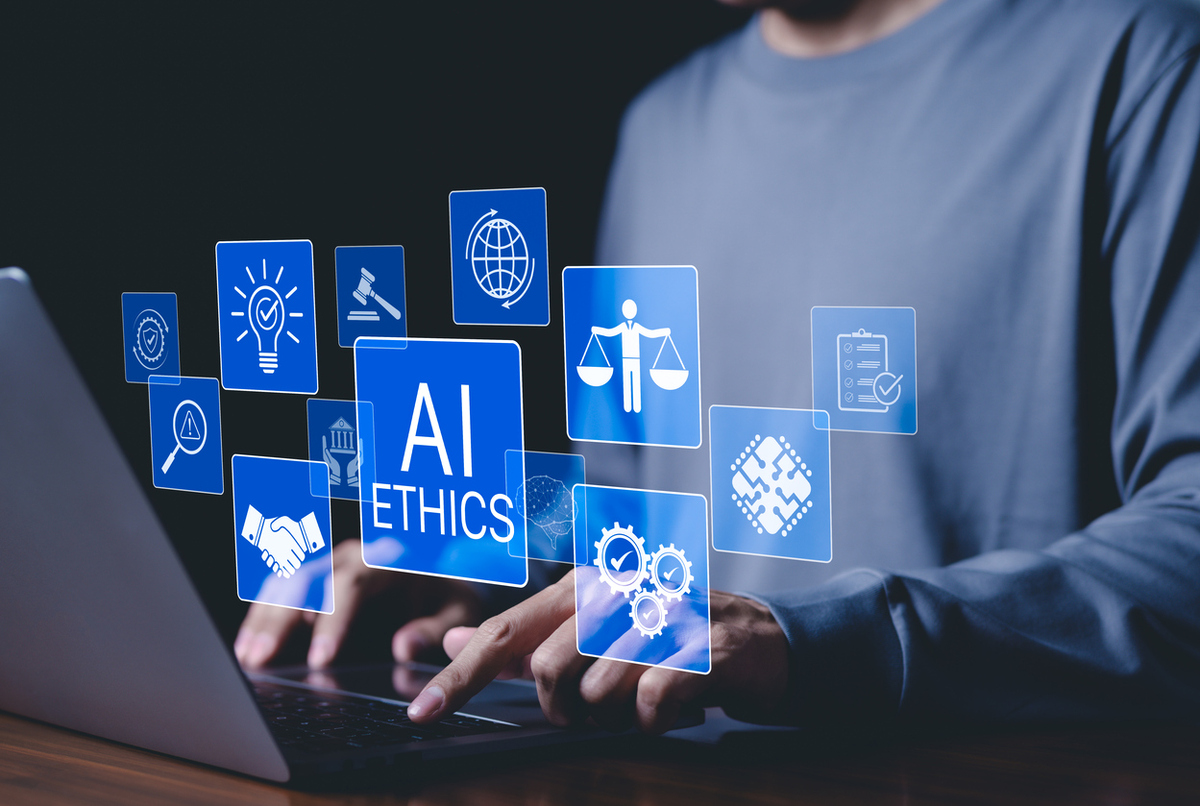
Developing and using AI tools responsibly is central to many organisations’ technology governance. A few legal frameworks exist that provide requirements around the socially acceptable use of AI, such as the EU’s AI Act, criticised by many as a block on innovation, and China’s pragmatic regulations on generative AI.
However, regulatory thinking is still in flux, with many leading countries such as the USA and UK adopting a wait-and-see approach. Because of this, comparing ethical frameworks from prominent organisations can help one better understand best practices during this period of rapid change.
To do this, Business Reporter examined six governmental or supra-governmental AI ethics frameworks (UNESCO, World Economic Forum, European Union, Council of Europe, OECD and UK government). Many of these frameworks show similarities, although there is certainly not a full consensus on what is appropriate. To demonstrate this, the table below shows which principles are included in each framework and the “headline” descriptions that are used in each.
