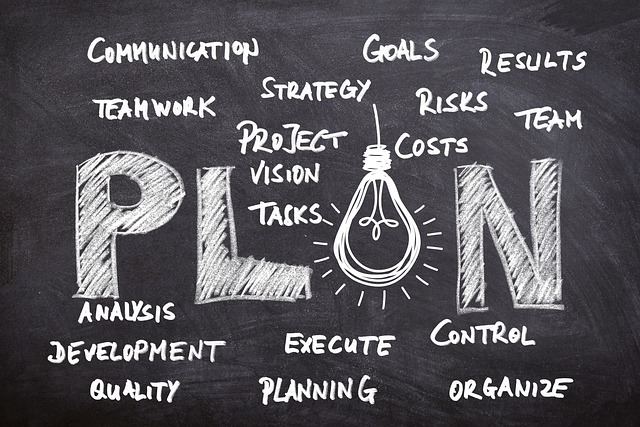
More bullish prognostications on recent generative and agentic AI progress suggest we might soon be lounging around in deck chairs while AI (Artificial Intelligence) is doing all the work we were supposed to do in the past. Operations will be autonomous, and AIs will be designing, orchestrating, running and improving all these processes on the fly.
But this is absurd, says Bernhard Schaffrik, Principal Analyst, Forrester, who witnessed similar rosy promises with Robotic Process Automation (RPA), low-code development, and intelligent document processing (IDP). Instead, new agentic AI tools will deliver substantial business value in the long run, but differently than most executives he talks to imagine.
For starters, replacing your existing working RPA or IDP (Intelligent Document Processing) automations with new agentic tools that are slower, more expensive, and hallucinate is a bad idea. Schaffrik watched many firms suffer when they imagined they could with RPA, and the damage will be similar with the agentic stuff.
Also, it’s important to level set expectations with the new crop of tools. Mostly, enterprises are doing a lot of pilots and trying to orchestrate some processes. Forrester believes that a maximum of 1% of core business processes will be orchestrated by generative AI this year. A core issue is trust, since these systems tend to hallucinate, and it’s hard to troubleshoot bias. Schaffrik says:
Even if you keep these agents and LLMs [Large Language Models] somewhat grounded and within certain guardrails, we haven’t found a senior decision maker yet who would agree to having generative AI fully orchestrate, maneuver, run, or improve a customer-facing end-to-end process.
A better place to focus is to use these new tools to augment people in building better processes rather than outright replacing people or automations. For example, he sees a generative AI impact in improving process design by accelerating human process designers, increasing code quality, and simplifying data integration.
Eventually, these tools and supporting infrastructure will get better at more dynamic process orchestration to improve flexibility. However, Schaffrik is convinced the deterministic process orchestration tools today will remain the technology of choice for years to come, so all the autonomous, unstructured workflow patterns and processes will have to wait. So, how do we get there?
The ROI problem
One problem Schaffrik comes across is when technology decision makers frame the ROI of AI projects. In its simplest form, this is just the value versus costs. Most of his conversational partners focus too much on costs, risk, effort, and uncertainty. How do you even assess the risk of biases and hallucinations?
After a while of listening, he flips the questions around with questions like:
- Have you thought about the value generation?
- What is the value promise of your generative AI project for which you want to calculate the ROI?
The usual response tends to be about saving many hours for many people. This is good, but it completely ignores the disruptive potential of generative AI. It’s not just another technology that allows efficiency. It can do so much more. In some way, this is to be expected since most existing solutions are tactical. They are happening in silos, which limits their applicability to certain departments. This is all good stuff, but it misses the disruptive potential of generative AI.
Cutting across silos
Realizing this disruptive potential will require finding ways to cut across cultural, organizational, political, and technical barriers and silos. Schaffrik says this is a great vision but a tough challenge:
Now you might say, ‘it’s very easy for an analyst to stand here and break silos. We have been trying this for decades, and it has never worked, and it won’t ever work.’
