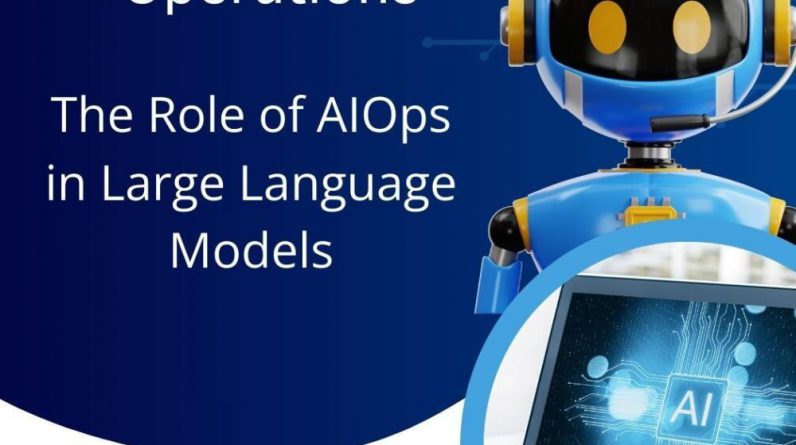
In this digital era, the rapid advancements in Artificial Intelligence (AI) have revolutionized the way large language models (LLMs) are developed and deployed. Artificial Intelligence for IT Operations (AIOps) has emerged as a groundbreaking solution, simplifying the complexities of managing these powerful systems. Sekhar Chittala’s analysis highlights how AIOps transforms LLM operations, offering automation, efficiency, and ethical governance. This article delves into innovative automation strategies, addresses operational challenges, and explores future trends shaping scalable and responsible AI management in enterprise landscapes.
Automating Complexity: Strategies for Deploying LLMs
Deploying large language models (LLMs) requires careful planning and strategic resource allocation due to their size and complexity. Automation plays a pivotal role in effective management, offering innovative solutions that simplify workflows and improve operational efficiency.
- Data Preparation Pipelines
Automated pipelines play a pivotal role in maintaining high-quality and consistent data by seamlessly integrating advanced techniques such as validation, anomaly detection, and data augmentation. These processes not only enhance efficiency and accuracy but also minimize human errors, providing a reliable foundation for building robust and scalable AI systems. - Optimizing Model Training
Automation accelerates training through techniques like neural architecture search and distributed computing. By refining hyperparameter tuning and gradient accumulation, training times and computational costs are significantly reduced. - Continuous Deployment Practices
Integrating CI/CD pipelines specifically tailored for LLMs automates testing and versioning while minimizing deployment risks. This approach ensures reproducibility and seamless scalability across organizational needs.
Tackling Challenges with AIOps
LLM management presents formidable challenges that AIOps effectively mitigates. By leveraging predictive analytics and dynamic scaling, organizations can optimize their AI infrastructure:
- Resource Allocation
Intelligent scheduling algorithms ensure efficient GPU/TPU usage, dynamically adjusting to workload demands. This minimizes waste and optimizes cost-effectiveness. - Latency Reduction
AIOps-driven profiling identifies bottlenecks and applies solutions like model quantization and load balancing. These measures enhance real-time performance for mission-critical applications. - Scalability with Precision
Dynamic scaling adjusts system resources in response to real-time demands. Techniques like model sharding and distributed inference empower organizations to handle diverse workloads without compromising efficiency.
Enhancing AI Governance: AIOps Meets MLOps
The integration of AIOps with Machine Learning Operations (MLOps) creates a holistic framework for managing LLMs throughout their lifecycle. This synergy ensures:
- Version Control and Governance
Automated tracking of model iterations enhances transparency and accountability, streamlining updates and audits. - Continuous Validation
Robust frameworks continuously test models against performance and integration benchmarks, ensuring reliability in production environments. - Feedback Loops
Real-time monitoring of resource usage and user interactions generates actionable insights, driving iterative improvements in model performance.
Building Ethical AI Systems with AIOps
As AI systems grow more autonomous, the need for ethical oversight intensifies. AIOps integrates ethical considerations into its design:
- Bias Mitigation
Automated tools analyze training data and outputs to identify and address biases, promoting fair and inclusive AI solutions. - Transparency Mechanisms
Techniques like SHAP and LIME provide interpretable insights into model decisions, fostering trust and accountability. - Governance Frameworks
By embedding human oversight and escalation protocols, AIOps ensures that ethical principles guide AI deployment and operations.
The Future of AIOps in AI Management
The evolution of AIOps is set to bring transformative advancements that will redefine AI management across industries:
- Sophisticated Automation
will drive innovation with self-healing systems and reinforcement learning, enabling proactive resource optimization and bolstering system resilience against disruptions. - Scalable Solutions
will leverage multi-cloud and edge deployments, empowering organizations to efficiently manage vast LLM ecosystems while cutting operational costs and enhancing performance - Emerging Technologies
such as quantum computing, 5G, and IoT will unlock groundbreaking capabilities, facilitating real-time decision-making and seamless distributed AI management, further advancing the potential of AIOps in creating dynamic and efficient AI ecosystems.
In conclusion, Sekhar Chittala’s work underscores AIOps as a transformative approach to addressing the intricate challenges of managing large-scale AI systems. By seamlessly integrating automation, ethical principles, and innovative strategies, AIOps empowers organizations to develop scalable and responsible AI solutions. As these technologies advance, they will remain instrumental in revolutionizing AI operations, driving efficiency, and ensuring alignment with societal needs and ethical standards. AIOps not only simplifies complexity but also paves the way for a future where AI serves humanity responsibly and effectively.