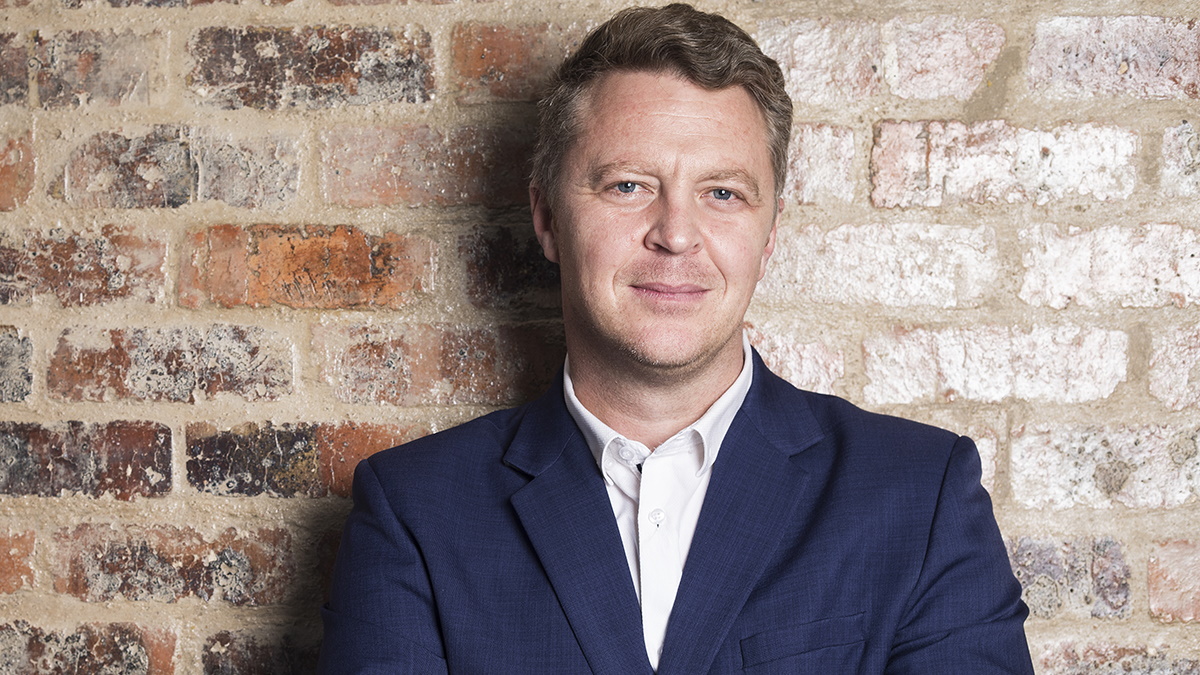
Professor Johan Steyn, founder, AIforBusiness.net.
In the realm of generative artificial intelligence (AI), prompt engineering stands out as a critical skill. This practice involves refining textual inputs to ensure precise responses from AI models.
By strategically crafting prompts, professionals aim to guide AI systems towards more accurate and contextually relevant outputs. It has significantly enhanced AI applications across industries, facilitating better customer interactions, content generation and data analysis.
However, recent discussions raise concerns about its long-term viability amid the rapidly-evolving landscape of AI development.
To optimise AI’s performance and relevance, prompt engineering customises textual inputs using linguistic finesse and deep knowledge of specific AI tools. While pivotal for current AI applications, its future faces intrinsic challenges:
Technological advancements: New AI models increasingly autonomously generate prompts with higher accuracy and relevance. This progress suggests a future where AI systems may require less human intervention in generating textual inputs, potentially reducing the need for specialised prompt engineering skills.
Dependency on AI models: The effectiveness of prompt engineering varies across different AI platforms and versions due to unique response patterns and language nuances. This diversity necessitates tailored approaches that may not generalise well across diverse AI environments.
Sustainability concerns: Discussions highlight the sustainability of prompt engineering as AI technologies advance. The rise of autonomous prompt creation in newer AI models challenges the ongoing relevance of human-engineered prompts, signalling a potential shift in AI interaction paradigms.
In contrast to the fleeting character of prompt engineering, problem formulation emerges as a core ability that brings with it an enduring significance in the period that is driven by AI.
In order to properly define and delineate difficulties, and in addition to establishing the framework for meaningful engagements with AI and novel solutions, problem formulation is required.
Problem formulation is an essential ability for efficiently navigating the intricacies of AI applications.
When prompt engineering is contrasted with problem formulation, the difference between the two becomes abundantly evident. The primary objective of prompt engineering is to improve the relevance of AI replies by optimising textual inputs. In order to construct prompts that effectively elicit desired outputs, this skill requires verbal dexterity, as well as a profound mastery of specific information technology tools.
Problem formulation, on the other hand, focuses on determining the core problem or purpose that AI is intended to handle. To guarantee that interactions with AI are in line with strategic objectives and obstacles that are encountered in the real world, it is necessary to conduct a comprehensive analysis and to frame problems within their proper contexts. Professionals are able to direct AI platforms towards more impactful and specialised solutions by specifying the appropriate problem parameters. As a result, problem formulation is an essential ability for efficiently navigating the intricacies of AI applications.
Professionals can cultivate proficiency in problem formulation using key strategies informed by industry insights:
Problem diagnosis: Involves employing methodologies such as the “Five Whys” to uncover root causes and establish primary objectives for AI-driven solutions. The “Five Whys” technique is a problem-solving approach aimed at getting to the core of an issue by repeatedly asking “why” until the fundamental cause of a problem is identified. Each “why” helps peel back layers of symptoms to reveal deeper underlying factors contributing to the issue.
Problem decomposition: Break down complex challenges into manageable sub-problems, facilitating clarity and targeted solution development in AI interactions.
Problem reframing: Explore alternate perspectives and interpretations of problems to broaden solution horizons and overcome creative bottlenecks.
Problem constraint design: Define boundaries and criteria for exploring AI solutions, aligning efforts with specific contexts and desired outcomes.
Navigating the future alongside AI
While prompt engineering meets current needs for optimising AI interactions, its sustainability amidst rapid technological advancements remains debated. Investing in problem formulation equips professionals with the agility and foresight needed to navigate the evolving AI landscape effectively.
While prompt engineering remains a critical component of current AI systems, its reliance on specific models and methodologies raises concerns about its long-term viability. As the landscape of AI continues to evolve rapidly, there is a growing realisation that placing a stronger emphasis on problem formulation as a core competency is essential.
Mastering problem formulation is increasingly recognised as pivotal for driving innovation, achieving strategic objectives, and fully harnessing the transformative potential of AI. In today’s dynamic environment, where AI technologies are reshaping industries and societal norms, the ability to define and analyse problems with precision is crucial. It allows individuals and organisations not only to adapt to technological advancements but also to proactively shape the future of AI applications.
By prioritising problem formulation, stakeholders can navigate uncertainties effectively and steer AI initiatives towards solutions that are not only technically sound but also strategically aligned with real-world challenges. This approach ensures that AI efforts are focused on addressing root causes rather than just symptoms, thereby enhancing their relevance and impact over time.
As AI becomes increasingly integrated into business operations and everyday life, the ability to master problem formulation emerges as a cornerstone skill for sustainable innovation and strategic success in the AI-driven era.