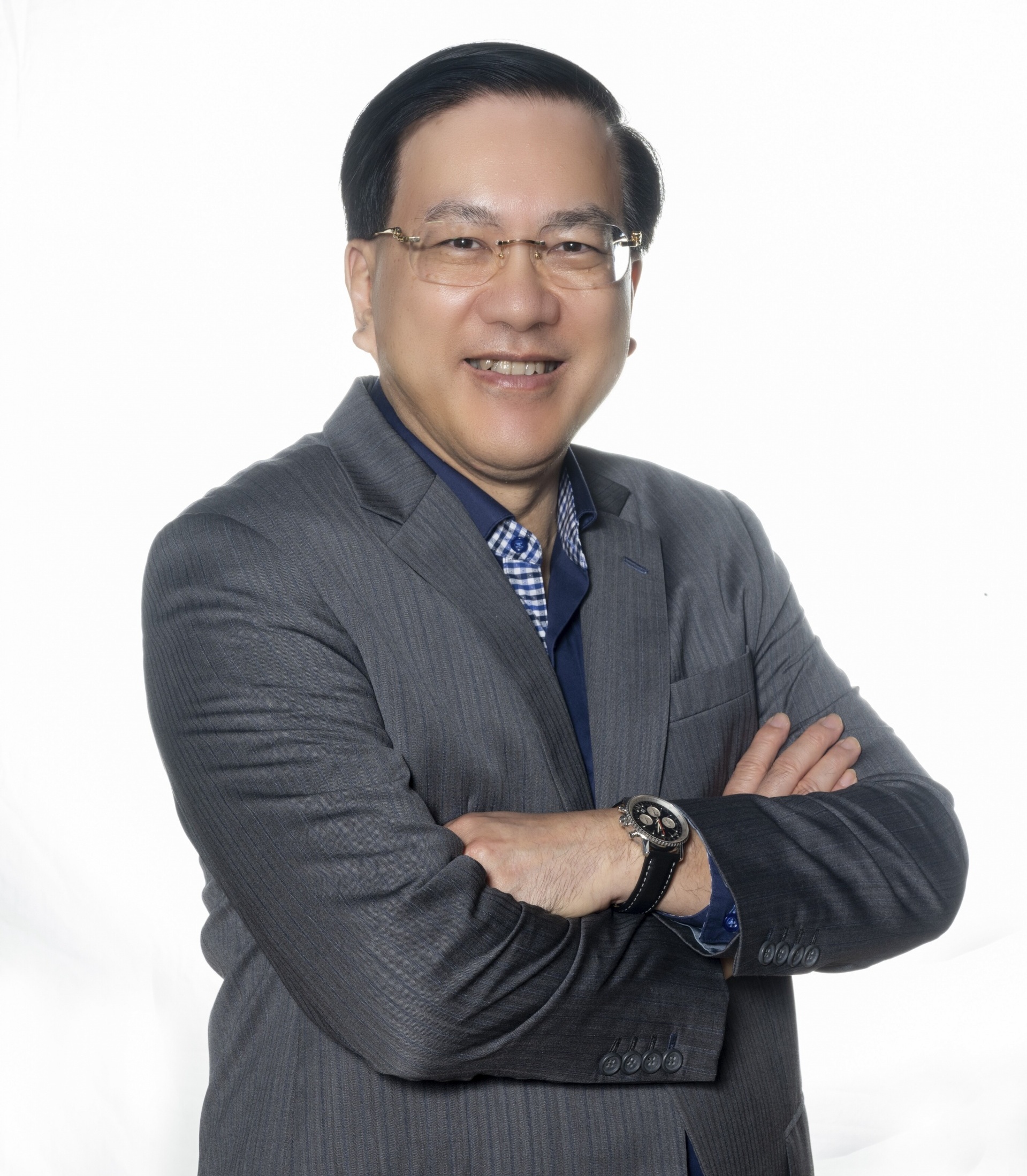
A decade ago, certain types of AI were a curious technology that researchers and enterprises were using to play and win games against humans, as well as achieving some important advances along the way. With new generative AI technologies such as ChatGPT, GitHub Copilot for developers, Sora, and Midjourney, many are now talking about AI and enterprises are utilising these new capabilities.
Christanto Suryadarma, sales vice president for Southeast Asia (SEA), South Korea and Channel APJeC, Zebra Technologies. Photo: Zebra Technologies |
But there is also a world of difference when it comes to consumer versus enterprise use of AI. Budgets, security, use cases, return on investment, and expertise needed to reach new levels in businesses, leaving little room or time to admire the hype. Measurable productivity, process efficiency, workforce optimisation, cost savings, and revenue growth are top of mind.
Business executives rank AI and GenAI among the top three tech priorities for 2024. Yet, 66 per cent of leaders are ambivalent or dissatisfied with their progress on AI and GenAI, and only 6 per cent have begun upskilling in a meaningful way, says a new report by BCG. Yet 54 per cent of leaders expect AI to deliver cost savings in 2024. Of those, roughly half anticipate cost savings in excess of 10 per cent, primarily through productivity gains in operations, customer service, and IT.
