation incidents by 94.3%, improving data verification processes.
AI-Powered Anomaly Detection
Anomaly detection models analyze access patterns and detect threats with 98.9% accuracy. These AI-driven security frameworks identify risks faster than traditional methods, reducing operational threats.
Ethical AI Governance and Accountability
Bias Detection and Fairness Audits
Fairness audits and bias detection tools assess AI training data and decision outputs. Organizations implementing these measures report a 44% reduction in algorithmic bias, improving transparency and accountability.
Explainability and AI Oversight
Explainable AI (XAI) frameworks provide insights into AI decision-making processes. Companies adopting XAI experience a 59% improvement in stakeholder trust and regulatory compliance.
AI Ethics Committees for Responsible AI Use
AI ethics committees ensure continuous oversight, reducing compliance risks by 64%. These teams help enforce ethical AI policies and align AI decisions with organizational values.
Security-Aware AI Development
Secure Model Training and Authentication
AI models trained in secure environments experience an 88.5% reduction in adversarial attacks. Machine learning enhances authentication processes, reducing unauthorized access attempts by 96.7%.
Continuous AI Security Monitoring
AI-powered security monitoring systems improve real-time threat detection, increasing zero-day attack prevention rates by 82.6%. These systems analyze AI model behavior to identify vulnerabilities.
Future Directions in AI Security and Ethics
Decentralized AI for Privacy Protection
Decentralized AI frameworks reduce security vulnerabilities by 78%, ensuring privacy while maintaining performance in distributed networks.
AI for Compliance and Regulatory Automation
AI-driven compliance tools automate adherence to data protection laws, reducing regulatory violations by 51% and improving audit efficiency.
Advancements in AI-Driven Security
Emerging security solutions, such as adversarial training and real-time AI threat intelligence, reduce security incidents by 73.4%, enhancing resilience against evolving cyber threats.
In conclusion, Rajkumar Sukumar’s research emphasizes the importance of security and ethical governance in AI. By integrating encryption, privacy-preserving AI models, and AI-driven security frameworks, organizations can protect AI systems while ensuring transparency and accountability. As AI continues to evolve, prioritizing security and ethical governance will be essential for fostering trust, mitigating risks, and enabling responsible AI innovation. These frameworks will shape the future of AI, ensuring it serves both business and societal needs while maintaining privacy and security.