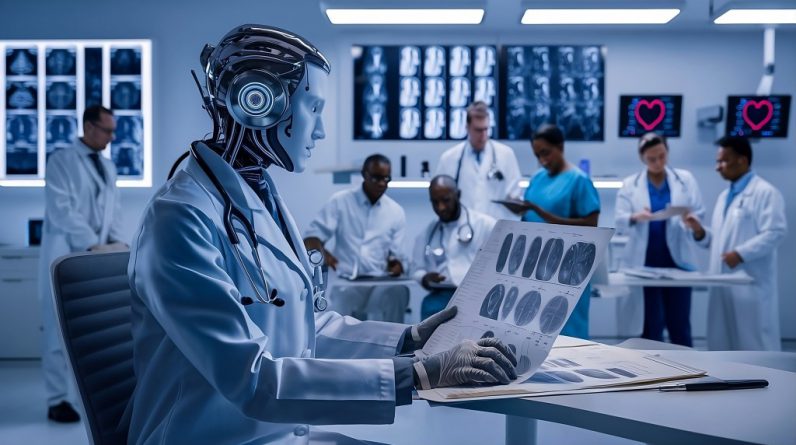
We want to hear from you! Take our quick AI survey and share your insights on the current state of AI, how you’re implementing it, and what you expect to see in the future. Learn More
In just a short period of time, AI has demonstrated viable capabilities in healthcare: Large language models (LLMs) can offer tumor diagnoses, provide sleep and fitness advice, scan medical images and analyze MRIs, X-rays and tissue samples.
For all its opportunities, though, there are significant — and valid — concerns around output accuracy, transparency, integration, data privacy, ethics, bias and regulatory compliance, among others.
“The integration of AI into healthcare is not just an evolution but a revolution that holds the promise of significantly enhancing patient care, operational efficiency and medical research,” Timothy Bates, clinical professor of cybersecurity in the College of Innovation and Technology at the University of Michigan-Flint, told VentureBeat.
But, he emphasized, “realizing this potential requires addressing substantial challenges.”
Countdown to VB Transform 2024
Join enterprise leaders in San Francisco from July 9 to 11 for our flagship AI event. Connect with peers, explore the opportunities and challenges of Generative AI, and learn how to integrate AI applications into your industry. Register Now
AI throughout the medical workflow
To start, AI can take over time-consuming, repetitive tasks such as summarizing appointments (which it has already been shown to do better than humans). The technology can also streamline administrative processes like scheduling, billing and patient management. Further, AI-driven predictive analytics can help with resource allocation.
“Despite legitimate concerns about generative AI, in five years, healthcare providers will wonder how they ever got along without it, especially for things like transcribing clinical notes and decision support,” said Dr. Colin Banas, chief medical officer at medication management company DrFirst.
Going beyond that, AI can improve diagnostics because it can analyze vast amounts of data quickly and accurately, said Bates. For instance, AI algorithms can analyze medical images to detect conditions such as cancer, heart disease or neurological disorders earlier and more accurately than traditional methods.
One example includes AIdoc, which is helping transform radiology by detecting anomalies in medical imaging with high accuracy.
“AI has algorithms that can detect cancer in imaging much sooner than what doctors can do now, providing for earlier, less invasive treatment and a higher chance of survival,” said Baruch Labunski, CEO at SEO company Rank Secure.
AI can also support more personalized medicine through analysis of data such as genetics, lifestyle, medications, conditions and past procedures, Bates pointed out. And, models can remotely monitor wearables that track vital signs and health metrics in real time. “This is particularly beneficial for managing chronic diseases and providing care in underserved areas.”
Similarly, predictive analytics can help foresee patient deterioration, sepsis and other critical conditions, thus allowing for timely interventions. For instance, hospitals are using AI to predict patient readmission risks and accordingly tailor post-discharge care plans, Bates pointed out.
From an infrastructure standpoint, predictive analytics can also optimize operations of complex hospital environments, said Bjorn Andersson, senior director for global digital innovation marketing and strategy at Hitachi Vantara. Models could analyze live input from sensors or even external data around weather forecasting to help institutions preposition resources in the case of an impending heatwave or other weather-related event.