Advances in machine vision is one example where we see manufacturers, like other industries, at different levels of maturity when it comes to getting results from AI. Modern machine vision unlocks new levels of analysis, accuracy, compliance, and quality in production processes, and it provides engineers with new tools to work more efficiently â the sorts of things that cut through hype and show the true value of technology.
For example, at one production site, Bosch Group develops solutions for diesel engine injection systems for the automotive industry. The injection nozzles are an important component that transports the diesel fuel into the combustion chamber of the engine. Bosch needed an imaging solution to further automate reading and verification processes, improve the traceability of injection nozzles, and reduce the number of machined parts needing to be checked manually.
With its machine vision system, the plant achieves a production volume of around 7,000 parts per day. The proportion of incorrect rejects has been reduced to less than 5 per cent, which is a significant improvement. The system runs on machine vision software, which controls the entire system and has enabled the team to reduce costs and set-up time and simplify installation.
AI (and users) need training
Deep learning neural networks are powerful, advanced AI tools that mimic the human brain, but they are not magical. It is important to educate stakeholders on the capabilities and limitations of neural networks. Neural networks can achieve remarkable results, but they need to be applied in an educated way. Realistic expectations should be based on the areas where neural networks excel, such as detection of surface defects, detecting or counting objects, reading difficult characters, or detecting unexpected deviations from previously seen objects (anomalies).
![]() |
Photo: Zebra Technologies |
Selecting the appropriate evaluation metrics is essential for accurately assessing model performance. The most basic metric is accuracy, but it may not be suitable for unbalanced datasets. Instead, metrics like F1 score for classification or average precision (area under precision-recall curve for detection tasks. Metrics like area under the receiver operating characteristic that rely on true negatives should be avoided, as the numbers they produce may be misleading, especially when the number of true negatives is very high.
There are also many data issues that need to be addressed in order for a business to reap the benefits of AI. Mixing training and testing datasets, inadequate and unbalanced same sizes, ambiguous and inconsistent data annotation, and environmental factors need to be considered to ensure deep learning solutions work properly.
Approach AI with a plan
Businesses also need guidance to cut through the hype to secure AI-driven value for their operations following the passing of the EU)s AI Act. The EU AI Act sets out a common framework for the use and supply of AI systems in the EU, along with a classification for AI systems with different requirements and obligations tailored on a risk-based approach.
In Vietnam, the Ministry of Science and Technology has likewise proposed that the government approve a national strategy on AI research, development and application by 2030. Citing relevant decisions by the government, Deputy Minister Nguyen Hoang Giang emphasised the need for the country to ensure an open legal corridor and learn from developed nationsâ experience in this field. The Ministry will focus on perfecting relevant policies and expand international cooperation, both bilateral and multilateral, in this realm.
Which manufacturing process requires automation and would benefit from AI? Which type of AI would be most appropriate? How is legal compliance ensured and recorded, who do you need in terms of staff and partners to make it happen? These are the sorts of questions that hype does not answer, but they must be addressed.
Advances without the hype
Presently, it is not so much AI simply creating and eliminating jobs, although many headlines may suggest this. As with the invention of the automobile, telephone, and internet, hosts of new jobs and industries will spring up thanks to the growth of AI. What we see now are manufacturers equipping their engineers, programmers, and data scientists with new and better tools with AI to do what they are doing but faster, more efficiently, and handing over certain tasks to AI-driven automation.
Manufacturers, like other industries, can be challenged by labour hiring, training, and retaining. In these cases, business leaders are turning to automation to fill labour gaps, train workers faster, and assist the workforce they currently have. Workers with AI capabilities will set themselves apart, as they will have the knowledge and expertise manufacturers want in their plants.
Companies will also prioritise the democratisation of AI and machine learning as a strategic priority. Some tools, like deep learning OCR â can be low/no code, meaning they are ready out-of-the-box and do not require specialist training. Other tools are higher end and operate more like ready-made environments for programmers and data scientists to create solutions using the platform, tools, and libraries provided.
Eventually, this approach will be standard rather than a differentiator in the battle for talent, skilling the workforce, and optimising the front line with new ways of working. Those who can introduce and use new AI tools without falling for the hype today will put themselves and their customers at an advantage tomorrow.
![]() |
APAC retailers turn to technology investment to drive profitability
As retailers in Asia Pacific (APAC) feel the omnichannel squeeze, particularly with managing online returns and reducing losses caused by theft, fraud and other factors, they are tapping into the power of technology to drive profitability. |
![]() |
How will the cloud change deep learning machine vision operations for manufacturers?
Christanto Suryadarma, sales vice president for Southeast Asia (SEA), South Korea, and Channel APJeC, Zebra Technologies, explains the benefits of cloud-based deep learning in machine vision operations. |
![]() |
Asia Pacific manufacturers prioritise digital transformation; struggle with innovation pace
Manufacturers around the world are focusing on digital transformation, but achieving that goal is fraught with obstacles, including the cost and availability of labour, scaling technology solutions, and the convergence of IT and operational technology. |