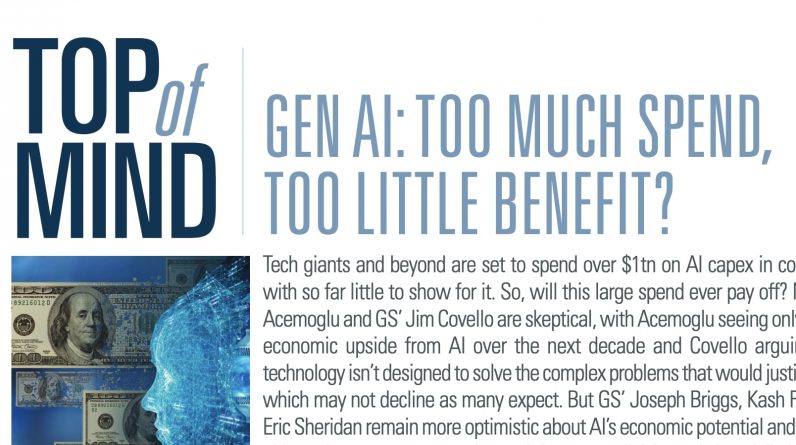
An MIT Professor of labor and productivity analysis, Daron Acemoglu, makes a case that AI progress to boosting world GDP growth will be less and transformative change will take longer. His case is not from a deep understanding or analysis of AI. He is looking at the comparative case of the internet. How long did the internet take to transform the world economy.
There are huge issues to get to global economy transforming levels of change. There are flaws with his analysis. His analysis ignores this wave of AI and neural networks solving humanoid robotics and self driving cars or advancing robotics. His analysis assumes that costly vision systems using brute force GPU compute resources are the only methods that are crudely applied without altering the business model or structure of the economy. His analysis focuses on OpenAI Chatgpt and its competing large language models. He assumes no breakthroughs and that LLMs do not have breakthroughs from scaling.
He says AI will only moderately improve some backend business processes.
Summarizing Daron’s Argument and Papers
Here is Daron Acemoglu 31 page report published with Goldman Sachs.
Daron forecasts a ~0.5% increase in productivity and ~1% increase in GDP in the next 10 years vs. GS economists’ estimates of a ~9% increase in
productivity and 6.1% increase in GDP. Why are you less optimistic on AI’s potential economic impacts?
Recent studies estimate cost savings from the use of AI ranging from 10% to 60%, yet you [Daron] assume only around 30% cost savings. Why is that?
Daron began with Eloundou et al.’s [OpenAI team] comprehensive study that found that the combination of generative AI, other AI technology, and computer vision could transform slightly over 20% of value-added tasks in the production process. But that’s a timeless prediction. Daron then looked at another study by Thompson et al. on a subset of these technologies—computer vision—which estimates that around a quarter of tasks that this technology can perform could be cost-effectively automated within 10 years.
The Thomson MIT team make the case that some tasks would be too cheap to replace with an AI vision system. A simple hypothetical example makes clear why these considerations are so important. Consider a small bakery evaluating whether to automate with computer vision. Bureau of Labor Statistics O*NET data imply that checking food quality comprises roughly 6% of the duties of a baker. A small bakery with five bakers making typical salaries ($48,000 each per year), thus has potential labor savings from automating this task of $14,000 per year. This amount is far less than the cost of developing, deploying and maintaining a computer vision system and so we would conclude that it is not economical to substitute human labor with an AI system at this bakery.
Nextbigfuture notes that the AI systems need to target the bigger opportunities with a business model that provides the benefits. AI chat systems could replace 80-100% of the internet searches that Google makes $200 billion per year performing. Vision systems with extensive GPU AI compute is not the only approach to applying AI.
Daron Acemoglu: Of the three detailed studies published on AI-related costs, I chose to exclude the one with the highest cost savings—Peng et al. estimates of 56%—because the task in the study that AI technology so markedly improved was notably simple. It seems unlikely that other, more complex, tasks will be affected as much. Specifically, the study focuses on time savings incurred by utilizing AI technology—in this case, GitHub Copilot—for programmers to write simple subroutines in HTML, a task for which GitHub Copilot had been extensively trained. My sense is that such cost savings won’t translate to more complex, open-ended tasks like summarizing texts, where more than one right answer exists. So, I excluded this study from my cost-savings estimate and instead averaged the savings from the other two studies.
Daron Acemoglu has a 57 page paper, The Simple Macroeconomics of AI.
Daron purposely excluded robotics and assumes no significant changes in the large language model approaches. He assumes the size of different comoponents of the world economy stay the same for the next ten years.
Tech giants and beyond are set to spend over $1tn on AI capex in coming years, with so far little to show for it. So, will this large spend ever pay off? MIT’s Daron Acemoglu and GS’ Jim Covello are skeptical, with Acemoglu seeing only limited US economic upside from AI over the next decade and Covello arguing that the technology isn’t designed to solve the complex problems that would justify the costs, which may not decline as many expect. But GS’ Joseph Briggs, Kash Rangan, and Eric Sheridan remain more optimistic about AI’s economic potential and its ability to ultimately generate returns beyond the current “picks and shovels” phase, even if AI’s killer application has yet to emerge. And even if it does, we explore whether the current chips shortage (with GS’ Toshiya Hari) and looming power shortage (with Cloverleaf Infrastructure’s Brian Janous) will constrain AI growth. But despite these concerns and constraints, we still see room for the AI theme to run, either because AI starts to deliver on its promise, or because bubbles take a long time to burst.
