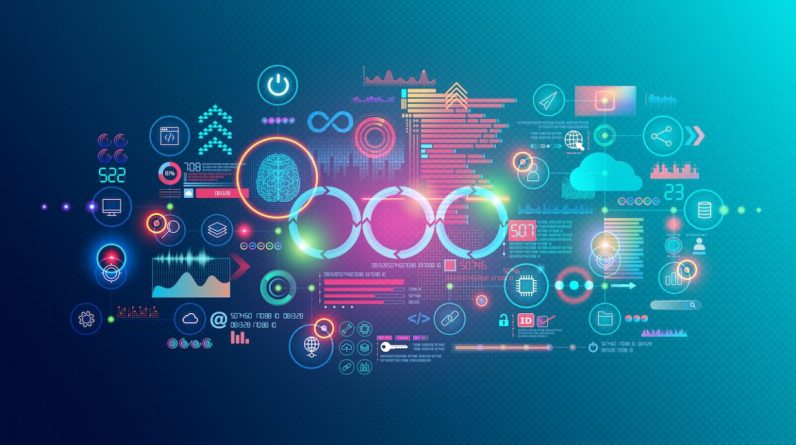
Presented by BMC
Today’s north star is the autonomous digital enterprise, characterized by three traits: business agility, customer centricity and the ability to drive decisions with actionable insights – three traits all highly dependent on (good) data. As a result, the value of data itself is greater than ever. Conversely, sifting through the vast mounds of increasingly complex data to efficiently and effectively generate value has never been a more crucial, or more challenging, task.
“Many organizations see the value of data but continue to struggle with data management, which gives a major competitive advantage to those organizations that can get it right — and poses an existential threat to those that haven’t yet,” says Ram Chakravarti, CTO of BMC Software. “That’s been a big pain point, what I call the last-mile delivery challenge for organizations. Becoming a mature data organization is key.”
In fact, a BMC global IT and business practices survey backs that up: It found that organizations with a high degree of data maturity see better outcomes in strategic decision-making, customer satisfaction, cost reduction and product development.
The obstacles to data maturity
Traditional data challenges are increasing dramatically in the age of AI. The costs of mining, storing and analyzing data as well as the expertise to manage and apply data are resulting in high investment needs and talent scarcity. New challenges are also emerging. For one, there’s the proliferation of new data sources across every aspect of business from devices, applications and people. Silos of data management and use, without top-down guidance, mean cultural shifts that can streamline data operations that don’t gain traction. And operationalizing at scale, at the speed and level of sophistication that stakeholders are increasingly expecting, is an ongoing obstacle. Automation, and emerging technologies like AI, can be incredibly useful but are ineffective at scale when data practices are not aligned.
“Many organizations have struggled to successfully operationalize their data management and analytics initiatives beyond a couple of use cases,” Chakravarti says. “It goes beyond technology enablers like automation. You need to re-orient your operating model and change your processes because traditional data management and processes don’t work in the age of AI – it requires DataOps.”
The DataOps difference
Short for data operations, DataOps is a practice that encompasses DevOps best practices, automation and intelligence, to democratize data and unlock business value. It incorporates the business side of the organization — the analysts, business data owners and stewards as well as the data engineers, data scientists and data translators, all working with security and risk management personnel to safely accelerate the collection and implementation of data-driven business insights.
“The collaboration needs to be absolutely spot on across this continuum of stakeholders, otherwise you’re severely hampered at the outset,” explains Chakravarti. “It’s an agile process, where data is considered a shared asset, so data models must follow the end-to-end design-thinking approach across teams, and support high value-use cases.”
That includes revenue creation opportunities – for instance, insight into customer behavior that a competitor doesn’t have in order to increase loyalty and spend. Also at the top of the list are productivity and efficiency gains that include employee self-service and knowledge management, as well as risk management and mitigation. Using data and intelligence to pivot on existing strategy is a use case that is more qualitative and difficult to achieve, but as data intelligence becomes more sophisticated, it’s gaining traction, and becoming a competitive advantage.
The technical foundation of a DataOps strategy
Automation is a critical enabler of DataOps, creating greater efficiencies in the orchestration of complex data pipelines that take relevant information from a whole host of traditional and emerging sources through all the steps required (ingestion, integration, quality, testing, deployment, monitoring, as well as metadata management and governance) before it can be turned into actionable insights. On top of that, automation brings in observability to deliver insight into the health and performance of data at any point in time in these pipelines. This oversight is profoundly important to the value of the end results.
Organizations also need to focus on maintaining high-data quality to ensure the effectiveness of AI and analytics projects. This includes addressing issues like data accuracy, consistency and completeness, and implementing tools and processes for data assurance, especially in analytics pipelines. That said, a pragmatic approach to improving data quality is usually necessary, as diving head-first into an initiative can require significant investment. More than technology, successful DataOps requires major process changes and a cultural shift that can sometimes prove to be seismic.
Starting the DataOps journey
When launching a DataOps strategy, you can’t set out to solve all the challenges of enterprise data management immediately. The DataOps approach calls for starting small, going for higher-value, easier wins and applying data quality best practices to those initial use cases. And when you’re ready to really start learning and scaling, you want a few things in place.
First, you need executive support and buy-in. Any process change will need cross-functional collaboration.
Second, you need the right organizational constructs in place, which means defining a solid operational and stewardship structure. In other words data should be owned and stewarded across the organization.
Then comes figuring out what you want to tackle. Understanding and knowing the outcome you want gives you the ability to identify the highest-value use case and invest appropriately. Data projects are most successful when the outcome is clearly aligned to, and positively impacts, a tangible business benefit (eg: customer success metric of X% increase in retention or efficiency impact on employee productivity through self-service).
You also need to be able to iterate and repeat, without compromise on standards for data quality, at every scale — taking small, systematic steps while baselining and benchmarking.
“Start small, show value, and generate it quickly, and ask the tough question: so what?” Chakravarti says. “Learn. Build. Scale. Define continuously. Engender new practices. Introduce new things systematically, in small bites, and you’ll get to a good place.”
Learn more about unlocking the value of your data at scale, here.
Sponsored articles are content produced by a company that is either paying for the post or has a business relationship with VentureBeat, and they’re always clearly marked. For more information, contact sales@venturebeat.com.