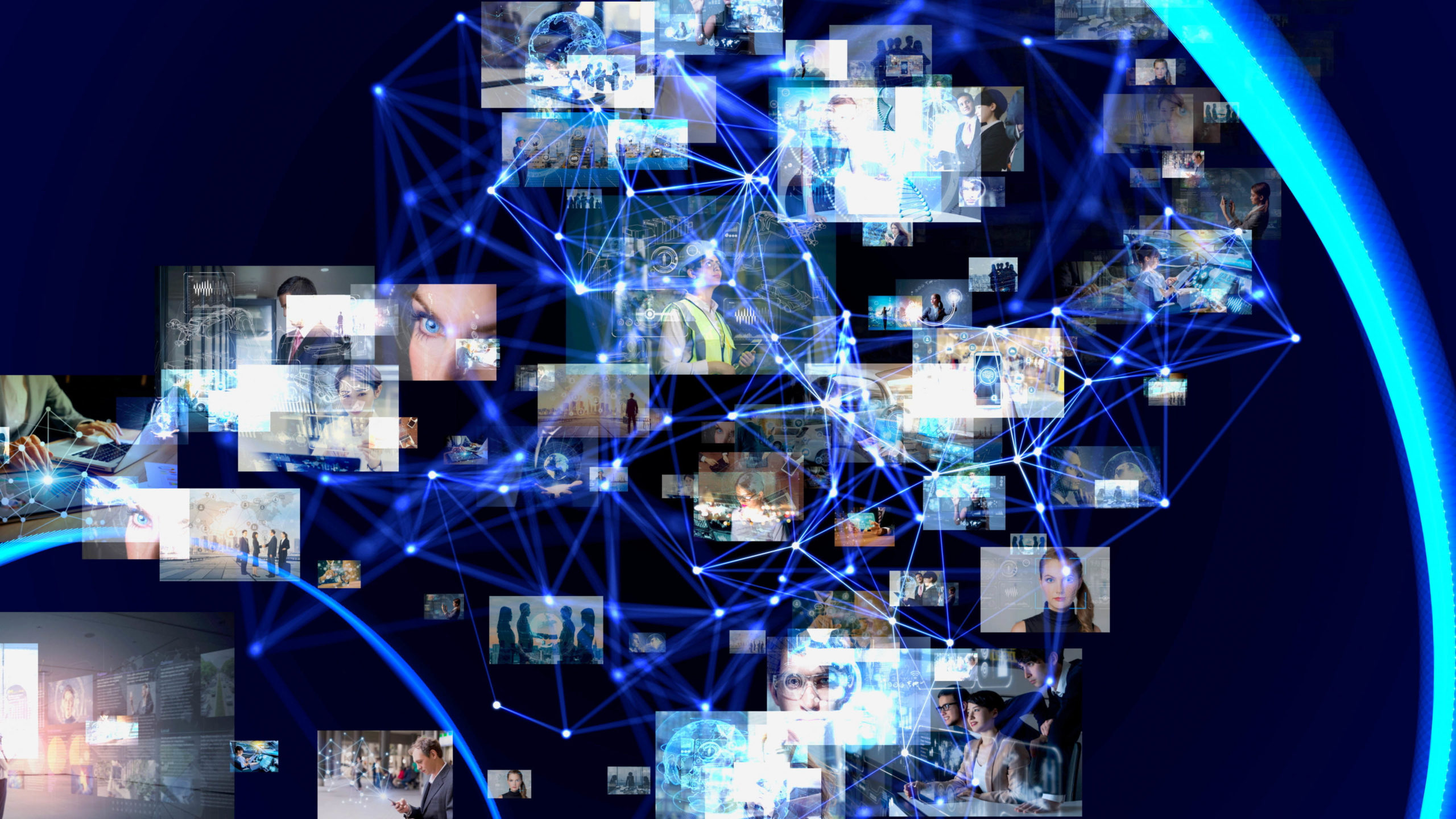
In what ways is TF1 already using AI in its processes and workflows?
Olivier Penin: TF1 has been using AI for many years via our content recommendation algorithm, automatic thumbnail identification on our TF1+ streaming platform. The former was developed internally by a dedicated AI and data scientist team, and the latter comes from a technology partnership that is also helping us improve the advertising experience through identifying ad break moments in our content with AI.
The release of ChatGPT in November 2022 opened up a new range of opportunities in terms of AI, especially GenAI (generative artificial intelligence). TF1 has been working on identifying where GenAI can have the greatest impact and conducting proof-of-concepts (PoCs) with the aim of industrialising the AI use cases that have proven to be successful.
Olivier Penin, director of innovation, TF1
There are several AI areas that we want to concentrate on to unlock maximum value, including improving our audience user experience (i.e. making it easier for our users to navigate on TF1+ and find the content they want), enhancing our production and content management processes and gaining efficiency (i.e. bringing automation to daily repetitive tasks within TF1 and notably the media supply chain).
To address the first AI objective of improving the audience experience, we’ve recently introduced the Synchro service on our TF1+ video streaming platform. Synchro allows users to easily find the content they want to watch when several people are in front of the TV screen, using their TF1+ profile characteristics.