He hopes this time it might be different. For one thing, uncertain economic pressure has been a wake-up call for many leaders of companies of all sizes. The traditional approach has been to kick off another cost-cutting or efficiency program. But this time, many leaders are realizing that these programs can’t cope with the uncertainty of quickly shifting tariffs, unprecedented climate disasters, and geopolitical restructuring. Responding to these novel circumstances requires:
Re-inventing their operating models, thinking across the silos. But of course, it’s very hard to do because they have unlearned that. And again, it’s very hard to augment that with the geopolitical climate we are in that demands a higher level of adaptiveness, of resilience and cross silo collaboration. Imagine a situation where salespeople being with the customers, sensing that their sentiments are changing slightly because of geopolitics or some other events, their behaviors are slightly changing. And if they were able to send these signals back into the organization, and immediately, people in Marketing, controlling Finance, and the different business lines were able to adapt. That’s true, cross silo, collaboration in real-time.
Also, Gen Z workers are just starting to enter the workforce. The majority don’t accept silos. Either they move to another company or mentally quit and stay with the company. As a result, the company does not unlock their creativity and ingenuity. These people keep on working because it pays the bills.
A third aspect is that organizations increasingly realize that generative AI needs lots of data to avoid bias, remain relevant, and not hallucinate too much. This means breaking data silos across Marketing, Sales, Production, and other departments to stay relevant. The technologies for doing this are finally ready.
Overcoming automation limits
RPA and IDP both work great for simple processes. But they hit limits when automating complex processes. Similarly, process automation initiatives are great but require efforts to map all the variants, exceptions, and errors. This means you have to anticipate at design time what will happen at run time, which is hard to do and time-consuming. As a result, most companies only automated the happy path.
Schaffrik suggests a better approach:
Now, what I think will help us to break silos is process orchestration from a technological point of view. Now opening up the whole thing allows you to put all the technologies on a process string, be it RPA bots, applications, API calls, human tasks, the famous AI agents and all the other beautiful things along an end-to-end process. And you don’t need to map out everything at design time. You don’t need to anticipate everything at design time, because these things have states, and there is a powerful state engine that can orchestrate them, acting upon rules.
But at runtime, things might behave differently from design time, especially AI agents and other agentic automation technologies, as they are great at handling ambiguities at run time. This gives you even more opportunity to operate and automate very complex end-to-end processes.
Process orchestration nirvana
This all sounds pretty good and doable when the process orchestration uses traditional deterministic approaches, since the probabilistic generative AI approaches are currently only applicable to 1% of processes. Schaffrik helpfully breaks this journey towards process orchestration nirvana across four lenses.
First, you must deal with these agents differently from classic applications and deterministic RPA bots. He suggests treating them like human new hires. You coach them, see how they perform, give them feedback and track their progress until you are fairly confident about their performance.
Second, keep in mind that AI is not good for everything. Don’t replace working automations. Stick to the existing automation tools for deterministic automations. The AI stuff is a better fit when it’s easier to describe the goal than the steps, when you have a lot of prior data, and when providing some human oversight is feasible.
Third, it’s important to understand the level of trust in the orchestration engine and automation technologies. These days, enterprises can see some value when using deterministic orchestration engines to manage processes that involve non-deterministic agents. This extends the scope of automation to support use cases not feasible with current tools, with well-defined guardrails. It will be a few more years before we build trust in orchestration engines that run the risk of quickly spinning up a lot of bad processes, increasing costs, and eroding customer goodwill.
Fourth, business strategy defines process strategy. The business strategy and desired competitive advantage dictate the process strategy. This could mean standardized/low cost at one end vs. differentiated/high touch at the other. This, in turn, impacts the choice of automation technology. Process automation tools suit low-variability, cost-focused processes, while process orchestration is better for high-variability, experience-focused processes.
My take
The conventional wisdom is that OpenAI ushered in a seminal new approach to the generative AI era in 2021 with ChatGPT. However, their real innovation was cobbling many existing things into a better product, much like Steve Jobs did with the iPhone. Four years later, all of the new breakthroughs in reasoning and agentic AI seem like incremental updates to a novel approach introduced in 2017 that continues to hallucinate and costs more to do what existing automation tech does more reliably and quickly. At the same time, there is tremendous value in some of these new things beyond just trying to reduce headcount. But it’s going to take a few more years to productize those.
Schaffrik brings a practical perspective to navigating the chasm between the promise of a more autonomous enterprise and the practical realities relating to trust, business value, and process improvement. Going back to his original ‘absurd’ vision, what else might have to change for people to believe that these automations might actually mean more time lounging in deck chairs, or at least engaging with our communities in meaningful ways? Whatever that looks like, I am pretty sure its going to be a bit more challenging than breaking silos.