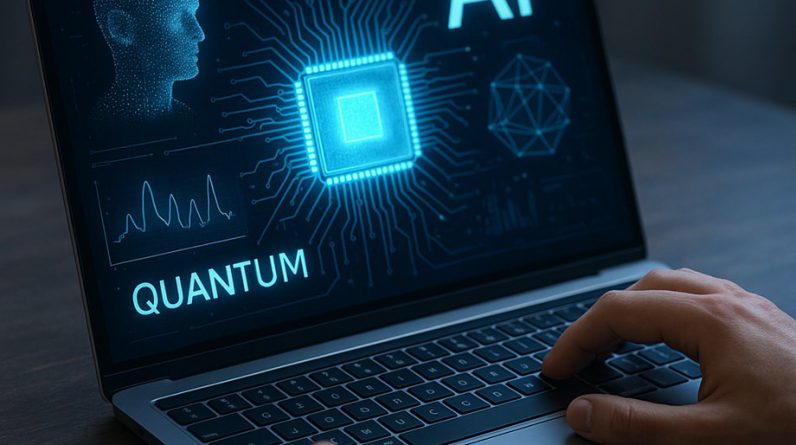
The rapid convergence of quantum computing and artificial intelligence (AI) is driving a paradigm shift in how science and engineering are conducted. Once limited to theoretical promise, these technologies are now merging into practical frameworks that reshape research methodology and industrial design. At the heart of this transformation is the concept of “Quantum Computer-Aided Engineering” (Quantum CAE), a novel approach integrating quantum algorithms into automated engineering workflows.
In a newly published perspective titled “Quantum Computing and AI: Perspectives on Advanced Automation in Science and Engineering” on arXiv, Tadashi Kadowaki explores how quantum computing and AI are accelerating the transition from human-led experimentation to fully automated scientific inquiry. The paper outlines a scalable framework for advanced automation, benchmarked against traditional CAE, and delves into real-world applications already under development.
How is scientific automation evolving with AI and quantum computing?
Scientific research, traditionally reliant on manual hypothesis generation, experimentation, and analysis, is undergoing a radical transformation. With increasing demand for faster and more cost-efficient discovery, organizations are prioritizing the automation of research processes. This transformation draws parallels with how Computer-Aided Engineering revolutionized product design in the past decades.
Scientific automation is now stratified into levels, similar to self-driving vehicle autonomy, ranging from Level 0 (fully manual) to Level 5 (fully autonomous). Current capabilities correspond to Level 3: AI systems can autonomously generate hypotheses, design simulations, and analyze results, though under well-defined conditions with human oversight. Fields like materials science and molecular biology are already utilizing AI-driven pipelines that closely mirror this level of automation.
By leveraging AI’s ability to simulate experiments computationally and quantum computing’s potential for accelerating algorithmic processing, researchers are now automating every step of the discovery cycle. These include topic selection, code development, simulation execution, data analysis, and even the drafting of manuscripts. Crucially, AI agents, termed “digital scientists”, are now capable of managing complex iterative workflows and decision-making processes within defined research domains.
What is Quantum CAE and why does it matter?
Quantum CAE is an emerging framework that applies quantum computing principles to standard CAE practices such as simulation, optimization, and machine learning. This approach aims to overcome the limitations of classical computational methods, especially in solving combinatorial optimization problems – common in real-world engineering scenarios involving discrete variables.
Initial implementations focus on substituting one step of the CAE process, such as optimization, with quantum-enhanced techniques. For instance, quantum annealing has shown promising results in identifying optimal design configurations from limited data. Two methods highlighted in the study, Bayesian Optimization of Combinatorial Structures (BOCS) and Factorization Machine Quantum Annealing (FMQA), demonstrate the practical utility of quantum technologies. These approaches deliver feasible solutions more efficiently than traditional algorithms, with minimal iterations.
In the automotive domain, researchers used these quantum-driven techniques to optimize mounting positions for electronic control boards, balancing vibration resistance, cost, and manufacturability. Similarly, for printed circuit board design in high-frequency electronics, quantum-enhanced combinatorial optimization effectively generated layouts that matched or outperformed those developed via traditional topology optimization methods.
These advances are not limited to mechanical or electrical engineering. Quantum CAE has potential applications in pharmaceuticals, optics, magnetic materials, and chemical design – any field where simulations and optimization are computationally intensive and design variables are discrete.
What’s next for AI-Quantum integration in research?
While current capabilities correspond to Level 3 automation, the study projects a future driven by Level 4 and Level 5 systems, where AI agents autonomously carry out entire research agendas – from problem formulation and hypothesis testing to full-scale experimentation and publication. Achieving this vision will require breakthroughs not only in AI capability but in inter-agent communication, collaborative logic, and quantum algorithm design.
One promising development is the application of generative AI for quantum circuit design. The study highlights recent efforts where Transformer-based models automatically generate quantum circuits tailored for specific tasks, such as molecular energy calculations or optimization problem solving. These circuits are trained using graph neural networks to interpret problem structures and then generate appropriate quantum logic – a task previously reserved for domain experts.
The integration of quantum computing with such AI agents creates feedback-rich loops where quantum acceleration enhances AI performance, and AI refines quantum algorithm efficiency. This reciprocal development is expected to dramatically reduce the time and cost of complex research and engineering tasks.
Researchers also emphasize the need for hybrid computing infrastructures that combine classical and quantum capabilities, supported by large-scale supercomputing facilities. Such platforms would allow digital scientists, whether general-purpose or highly specialized, to operate within integrated research ecosystems.