Acemoglu paper – It starts from a task-based model of AI’s effects, working through automation and task complementarities. So long as AI’s microeconomic effects are driven by cost savings/productivity improvements at the task level, its macroeconomic consequences will be given by a version of Hulten’s theorem: GDP and aggregate productivity gains can be estimated by what fraction of tasks are impacted and average task-level cost savings. Using existing estimates on exposure to AI and productivity improvements at the task level, these macroeconomic effects appear nontrivial but modest—no more than a 0.66% increase in total factor productivity (TFP) over 10 years. The paper then argues that even these estimates could be exaggerated, because early evidence is from easy-to-learn tasks, whereas some of the future effects will come from hard-to-learn tasks, where there are many context-dependent factors affecting decision-making and no objective outcome measures from which to learn successful performance. Consequently, predicted TFP gains over the next 10 years are even more modest and are predicted to be less than 0.53%. I also explore AI’s wage and inequality effects. I show theoretically that even when AI improves the productivity of low-skill workers in certain tasks (without creating new tasks for them), this may increase rather than reduce inequality. Empirically, I find that AI advances are unlikely to increase inequality as much as previous automation technologies because their impact is more equally distributed across demographic groups, but there is also no evidence that AI will reduce labor income inequality. Instead, AI is predicted to widen the gap between capital and labor income. Finally, some of the new tasks created by AI may have negative social value (such as design of algorithms for online manipulation), and I discuss how to incorporate the macroeconomic effects of new tasks that may have negative social value.
Daron Acemoglu is Institute Professor at MIT and has written several books, including Why Nations Fail: The Origins of Power, Prosperity, and Poverty and his latest, Power and Progress: Our Thousand-Year Struggle Over Technology and Prosperity. Below, he argues that the upside to US productivity and growth from generative AI technology over the next decade—and perhaps beyond—will likely be more limited than many expect.
AI will have implications for the macroeconomy, productivity, wages and inequality, but all of them are very hard to predict. This has not stopped a series of forecasts over the last year, often centering on the productivity gains that AI will trigger. Some experts believe that truly transformative implications, including artificial general intelligence (AGI) enabling AI to perform essentially all human tasks, could be around the corner. Other forecasters are more grounded, but still predict big effects on output. Goldman Sachs (2023) predicts a 7% increase in global GDP, equivalent to $7 trillion, and a 1.5% per annum increase in US productivity growth over a 10-year period. Recent McKinsey Global Institute (2023) forecasts suggest that generative AI could offer a boost as large as $17.1 to $25.6 trillion to the global economy, on top of the earlier estimates of economic growth from increased work automation. They reckon that the overall impact of AI and other automation technologies could produce up to a 1.5 − 3.4 percentage point rise in average annual GDP growth in advanced economies over the coming decade.
In this paper, I [Daron] focus on the first two channels, though I [Daron] also discuss how new tasks enabled by AI can have positive or negative effects. I do not dwell on deepening of automation, because the tasks impacted by (generative) AI are different than those automated by the
previous wave of digital technologies, such as robotics, advanced manufacturing equipment and software systems. I also do not discuss how AI can have revolutionary effects by changing the process of science (a possibility illustrated by neural network-enabled advances in protein folding and new crystal structures discovered by the Google subsidiary DeepMind), because large-scale advances of this sort do not seem likely within the 10-year time frame and many current discussions focus on automation and task complementarities.
Many production workers today, including electricians, repair workers, plumbers, nurses, educators, clerical workers, and increasingly many blue-collar workers in factories, are engaged in problem-solving tasks. These tasks require real-time, context-dependent and reliable information. For instance, an electrician dealing with the malfunctioning of advanced equipment or a short-circuit on the electricity grid will be hampered from solving these problems because he or she does not have sufficient expertise and the appropriate information for troubleshooting. Reliable information that can be provided quickly by generative AI tools can lead to significant improvements in productivity. Similarly, generative AI in class- rooms can lead to a major reorganization of how teaching takes place, with greater levels of personalization, as these tools help teachers identify specific aspects of the curriculum with which subgroups of students are having problems and propose new context-dependent teaching strategies.
My assessment is that there are indeed much bigger gains to be had from generative AI, which is a promising technology, but these gains will remain elusive unless there is a fundamental reorientation of the industry, including perhaps a major change in the architecture of the most common generative AI models, such as the LLMs, in order to focus on reliable information that can increase the marginal productivity of different kinds of workers, rather than prioritizing the development of general human-like conversational tools. The general-purpose nature of the current approach to generative AI could be ill-suited for providing such reliable information. To put it simply, it remains an open question whether we need foundation models (or the current kind of LLMs) that can engage in human-like conversations and write Shakespearean sonnets if what we want is reliable information useful for educators, healthcare professionals, electricians, plumbers and other craft workers
Brian Wang is a Futurist Thought Leader and a popular Science blogger with 1 million readers per month. His blog Nextbigfuture.com is ranked #1 Science News Blog. It covers many disruptive technology and trends including Space, Robotics, Artificial Intelligence, Medicine, Anti-aging Biotechnology, and Nanotechnology.
Known for identifying cutting edge technologies, he is currently a Co-Founder of a startup and fundraiser for high potential early-stage companies. He is the Head of Research for Allocations for deep technology investments and an Angel Investor at Space Angels.
A frequent speaker at corporations, he has been a TEDx speaker, a Singularity University speaker and guest at numerous interviews for radio and podcasts. He is open to public speaking and advising engagements.