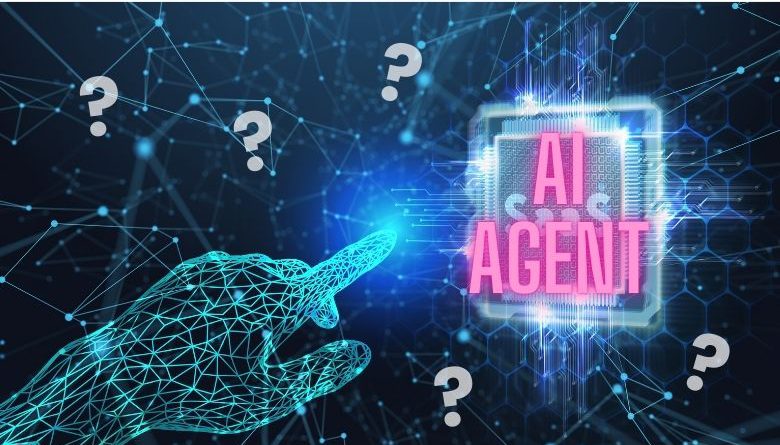
(© putiilch at Canva.com)
How disruptive is the rise of agentic AI going to be for the current generation of enterprise application vendors? In part 1 of this article we looked at the impact of AI agents on SaaS pricing models, but even more fundamental change is coming to how vendors package their products — and services — for customers. Some are even saying that it could spell the end for today’s monolithic applications and bring challenges for incumbents like Oracle, Salesforce and SAP. A blog post earlier this month by Ashu Garg and Jaya Gupta, partners at VC firm Foundation Capital, argues that Systems of Agents will collapse the enterprise stack. They write:
This isn’t just a new category of software; it’s the dismantling of enterprise software as we know it.
That’s quite a claim, which we’ll come back to examine in further detail later on. But it hasn’t come out of nowhere. For the past year, particularly in the VC community, there’s been growing excitement about the huge potential market that could be opened up by combining autonomous agents with software. In this new model, instead of simply providing a tool that people use to get their jobs done, the autonomous agent does the job itself. The vendor is no longer selling a tool, but rather the completed outcome — and potentially eliminating the need for the employee who would previously have used the tool. Partners at Foundation first outlined this concept of an AI-fueled service-as-software paradigm shift back in April, in a blog post co-authored by Gupta and Joanne Chen, another partner at the firm. They provide an example of how this might work in the CRM market:
The shift in business model for sales companies might look like this. Rather than charging customers per seat based on the number of Sales-Development Representatives (SDRs) and Account Executives (AEs) on a platform, software vendors instead charge based on the number of qualified opportunities or signed customers their software delivers.
Note that there’s a shift in the pricing model from charging per-seat to charging by results. But as we discussed in Part 1, that implies a consequent shift from a single license fee for the whole platform to multiple fees for each type of result — one price for each closed deal (possibly with a gradient based on deal complexity), another for renewals, and a range of prices for other outcomes such as resolved support tickets, customer success engagements, and so on. Instead of a monolithic package, the offering becomes a portfolio of interlinked but separate agent outcomes.
The Foundation team don’t discuss pricing or get into any of these details around how it will all be packaged for customers. Their focus in on start-up opportunities, where newcomers are focusing on a specific set of agent capabilities rather than the full spectrum of enterprise needs. Instead, they examine the potential Total Addressable Market (TAM) once you start talking about labor replacement.
In their April blog post, the estimated TAM for such offerings is counted in trillions of dollars, based on projections of these new solutions being able to eat into enterprise salary spending on in-house functions such as sales & marketing, software engineering, security and HR, together with spending on outsourced IT and business process services and salaries. In a paragraph that will chill the blood of any labor union leader that happens across it, they write:
How much work will AI + automation and the Service-as-Software model do away with? We believe this is a $4.6 trillion-dollar question.
Labor replacement
Others in the investment community are eagerly mapping similar opportunities. In a blog post in October, Jake Saper, a partner at SaaS VC firm Emergence Capital, discusses the potential for startups offering AI-enabled services to make headway in highly repeatable ‘job-to-be-done’ services such as cloud migration, application maintenance and penetration testing, bookkeeping and financial reporting, and outsourced customer support. Not as absolutist as the Foundation team, he sees a continuing role for people alongside agents in providing these services, and cautiously notes that it’s a new field with unanswered questions:
These hybrid companies — part AI-enabled software, part people — don’t have a lot of precedent. There are a lot of open questions: Can AI-enabled solutions perform well enough? How to build a trusted brand when clients are already skeptical? Do you hire the same types of people or not?
At Y Combinator, the focus is on vertical markets, but there’s a similar sense that the advent of agents will disrupt incumbent SaaS vendors. A video discussion earlier this month with four partners led by Garry Tan, CEO at Y Combinator, asserts that vertical AI agents could be 10X bigger than SaaS. Summaries by angel investor Ben Lang and investor community Longport provide the gist of the discussion. Again, this is about AI agents replacing both software and labor, particularly where the work is mostly boring, repetitive administration that humans don’t like doing anyway. Illustrative examples from the Y Combinator portfolio include Momentic’s automated QA testing, Triplebyte’s automated software engineer recruitment, Sweetspot to automate responding to government RFPs, and Salient’s AI voice calling for automated debt collection in the auto loan sector.
