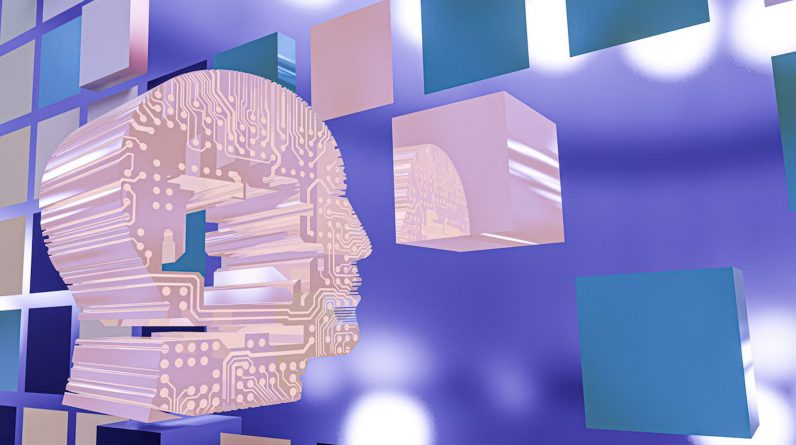
One of the most recognizable trends of the early years of the 21st century has been the spread and application of AI (Artificial Intelligence) within many professional areas. The data analysis, pattern recognition, and decision-making functionalities in AI have produced remarkable efficiencies and ideas. However, ethical concerns have risen to dominate as these artificial intelligence systems including machine learning algorithms penetrate our daily lives. This signifies a significant year in our journey towards addressing these issues that would ensure that equity is promoted in AI systems and prevent them from perpetuating or worsening societal disparities by 2024.
Understanding bias in AI
The term bias in AI refers to systematic discrimination or advantage afforded to some individuals or groups and not others. This can be expressed in different ways like racial, gender, socio-economic status, and age biases among others. Such prejudices are usually derived from the data used for training machine learning models. If the training data is non-representative of a varied population on earth or it contains historical biases, then such AI systems are likely to capture those partialities resulting in unfair and disproportionate outputs. How this AI biasness algorithms and Machine learning working practically that you can understanding from multiple AI tutorial or Data Science Course available online.
The ethical imperative for fair AI
The reason to create artificial intelligence systems that are fair is justice. In critical fields such as health care, law enforcement, employment and financial services, these technologies play a bigger role. The effects of biased decisions can be life-changing for individuals. Guaranteeing fairness in AI has more than one aim: it’s about making systems that mirror our shared values and promote a more equitable way of life.
Strategies for tackling bias in AI
Diverse and representative data
One of the leading tactics aimed at fighting bias in artificial intelligence is to ensure that the data sets used for training the machine learning models are diverse and representative of the global population. This means demographic diversity, but also different experiences, perspectives and environments. Again, efforts aiming at auditing and cleansing datasets from historical biases, are important too.
Transparent and explainable AI
Transparency is about an AI system that can be understood and investigated by humans in the way it was created. This is closely related to the idea of explainable AI, where models are built to provide reasons for their decisions in a language understandable to human beings. Hence, stakeholders can grasp how and why particular choices were made thereby identifying and mitigating biases.
Regular auditing and monitoring
It is important to continuously check the bias of AI systems. Such checks include both pre-deployment and after-deployment processes that ensure continued fairness even as they encounter new data or scenarios.
Ethical AI frameworks and governance
Ensuring AI fairness requires developing, and implementing ethicalness of AI frameworks as well as governance arrangements at the societal and organizational levels. These AI framework is little bit very complex task to understanding. Multiple artificial intelligence course helps to understand these complex structure of fairness pattern in AI. Establishing guidelines, principles or standards for developing and using ethical artificial intelligence alongside mechanisms that can hold accountable those who have suffered from bad decisions of AI are fundamental in this regard.
Cross-disciplinary collaboration
Tackling bias in AI is a complex challenge that requires collaboration across disciplines, including computer science, social sciences, ethics, and law. Such collaboration can bring diverse perspectives and expertise to the forefront, facilitating more holistic and effective solutions.
Embedding ethical AI principles in development processes
- Design and User Experience (UX):Designers and user experience professionals are essential in creating an inclusive and accessible design for all users. In particular, by incorporating Ethical AI practices, developers can avoid unconsciously marginalizing specific subsets of users, particularly when using AI-based personalization algorithms.
- Data Handling and Privacy:Full Stack Developers manage the movement of data across the entire life cycle of an application, starting from data gathering (front-end) to storage and processing (back-end). Ethical concerns about data privacy, consent and security are critical particularly in AI-based applications that require lots of training data. More about these front end as well as backend system of Full Stack can be get from online full stack developer course and tutorials.
- Bias Mitigation at Every Layer:At each layer of the application, from the design of the database schema to data processing algorithms and information presentation through a user interface, there can be biases. Full stack developers have a unique position as they can look at all parts of it and address bias at any place in the stack ensuring that AI components of the application are impartial and just.
The future of ethical AI
A dynamic and constantly changing field is the ethical AI adventure in such a way that it remains very important even as we go forward. Technology and methodology advancements combined with an increasing understanding among the general population about ethical considerations are facilitating the movement to more equitable AI systems. The concern is on making sure that harm has ceased happening and also utilizing AI potentiality towards societal benefit and human well-being.
In conclusion, bias in AI and fairness issues rank top among various pressing ethical challenges facing the AI community now. In addition, diversity and ethics, continuous vigilance, transparency, accountability, and oversight of research operations involved in its development will foster not only innovative but also just outcomes for all people from different backgrounds.
Featured image credit: Steve Johnson/Unsplash