For the second objective of enhancing production and content management processes, we released the Top Chrono service on TF1+ for the Rugby World Cup in 2023. This allows users to create a summary of rugby games and choose the duration (i.e. 5, 10 or 15 minutes). The summary generation relies on identifying highlights thanks to AI. We plan to make this service available again for football matches during the Euro 2024 competition.
To meet the third objective of boosting efficiency, alongside ad break moments identification (described above), we are also using AI to gain efficiency in hiding brand logos in content due to compliance constraints, and in SEO for our written news content and computer coding assistance. Finally, we are working on developing an internal chatbot similar to the style of ChatGPT that offers security compliance (i.e. no data is shared with OpenAI while using the chatbot).
How do Moments Lab’s next-gen AI indexing models align with TF1’s group ambitions and goals?
OP: Moments Lab’s AI models provide TF1 with an efficient way to index content, generate tags, and create content summaries. This opens up opportunities to address important use cases for us, including the ability to:
- Improve searchability within our archives, especially for news content, allowing our journalists to increase the efficiency of news production.
- Investigate new content formats and create trailers more efficiently by having the ability to index and identify highlights in non-sport content.
- Write text summaries for all of our content with greater efficiency (for TF1+ as well as for the EPG TV guide).
From a global standpoint, properly indexing our content is key to generating data around our content. It can then be converted for specific use cases to address our AI objectives. Our partnership with Moments Lab allows us to rapidly move forward in this field.
What will participation in the research project entail – how will TF1 be able to contribute?
OP: TF1’s participation relies on the use cases described above: improved media library/archive searchability, new content formats and more efficient creation of text summaries for media assets. We contribute to the research project by providing Moments Lab with problems we encounter in our workflows, and the data needed to advance research into these problems after being translated by Moments Lab into scientific/ technology questions. Moments Lab then kicks off the research and leverages AI to invent the right technology to produce the results we are looking for. We provide regular feedback on iterations to reach a successful PoC.
How many members of your team will be involved?
OP: The TF1 Innovation department is a small team of about 10 people, three of which are involved with these projects, plus many people from other TF1 teams. Globally, there are about 15 people involved in Moments Lab AI use cases.
What does TF1 hope to achieve by joining the Moments Lab research project?
OP: As a partner of Moments Lab for several years, TF1 is excited to make progress on content indexing and video understanding, as these are core to several of our high-value AI use cases that aim to offer our audiences a better user experience and improve the efficiency of our internal processes.
The programme places emphasis on building low energy consumption AI indexing models to learn fair representations of a diverse and multicultural world – how do the solutions avoid bias?
Yannis Tevissen: The first step to avoid biases is to be able to detect them. Some of our current work aims at detecting biases within AI models already used for the media industry. We know that most popular models, developed in North America, suffer from strong cultural biases, making their use challenging when it comes to covering news in the Middle East, for example.
Yannis Tevissen, head of science, Moments Lab
Once biases are detected, they can be avoided by fine-tuning models on well curated datasets, by using targeted post-processing rules, or with custom and contextual prompt engineering. Another option is to use a companion AI expert system to check, correct or even improve specific parts of the main AI analysis. This method was proven to be successful at including people’s names in automated image descriptions in a scientific paper published by Moments Lab. All of these solutions will be carefully crafted and benchmarked in the context of this program.
How does the programme ensure robust data security?
YT: The data used for the programme will be stored on the already existing Moments Lab platform, and is encrypted throughout its lifecycle, making it unavailable to the outside world (or any third-party tool — the LLMs we use are deployed internally to prevent unauthorised use). Apart from the case of very specific training, videos are always watermarked, preventing the unauthorised use of original content. Finally, all other actors involved, such as academic labs, work in a private and closed computing environment.
When do you expect to publish your first findings?
YT: First findings have already been published in the form of a research paper authored by Moments Lab about multimodal chaptering for newscast videos. This method allows the use of multiple modalities (i.e., raw audio, image, transcript, speaker names) to automatically create chapters fitting TF1’s editorial sequencing. This state-of-the-art method was made possible thanks to the combination of Moments Lab multimodal AI expertise and TF1’s high-quality data.
Further research will aim at developing AI-assisted video creation through natural language assistants that could help TF1 achieve faster video search and editing while preserving the human creative journey.
What future uses can you see for AI in the media?
YT: As AI vision-language models are becoming more and more efficient, many new applications of such systems are envisioned for the media sector. AI can especially help accelerate fastidious tasks such as suggesting or retrieving moments within a large video archive based on visual and speech highlights. AI can also be used to suggest new and unexplored editorial angles or original video edits, always with the goal of fostering the creativity of journalists and video editors, enabling them to spend time on what matters, be it a creative or reporting process.
OP: AI will likely have the most impact at different levels of the media value chain, from production to distribution. On the production side, animated content and VFX might derive value from GenAI in the coming months, not necessarily through 100% AI-generated content at first, but by implementing AI throughout production processes to make them more efficient. In the case of VFX, AI could help generate high-end VFX faster and in a more accessible way than today. On the distribution side, content searchability and personalised user experiences might gain attention (e.g., more efficient content search bars, personalised trailers depending on the user profile, customised advertising, etc.).