Principle |
UNESCO |
WEF |
EU |
CoE |
OECD |
UK Gov |
Fairness |
Diversity and inclusiveness |
Fairness |
Diversity, non-discrimination and fairness |
Equality and non-discrimination |
Human rights including …. fairness |
Deliver fair services for all users |
Accountability |
|
Accountability |
Accountability |
Accountability and responsibility |
Accountability |
Be clear who is responsible |
Transparency |
|
Interpretability (explainability, transparency, provability) |
Transparency |
Transparency and oversight |
Transparency and explainability |
Help users understand how it impacts them |
Lawfulness |
|
Lawfulness and compliance |
|
|
|
Ensure you’re compliant with the law |
Oversight |
|
Human agency |
Human agency and oversight |
Transparency and oversight |
|
|
Utility, accuracy |
|
|
|
Reliability |
|
Build something future-proof |
Diligence in development |
|
|
|
|
|
Test to avoid unwanted outcomes |
Autonomy, safety and human rights |
Human rights Peace and justice |
Safety |
Robustness and safety |
Safe innovation Human dignity and individual autonomy |
Human rights and democratic values |
Handle data safely and protect citizens’ interests |
System security and privacy |
|
Reliability, robustness, security Data privacy |
Privacy and data governance |
Privacy and personal data protection |
Human rights … including … privacy Robustness, security and safety |
Handle data safely and protect citizens’ interests |
Sustainability, social good |
Ecosystem flourishing |
Beneficial AI |
Societal and environmental wellbeing |
|
Inclusive growth, sustainable development and wellbeing |
|
Table 1. Governmental/supra-governmental AI ethics frameworks
In addition, we looked at frameworks from organisations more closely related to industry: two standards organisations (IEEE and ISO), an academic specialist (the Turing Institute) and three leading technology businesses (IBM, Google and Microsoft). These are shown below.
Principle |
IEE |
ISO |
Turing Inst |
IBM |
|
Microsoft |
Fairness |
|
Fairness Inclusiveness |
Fairness |
Fairness |
… avoid unfair bias |
Fairness Inclusiveness |
Accountability |
|
Accountability |
Accountability |
|
|
Accountability |
Transparency |
Transparency Education and awareness |
Transparency |
Transparency |
Explainability Transparency |
|
Transparency |
Lawfulness |
… address legal issues of culpability |
|
|
|
|
|
Oversight |
|
|
|
|
… appropriate human oversight |
|
Utility, accuracy |
|
|
|
|
… align with user goals |
|
Diligence in development |
|
|
|
|
… design, testing, monitoring… to mitigate unintended or harmful outcomes |
Reliability |
Autonomy, safety and human rights |
Respects human rights, freedoms, human dignity and cultural diversity |
Non-maleficence |
|
|
… align with … human rights |
Safety |
System security and privacy |
Verifiably safe and secure |
Privacy Robustness |
|
Robustness Privacy |
… approaches to advance safety and security … privacy and security, and respecting intellectual property rights |
Privacy and security |
Sustainability, social good |
… maximum benefit to humanity and the natural environment. |
|
Sustainability |
|
… social responsibility |
|
Table 2: Commercial/quasi-commercial AI ethics frameworks
Please note that the tables above represent only a “shorthand” analysis of the content of the different frameworks; in all cases, there is a considerable amount of detail that underpins each area which may not be accurately represented by the headline words used.
As can be seen it is relatively simple to create nine different areas of ethical concern into which the various frameworks can be subdivided. So, let’s take a look at each of these areas.
Fairness
The principle that AI should be fair is included in almost all frameworks. This can be considered to include the avoidance of unwanted bias, the production of outcomes that avoid unjustified discrimination and the availability of the system to all authorised stakeholders (“diversity” and “inclusiveness” are words that are sometimes used). Many AI systems are designed to discriminate for commercial or other reasons – but as well as being lawful this must be achieved equitably. Transparency in the aims and methods of the AI system will help here.
One notable absence is the discussion of accessibility: this could perhaps be considered a technical issue; however, putting accessibility to all authorised and intended users, irrespective of physical or mental disability, should be at the heart of achieving fairness.
Accountability
Ethical accountability should involve the identification of a person or small group of people who are accountable (morally and legally) to internal and external stakeholders (including law enforcement) for the outputs of the AI system. It will never be ethical to devolve accountability to a system, organisation or large group of people where individuals can escape the consequences of malicious or negligent AI use. Even in a highly automated system, such as traffic control, there should be a human being who is ultimately in charge.
Note that responsibility and accountability are two very different things. An individual may be responsible for making a decision relating to an AI system, but another, probably more senior, individual should be accountable for their decision, meaning that they should, for example, ensure that the responsible person has sufficient resources, including information and authority, to make an appropriate decision.
Transparency
The area of transparency is both very important yet still only partially understood. At its simplest, it means ensuring users of a system know that AI is involved in generating outputs and ideally explaining to them how the use of AI has affected them.
However, transparency can also involve more complex explanations of how a system is operating. Words such as “explainability”, “understandability”, “interpretability” and “provability” are used: these relate to the degree to which users (typically professional users) can trust the outputs. In these cases, transparency involves the provision of information about how decisions were arrived at and what data, assumptions and calculations were made in reaching the decision. Where machine learning is involved, transparency can be hard – even impossible – to achieve and other methods to indicate the trustworthiness of the outputs (such as previous accuracy or utility) must be sought.