In her April essay, Foundation’s Gupta uses CRM incumbent Salesforce as an example of how much larger the addressable market becomes when you include labor costs as well:
Salesforce, one of the most successful software companies of all time, generates only $35 billion in revenue annually and will grow incrementally. Global companies spend $1.1 trillion on sales and marketing salaries each year. The size of the opportunity for AI disruption is many multiples larger than Salesforce could ever be.
Two weeks ago, Salesforce showed that it had got the message and wants its own slice of that larger pie. Launching its Agentforce 2.0 platform as a Digital Labor platform, it estimated its Total Addressable Market as high as $7 trillion, based on the labor costs that it could replace. This incumbent, at least, isn’t going down without a fight. But as I said in part 1 of this article, I have several reasons for being skeptical about the figures being bandied about. I think that the value that can be realized from labor replacement is being overestimated, while the difficulties in realizing it are being underestimated.
On the first point, I have to say that some of the labor due to be replaced by autonomous AI sounds almost quaint. One of the Foundation articles includes the example of “entering data and reading faxes.” These are activities that should in most cases already have been automated and which don’t need a new generation of AI agents to enable their automation. As one Reddit commenter put it after listening to the Y Combinator video on vertical AI agents:
This video is 40 mins of empty talk. It can be summarized as ‘if you automate the workflow of your SaaS you’d need less employees and you can work faster therefore you would be bigger than the competition that hasn’t automated their workflows.’
In other words, while it’s true that AI in many cases makes it easier and faster to automate previously manual processes, it’s not that big a deal. These processes were overdue for automation anyway. One aspect of the Y Combinator discussion that I do like is that it emphasizes the importance of marrying AI automation with detailed knowledge of how that vertical actually operates. This is a matter of finally being able to automate processes that it was never previously viable to automate because of cost or complexity.
Dubious assumptions
In an October blog post, Foundation’s Gupta and Chen write about systems of specialized agents collaborating to complete a process. They make the intriguing point that this level of automation opens up the possibility of doing some processes more frequently than was previously possible, such as sales team realignment, or at-scale personalization of customer experiences. They advise:
Some will consider using AI to replace work that humans once did; founders should think about using AI to do work that humans never did, due to time constraints or other limitations.
But while this new technology speeds up the pace of automation, this brings the massive danger of automating processes that were already sub-optimal, or else adding new automations that omit vital steps and safeguards that were never documented. This I think is where the flaws start to emerge in Foundation’s over-optimistic assessment of impending doom for incumbent vendors. Agentic AI is certainly bringing big changes, but it’s not going to be an overnight success. Foundation’s most recent article about Systems of Agents earlier this month makes this sweeping statement about systems of record:
Their core value proposition — being the structured source of truth — evaporates when AI can create structure from natural human workflows.
The authors come to this conclusion after describing how existing enterprise systems have developed over time, with incumbent systems of record gradually overlaid with systems of engagement to make it easier for users to interact with them, followed by an additional layer of systems of intelligence to analyze and report on the data they hold. Now, the argument goes, systems of agents will eat through all of those failing layers and ultimately replace them all. The authors write:
Unlike previous approaches that add complexity by adding layers of tech, Systems of Agents streamline and unify. They eliminate the need for siloed tools like CRMs, marketing platforms, or analytics dashboards by collapsing these functions into a single cohesive system.
The concept is to replace the traditional interactions through forms, buttons and dashboards for capturing and presenting information and replace them with agents that automatically collect and interpret data at source. They go on:
Systems of Agents revolutionize the entry point, replacing human processes at their source and capturing data where it’s born — not where it’s recorded. The interface is reimagined entirely and fades in importance: no more forms or fields, just an organic workflow where data capture happens automatically… Most critically, such systems result in superior data, generating richer, more comprehensive information including both structured and unstructured content.
There are some dubious assumptions built into this rosy picture. It assumes that the data — and the documentation of the processes — is organized, self-evident and complete enough for these autonomous systems to entirely supplant humans while completing the work that needs to be done. The reality, as we’re seeing from real-world experience of enterprises adopting generative AI, is that most of the unstructured data that exists is poorly secured, mapped and catalogued, while processes are often ad-hoc and inadequate, with many of the gaps being filled by largely unrecognized human ingenuity. While generative AI may be able to paper over these cracks and present what appears to be a smoothly operating automated process, it can only work with the data that it has. The likely result of depending too soon on systems of agents is that they will simply expose the many flaws in existing data stores and automations. That’s even before we get into the downsides of using probabilistic logic to interpret and report transactional data.
But leaving aside the overegged ambitions and outlandish TAM calculations, it’s clearly the case that there are emerging opportunities for startups to deliver value and gain market share by automating neglected pockets of repetitive work currently carried out by people. There are no doubt particularly rich pickings in specialized sectors where automation previously wasn’t a sufficiently economic proposition — and for a select few, these may serve as a beachhead from which to expand into other adjacent opportunities and build a broader business. But coming back to the core topic, what does this mean for the future shape of enterprise applications? Here are my thoughts.
