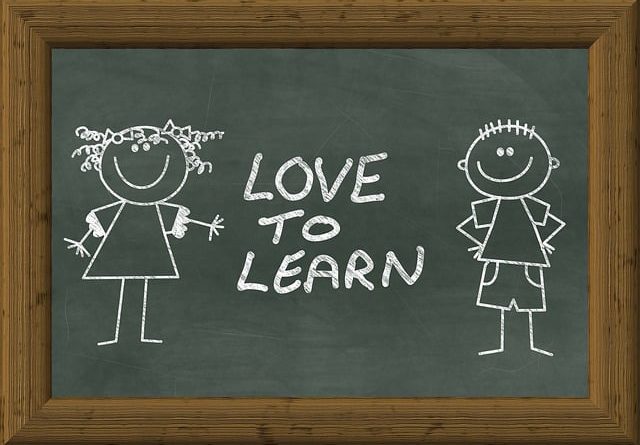
Linus Hakannson
A recent survey of 300 enterprises by Gravitee, an API management vendor, found a mix of enthusiasm for agentic AI adoption with strong caution, particularly around governance, privacy, and security. A striking 72% of organizations claimed to be actively using agentic AI systems. Another 21% planned to implement them in the next two years. How many of these were proof of concepts versus widely used systems is unclear.
Operational efficiency, customer experience, and reduced costs were top drivers. Enterprises were also struggling with integration, privacy and security challenges. Controlling the costs of large language model (LLM) interactions stood out as the top concern. The most common approach was establishing a dedicated agentic AI team as a cross-disciplinary specialty, while nearly as many organizations turned to dedicated data science or engineering teams. About half of these efforts were backed by new budgets.
I connected with Linus Hakansson, Chief Product Officer at Gravitee, to understand the study findings and get his take on the evolution of agentic AI systems in the enterprise. He argues that the survey focused on agentic offerings from OpenAI, Google, Microsoft, and IBM rather than platform-specific efforts because these represent the core foundational and orchestration platforms shaping enterprise adoption of agentic AI at scale.
These providers offer general-purpose capabilities, including Large Language Models (LLMs), tool-use frameworks, and orchestration layers that underpin a broad range of applications across industries. Platform-specific efforts, like Salesforce’s Agentforce, are important but tend to be verticalized or use-case specific. Gravitee plans to investigate these platform-specific efforts in future surveys. Hakansson explains:
Our goal was to understand how enterprises are engaging with the base layers of the stack where decisions about governance, integration, and AI architecture are first made, and how that influences downstream adoption. More specifically, we are looking at how the latest technologies, such as Google’s A2A protocol, enable governance on multi-agent systems, and these frameworks are likely to adopt and benefit from A2A in rapid order.
Is it just FOMO?
So, what’s driving this enthusiasm? In these early days of agentic AI, it’s hard to find much real evidence that enterprises are seeing value today. Hakansson acknowledges that fear of missing out (FOMO) plays a role, particularly for enterprises that missed the cloud or mobile waves. But it also reflects a deeper shift in how we think about automation and intelligence. He argues:
Until now, most AI was reactive. You’d give it a prompt, and it would return a result. But agentic AI introduces autonomy. These systems can plan, take actions, use tools, and even collaborate with other agents or APIs. That’s a step-change, and it captures public imagination because it starts to resemble the kind of AI we’ve seen in science fiction, AI that does, not just responds.
Executives are also cautious because they understand the gap between agentic AI promises in the press and what can be delivered in production. They’re intrigued by the potential, but they’re waiting for clearer guardrails, proven use cases, and ways to govern these agents before scaling across their organizations. Hakansson observes:
The press often frames agentic AI as if it will imminently automate entire companies, but leaders on the ground know better. They’ve been through hype cycles before, cloud, blockchain & metaverse, and they know that transformative technology takes time to mature, integrate, and govern. What’s different here is the complexity. Agentic AI doesn’t just automate tasks. It makes decisions, interacts with systems, and can act autonomously. That introduces real risks around security, compliance, and unpredictable behaviors. Executives aren’t just thinking about, ‘Can we deploy this?’, but, ‘Should we?’,and, ‘How do we control it?’
Let’s talk about value
A little skepticism seems warranted here. Enterprises seem to be struggling to realize value from LLMs used independently. Orchestrating these into agentic systems seems an even more arduous task, not to mention the technical and cultural challenges with reengineering processes.
Hakansson concurs that LLMs alone often don’t deliver clear ROI because they’re used in vague ways, like adding a chatbot no one asked for. Also, customers won’t pay extra unless AI tangibly improves speed, accuracy, or outcomes. However, agentic AI provides a framework for targeting specific, complex processes and breaking them into actionable steps. This can potentially lower costs, reduce errors, and enable new use cases that traditional automation couldn’t touch. He argues it also opens the door to new use cases where traditional automation failed, especially in domains that require judgment, context switching, or integration across tools.