Lawfulness
Compliance with the law may not equate to ethical behaviour but lawfulness or compliance is mentioned in several frameworks, and there are obvious reasons why any organisation needs to comply with laws and industry regulations. From an ethics perspective, these laws are generally in place to protect people or wider society from harm and so they may help organisations that lack expertise find a way of acting ethically. In addition, complying with legal requirements can protect employees and other stakeholders from legal problems.
A key consideration here is whether organisations can be trusted to regulate themselves or whether laws are needed. Issues in social media and online harm might suggest that many “big tech” organisations are not prepared to self-regulate sufficiently and therefore laws are needed to force them to behave ethically. But there is a balance that should be struck between erecting guardrails to prevent a dystopian future from happening and leaving room for innovation and increased prosperity.
Human oversight
Oversight might be considered a practical way of achieving ethical outcomes rather than an ethical requirement in its own right, perhaps a subset of accountability. But it does seem important that human beings are involved in most AI systems. This could be to sense-check outputs (possibly as part of a quality assurance programme) or it could be to act as a resource for users who are unhappy with outcomes so they can question them, and perhaps be advised about how a different outcome can be reached. This might be particularly relevant in financial services, social services and healthcare.
Utility and accuracy
Does the AI system do what we designed it to do? This is a question any organisation using AI needs to ask. And this is not just from a commercial perspective. Users have a right, especially if they have paid for a service, to assume that it will deliver what they have been promised. The utility of a system (sometimes expressed as “reliability” or “aligning with user goals”) is important. Linking to transparency (and particularly explainability and interpretability), systems need to be monitored continuously so that the quality of the outputs can be measured and, if necessary, the system recalibrated. An interesting aspect of this is the UK government’s requirement for systems to be future-proofed, something which all organisations using AI should consider if they wish to maintain utility.
Accuracy is closely linked to utility, in that inaccurate outputs, often caused by insufficient training data, will be of little use to users and may well even be harmful.
Diligence
Diligence, especially diligence in looking for and mitigating risks, is an essential part of responsible AI use. Care should be taken that the AI system is delivering, or will deliver, what it is designed to do, at all stages of development and operation. This is not something that is frequently covered in AI frameworks (kudos to UK government and Google), but we believe it should be. A failure to test outputs at all stages of development and use is negligent and could easily result in harm.
Agency and human rights
As might be expected, there is a focus on human rights in almost all of the reviewed. Safety (physical and mental) is an obvious part of that. Less often mentioned is agency – the avoidance of using AI systems to force people to act in ways they don’t want to or to take away their own agency and choice. Human agency is, at least currently, central to the ethical operation of autonomous systems such as vehicles that have the potential to harm people and property, and where it is therefore considered appropriate for a human to be able to take the final decision in a dangerous situation: in this respect agency is related to accountability and oversight.
Security and privacy
AI systems need to be secure. This means that their processes (such as algorithms) should be free from unauthorised interference and their data should be protected against malicious attacks designed to poison it as a way of altering the outputs.
A key part of security is the protection of any personal data that the system may hold or be capable of creating. Clearly this will need to be protected for GDPR purposes. However, if an AI system is subverted in such a way as to generate false and malicious personal data this would not just be a failure of privacy, it would also be a failure of the requirement for utility and accuracy.
Social good
It could be argued that the creation of social good has no place in a framework for AI ethics aimed at commercial organisations: suggestions that AI should be “beneficial to all” or provide “maximum benefit to humanity” might seem a little naïve. However, the avoidance of social harm definitely does have a place in an ethics framework. This harm could range from misinformation that affects democracy or causes unwarranted panic, through to systems that cause environmental harm – for instance, through excessive or unnecessary energy use. This is perhaps one of the most difficult areas to address, as an AI system that causes job losses could be considered socially harmful, at least in the short term. Although there are plenty of arguments that can be made for increased productivity in organisations as a route to greater general wealth and wellbeing.
The way forward for responsible AI
As matters stand currently, there are some very useful examples of ethical frameworks for the responsible use of AI that organisations can co-opt for their own use. The suggestions above (which have the happy mnemonic of SAD LOUD ASS!) can be a useful starting point for anyone trying to structure an ethical approach to the governance of AI.
We are not suggesting that all of these suggestions are essential, nor that they should be in the way that we have expressed them, but we would propose that any organisation that decides to ignore any of these principles should have a good reason that they can present to their stakeholders.