“This combination of physical and cyber is becoming more important as things like weather events become more unpredictable and severe,” said Andersson.
Supporting drug discovery, clinical trials
AI also has immense potential in research labs, notably around drug discovery and development, experts note.
For example, researchers at the National Institutes of Health (NIH) National Cancer Institute have built a machine learning (ML) model that generates more accurate predictions for immune checkpoint inhibitors (drugs that treat cancer). Another example is Google’s DeepMind, which has made breakthroughs in protein folding predictions to aid drug discovery.
“One of the best aspects of using AI in healthcare is in analyzing proposed drug treatments, side effects and how they will likely interact,” said Labunski.
Meanwhile, in clinical trials, gen AI can pre-screen patients by comparing data from questionnaires against trial requirements, thus increasing the number of qualified participants, said Cara Brant, CEO at patient recruitment platform Clinical Trial Media.
“This helps reduce cost and time, which both impact how quickly potentially life-changing drugs get to market,” she said.
Concerns around data privacy, integration, transparency
On the flip side, two of the most critical concerns around AI use in healthcare settings are data privacy and security.
Bates pointed to the “highly sensitive” nature of healthcare data, which will require “robust data protection measures” with AI use. “Ensuring patient confidentiality and securing data against breaches is paramount,” he said.
Regulatory compliance is another biggie, and healthcare institutions must balance data accessibility with stringent privacy regulations such as HIPAA. “Ensuring compliance with existing regulations while also adapting to new ones can be a daunting task for healthcare providers and technology developers alike,” said Bates.
Further, medical institutions can struggle to integrate AI with existing legacy systems, which can lead to interoperability issues. This in turn requires investment in upgrades and staff training. Also when it comes to staff (patients, too) there can be skepticism around the reliability and efficacy of AI, Bates pointed out.
“AI doesn’t share how it arrives at conclusions, and the lack of transparency in that process could pose problems in treatments and even providing care,” said Labunski.
Muddu Sudhakar, co-founder and CEO at enterprise search company Aisera, agreed that “models can be complex black boxes with little transparency.” Also, “AI is not perfect. It can make mistakes.”
Thus, going forward, Bates noted, it will be important to build trust through transparent processes, rigorous testing and demonstration of tangible benefits.
Ensuring humans continue helping humans
As the industry increasingly adopts AI and automation, there is a “significant concern” that there will be a shift away from people interactions.
“Healthcare is deeply personal and intimate, which is one of AI’s largest limitations,” she said.
Patients want to work with providers they trust, and the average person may not fully understand what their diagnosis or treatment plan means. They must work hand in hand with another human to understand all the different nuances. “The healthcare industry is challenged with toeing the line between using AI to optimize processes and reserving some areas of care solely for humans,” she said.
AI, at least at this point, is a provider from which to “get a second opinion” on scans and test results, agreed HP Newquist, executive director at The Relayer Group. AI can analyze a “data warehouse” of each patient, and run that against the most up-to-date medical papers and state-of-the-art practices. Data can then be summarized quickly and presented to a doctor along with recommendations.
“The key word here is ‘recommendation’—AI is not even close to a point where it should be making decisions without human supervision,” said Newquist.
He noted that no one doctor can keep up with the flow of new information in their chosen field. However, an LLM fed with daily reports from peer-reviewed journals could quickly recommend the newest perspectives on specific diseases, operations and patient care.
“For more clinical tasks, ‘augmented intelligence’ is the sweet spot for healthcare,” Banas agreed.
Be sure to check out VentureBeat’s upcoming VB Transform, to take place July 9-11 in San Francisco. We will welcome experts from Kaiser Permanente, Sutter Health, Google, Stanford Hospital, Healthvana and more, who will address key trends in the healthcare sector.
VB Daily
Stay in the know! Get the latest news in your inbox daily
Thanks for subscribing. Check out more VB newsletters here.
An error occured.