Hakansson says they are seeing real success where agentic AI is applied to targeted, high-friction areas, where legacy automation fell short:
They’re applying agentic AI to well-defined problems with measurable business impact. The lesson for the broader market is clear. Start small, align with strategic pain points, and ensure governance is in place from day one. That’s where value emerges, and where trust in the technology is built. So, the key isn’t just building agents. It’s building the right agents, for the right tasks, with the right guardrails.
Re-shaping the automation stack
Agentic AI builds on a rich history of automation approaches, including API-automation, low-code, and RPA. Each approach brings some advantages and limitations. For reference, Gravitee has previously focused on API automation and event stream orchestration. Here is Hakansson’s take on where these various approaches stand today:
- API-based automation is fast, scalable, and robust. Ideal for integrating well-structured systems. However, it requires strong developer resources and upfront integration work.
- Low-code platforms democratize automation, letting business users build workflows. They are great for agility but can lead to sprawl and governance challenges at scale.
- RPA shines when APIs aren’t available. It’s a quick fix for legacy systems. But it’s brittle, often reliant on UI scraping, and hard to maintain over time.
- Agentic AI is a new layer. It’s adaptive, goal-driven, and capable of reasoning across systems. It doesn’t replace the others but complements them, particularly when logic is dynamic or unstructured.
These bounds were already starting to blur before LLMs came along, and as automation vendors find better ways to use LLMs to generate low-code flows, drive API orchestration, and guide RPA bots. Agentic AI might also evolve as a conductor for deciding which approach to use for a given task. Hakansson predicts:
The real shift isn’t one architecture replacing another. It’s moving from task-based automation to outcome-based orchestration, with AI as the brain coordinating the right tools for the right moment.
Hakansson argues legacy API management vendors have treated governance as an overlay or afterthought with rigid policies that don’t scale across new architectures. Gravitee is approaching this problem with a unified control plane, traditional APIs, event streams like Kafka, and now agentic AI systems. This includes declarative policies and SDKs for developers, and fine-grained observability, real-time decisioning, and tool reporting for governance.
The broader context
Against this backdrop, numerous agentic protocols, frameworks and platforms are all being developed by collaborations. Hakansson says the current focus is on development and orchestration, and governance is often overlooked. To mature this space needs:
- Security controls for identity, scope, and access across agents and tools.
- Risk frameworks to manage unpredictability and LLM drift.
- Cost visibility as agents trigger chains of expensive API or model calls.
- Explainability to trace decision paths and resolve failures.
- Governance hooks that plug into existing IT and compliance systems.
Hakansson predicts:
Just like API management platforms became critical as REST exploded, agentic governance platforms will become non-negotiable as enterprises move from LLM experiments to operationalized AI systems. In the future, governance and risk management won’t be static checklists or after-the-fact audits. They’ll be living systems, dynamic, embedded into workflows, and capable of adapting in real time as humans, AI, and hybrid processes interact. As organizations decompose their operations into smaller, more modular tasks, some human-led, some agent-led, governance will shift from centralized control to distributed, contextual oversight. Every process node, whether it’s a person, an API, or an AI agent, will carry with it embedded policies, risk thresholds, and explainability requirements.
What might this look like in practice?
- Efficiency with accountability: Tasks are automated where possible, but always under watch. Guardrails, audit trails, and rollback mechanisms will be standard.
- Trustworthy automation: LLMs, agents, and other AI components will be certified or constrained based on use case, risk level, and data sensitivity, akin to compliance levels for cloud providers today.
- Democratized governance and compliance: Teams across the business will have self-service visibility and controls, through dashboards, policy builders, and AI copilots, to align their processes with broader governance goals like sustainability and regulatory compliance.
My take
A bold vision! And yet it’s important to remember that the current crop of agentic systems is all built on LLMs that tend to hallucinate confidently. More breakthroughs will be required to realize this vision on a larger scale.
Another major challenge is decomposing existing processes more granularly to see where different kinds of automation can provide the most value and where humans are required for oversight and context. This will be essential for automating or reimagining existing processes.
In the meantime, it seems worthwhile to experiment with small projects to build an understanding of the challenges and opportunities today. This will make scaling agentic AI automation as the foundation models and supporting infrastructure easier. It’s also probably a good idea to keep governance, trust, and long-term value for the enterprise and society at large at the forefront of the conversation. Hakansson hopes:
Ultimately, governance becomes a design-time and run-time concern, built into the architecture of digital transformation, not bolted on. AI will help make governance smarter. But more importantly, governance will make AI safer, more efficient, and more aligned with enterprise values. In the future, governance won’t be a gate. It’ll be a smart mesh woven into every interaction between humans, data, and machines.