My take
Despite all of the red flags I’ve been raising, there’s still little doubt that the Large Language Models (LLMs) that drive generative AI are a hugely impactful technology, particularly because of their emerging ability to actually plan and orchestrate tasks. The impact is likely to be on a par with the creation of the Internet, or cloud computing, or smart mobile technology — and change on this scale inevitably creates new market openings while providing disruptive challenges for incumbents. But even though the current pace of innovation is unprecedented, I think it’s safe to say that when considering enterprise applications, this is one of those cases where big changes will happen over a ten-year time span, even though the next year or two will be crucial for early adopters on the frontiers. Because of budgets, decision cycles and organizational inertia, it simply takes time for changes of this magnitude to go truly mainstream.
Nevertheless, various developments over the past ten years have prepared the ground to make it easier for this latest technology to take hold. Rather than seeing this as a brand new departure, I prefer to see the rise of LLMs as just another chapter in the ongoing evolution of connected digital technologies over the past quarter century, a long-term trend towards what I’ve been calling Frictionless Enterprise.
For example, the notion of decomposing monolithic applications into a more flexible collection of functions and data stores is one that’s been developing for more than a decade now, with online commerce and digital experience platforms at the forefront of the move towards more composable architectures. The advent of AI has simply accelerated the impetus towards joining up data end-to-end across the enterprise and allowing users to access functionality in the flow of work rather than having it siloed in individual applications. This Tierless Architecture, as I’ve called it, provides an IT landscape that is already well suited to work with the new generation of autonomous agents. In this sense, enterprise applications are already primed to break up into more outcomes-oriented agent offerings.
There’s also learned experience that can be helpful as these new agent-based solutions come to market. One of the challenges of a composable architecture in the enterprise market is that managing procurement from multiple vendors is more complex. The MACH Alliance of vendors that offer composable solutions has brokered standardized agreements to help solve this problem. If enterprises are going to end up buying outcome-focused agents from an ecosystem of vendors, they’ll look for similar standardization — or maybe seek out an agent-based solution that handles the problem for them.
But one of the most important principles of Frictionless Enterprise that I keep coming back to is the notion of unbundling and rebundling, in which products or processes that were previously packaged up together are split apart and repackaged in new ways. What we should be looking at is not how agents can automate existing processes, but how they can enable entirely new processes. As I wrote when I outlined these principles:
Frictionless Enterprise is a massive exercise in unbundling and rebundling, as new patterns of digital connection enable the reconfiguration and reinvention of products, processes, entire organizations and even industries.
A case in point is spend management vendor Coupa’s plan to build an AI-enabled network for collaborative sourcing and transactions between buyers and sellers. Instead of aiming to build agents that automate each of the existing processes of managing purchase requisitions, evaluating suppliers and drafting contracts, the vendor is aiming to create a new network of agents that autonomously carry out all of the sourcing, contracting and procurement. Salvatore Lombardo. Chief Product and Technology Officer, says:
If you use AI and just ask the question, ‘What can I automate tomorrow?’, you will gain a little bit of value out of it. But this is not the real disruption. No, the real disruption is combining it with the idea of collaboration, inventing collaboration objects which know each other [and] have the data set, which they can call, discuss, and create things with each other because they’re intelligent agents as bots, talking to each other.
This is an example of how an enterprise process can be completely unbundled and repackaged to operate in a completely new but far more efficient way. But it will take time to conceive and build this new platform, and then even longer to win acceptance for it. Many of these innovative new approaches will depend on the creation of standardized processes and data models, which again take time to coalesce.
This is one of the reasons I’ve paid close attention to the ongoing developments in the realms of digital teamwork, where many of the leading players are evolving their own frameworks for collaboration that could provide foundational templates for process standardization. Thinking about the potential for AI agents in vertical markets, for example, while there may be many opportunities for individual solutions, imagine how much more could be achieved with a platform that any specialist could easily configure to the needs of their own vertical market.
But what about this notion of software-as-services, this idea that software and autonomous agents will combine to create a new class of digital workers to replace today’s salaried knowledge workers? I find it over-simplistic, both in its implication of direct replacement — which as I’ve outlined above, I don’t see as the end game for autonomous agents — and also in its implicit anthropomorphism of AI systems. AI agents are just a new form of automation, and they should be packaged and priced based on the value they create for enterprises, not on the salaries of the people they allegedly replace. And while many people are going to see elements of their existing work taken over by autonomous agents and will often have to retrain or adjust to new responsibilities to stay employed, the disruption doesn’t stop there. Many enterprises will also see their existing revenue sources disappear and will often have to pivot to new propositions to stay solvent.
Over the next few years, we’re going to witness a lot of experimentation. Outcome-based pricing for autonomous agents that perform specific functions will become prevalent, but there will be many setbacks too as suppliers and their enterprise customers adjust to these new terms of trade and the unexpected pitfalls they bring with them. How this will all turn out is anyone’s guess, but it’s going to be an interesting few years.