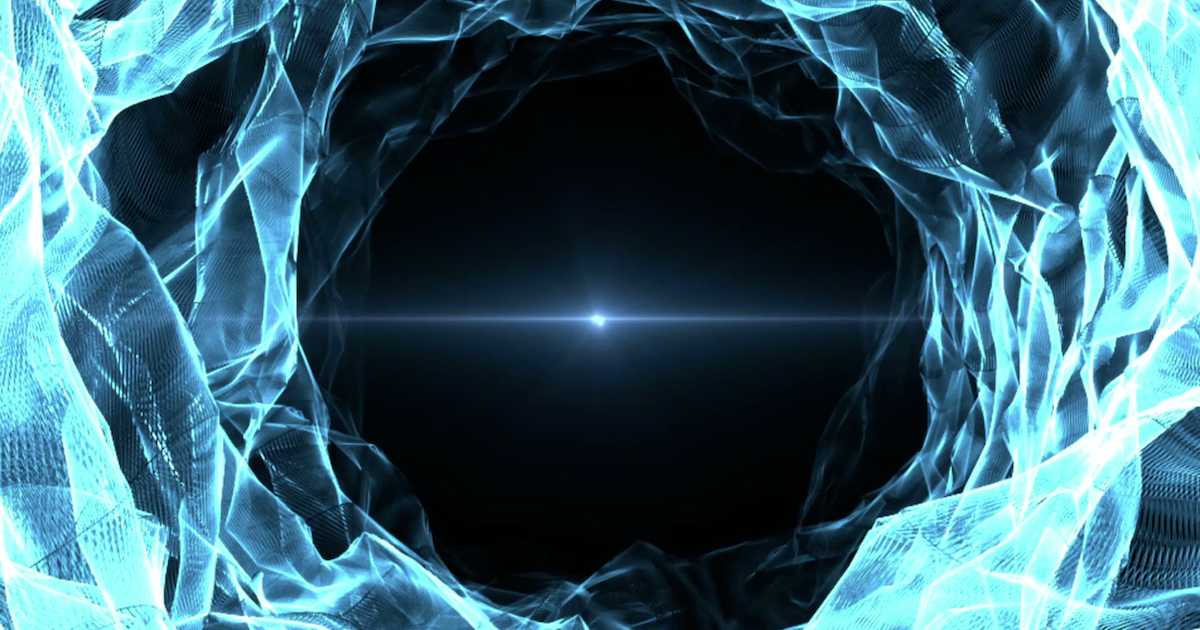
At QCon London, Rachael Greaves, chief executive officer at Castle Systems, presented both the obligations and benefits of data minimisation as a mechanism to decrease the impact of data breaches. AI autoclassification and automatic decision-making tools help with the ever-increasing data volumes as long as ethical principles are considered, allowing decisions to be challenged.
Greaves started her presentation by pointing out that cybersecurity focuses mainly on reducing the likelihood of a breach through training, firewalls, and encryption. But, risk is a combination of likelihood and impact, i.e. “There could be a small likelihood of penetration but a critical impact”.
Data minimisation is a mechanism to lower the impact of data breaches. She stated that creating an impenetrable system is impossible: “There will always be the zero-day, the trusted inside man or the misconfiguration”. It is a security and privacy principle that requires organisations to limit the amount of information they hold, knowing that they might be breached or the data spilt into the public domain at any moment.
Besides the legal obligations, Greaves pointed towards the benefits of implementing data minimisation:
- Deterrence: Data minimisation reduces the potential harm that can be inflicted when data is spilt but also discourages further attempts to break into the system (minimising the amount of data that ill-willing actors can monetise will discourage further attempts).
- Response and Recovery: If you fully understand your data, this is a secondary benefit of data minimisation. Knowing this ahead of an incident will allow you to know “who’s in the spill” (which customer was affected). In case of a breach, you can swiftly alert the affected parties, minimising the impact.
- Insurability and Risk Transfer: Even if the evaluation process is opaque, the evaluation of cyber insurers has broad parts related to sensitive data. It was also found that organisations containing large amounts of sensitive information tended to have higher insurance costs.
- Organisational Effectiveness: You need to understand all your information holdings, i.e. what has risk and what has value, and importantly what rules apply to that information (retention rules, secrecy rules, regulatory obligations).
Even if many of the actions related to data minimisation can be enforced through governance, Greaves considered this to be an effort for the whole organisation, an effort in which developers play a key role, especially with data inventory (identify sensible or high-value data). She stressed that if done properly:
Data Minimisation enables Organisational Maximisation
Data minimisation is not an additional phase in your project, but it’s an ongoing effort throughout the whole data lifecycle from creation or capture to eventual disposal. Three key elements of data minimisation stand out:
- Minimising collection: Don’t collect extraneous personal details, don’t collect the same data twice as part of two different services, don’t keep duplicates, excessive backups or offline copies, and collect only what’s needed.
- Minimising access: Minimise the number of people with access, their privileges and the duration of their access (“seeing who is doing what to data and being alerted to actions on sensitive and high-value data helps identify privilege creep..”).
- Data End-Of-Life Management: Data disposable is more than just hard drive degaussing. Much can be accomplished through policies and governance around records management and retention policies.
Greaves underlined again that even if many of the results come from processes and governance, it is of the utmost importance that developers buy in and support the “data-minimisation philosophy” as data privacy and governance are shifting left.
Given the complexity and vast amounts of data, technology can shed light on “what’s valuable and what’s sensitive” in a timely and precise manner (Minimising risk and maximising outcomes). Artificial intelligence is very suitable for such tasks, especially autoclassification of data and Automated Decision Making (ADM). Systems need to be able to gather and flag sensitive data across multiple systems without affecting the source systems, but they should still keep humans as the ultimate decision-makers:
With something as risky as data governance it is important not to remove the people completely from the loop.
To avoid AI bias, hallucinations, or the risk of AI systems being used maliciously, some software-assisted obligations (AI-enabled or not) need to be explainable and transparent. This way, they can be challenged to avoid harm being inflicted on the most vulnerable communities.
Greaves concluded her example-rich presentation (covering the OPM data breach, Australian University data breach, Windrush scandal, etc.,) with checklists that incorporate the best practices presented beforehand. According to her, privacy laws are skewed towards destroying data, records laws are skewed towards preserving it, and national security laws tilt the balance back towards the disposal of sensitive data. So, regardless of how hard it seems, systems need to balance the tension between data risk and data value through system design.