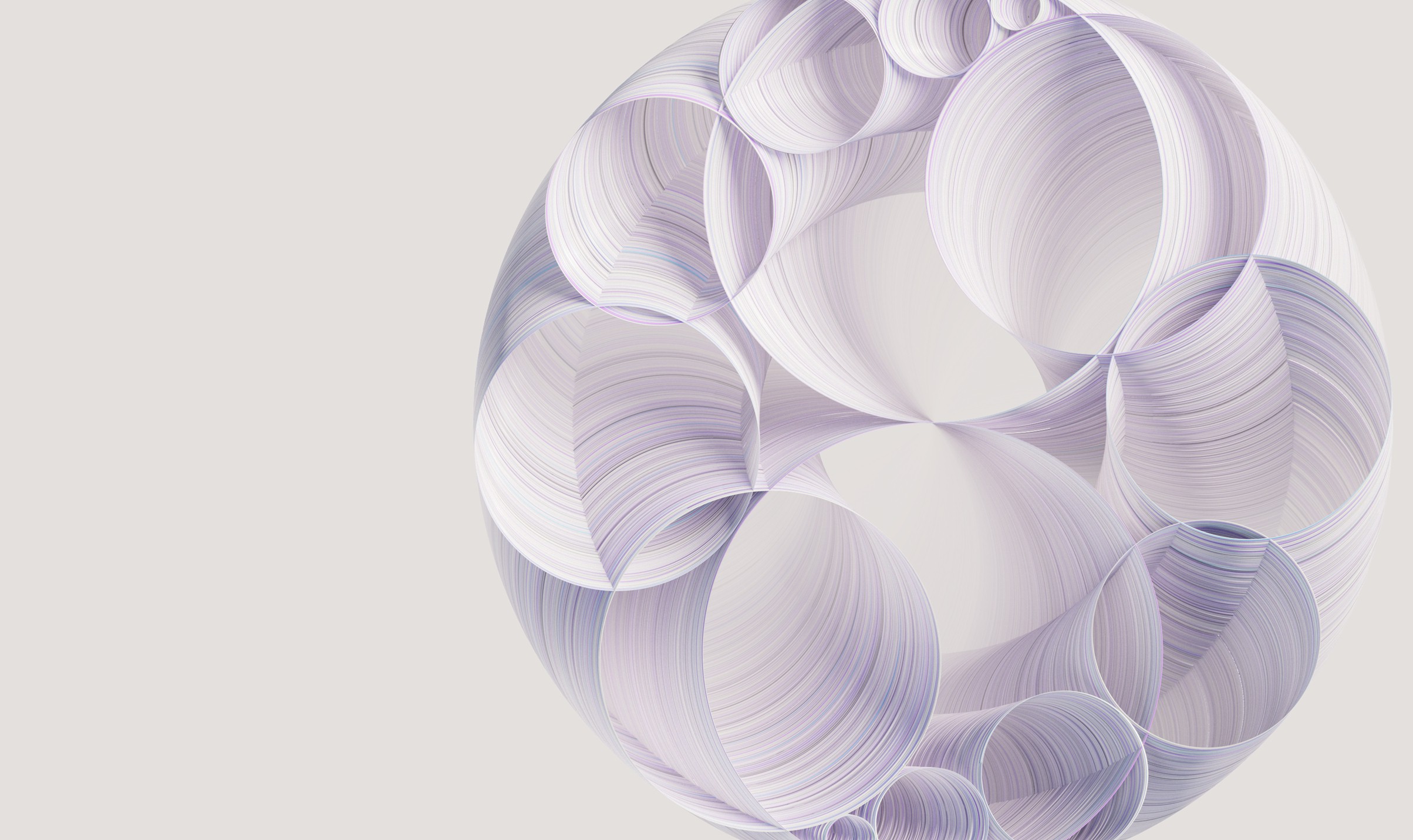
The AI era has a much different vibe than prior eras in the IT industry. The pace of innovation and the amount of investment are both breathtaking. In the two short years since OpenAI’s release of ChatGPT, there has been a sprint from prompt-and-respond with pre-trained large language models to small language models, retrieval-augmented generation, and now agentic AI.
But while research and development has pushed forward at breakneck speed, most enterprises are still not much beyond the starting line, according to Joe Fernandes, vice president and general manager of Red Hat’s AI Business Unit. Fernandes says, however, that they are armed with plans to continue adopting and deploying the technology.
“Just about every enterprise customer I talk to is trying to figure out what its generative AI strategy is going to be,” Fernandes tells The Next Platform. “Enterprises are either running use cases or implementing some initial pilots or proofs of concepts. Some are further along and are actually putting some use cases into production. We are just at the very beginning of this journey and this is going to eventually be pervasive throughout these businesses. I have yet to meet an enterprise customer that’s like, ‘This is just a fad.’”
Right now, most are going down the path of least resistance, Fernandes says, adding that “they’re all starting with what they know, which is, ‘I know about ChatGPT, GPT-4.’ OpenAI is one path, Azure OpenAI, maybe Google. There are these services and these frontier models that are being used.”
Momentum Is Building
Indicators so far are trending up. A study published in October by AI at Wharton, a research arm at Penn University’s Wharton School, and consultancy GBK Collective found that of more than 800 senior business leaders surveyed, their weekly use of generative AI jumped from 37 percent in 2023 to 72 percent this year, an included significant growth in departments like marketing and HR, where adoption of new technologies tends to be slower.
In addition, 55 percent said they use generative AI across business functions and spending on the technology jumped 130 percent since last year, and 72 percent expect their generative AI budgets will grow again in 2025, most more than $5 million. That said, 57% said they expect spending increases to slow in the coming years, likely as they look for ROI from their initial investments and as enterprises start developing organizational structures to support their initiatives.
Step By Step
There are a lot of issues for enterprises to sort through as they adopt the technology, Fernandes says, from managing costs to finding skilled talent to creating the right structure for using it they way they want. They’re taking the necessary steps, he says.
“They’re all on this progression, like prompting a model and prompt tuning and prompt engineering,” Fernandes says. “That’s where everybody starts. then you realize that only effects what’s in the model. It doesn’t affect what’s not in the model. Coupling that with RAG or fine tuning is sort of step two. That gets you further down the path of the current set of use cases I think people are putting into production or piloting, which are the chatbot use cases, the summarization, the classification.”
Then comes agentic AI, which enable systems to work more autonomously to solve problems and address instructions given them. Such autonomous systems go beyond single-shot request-response. Instead, there are multiple steps to execute to create more of a workflow.
“People are just getting started with that thinking. It’s clearly where things are going next,” he says. “But there’s still a lot that people can do with the existing set of tools, like prompting, RAG, fine tuning, just on a single-shot response model. There is still a lot of value you can get from them, but that value grows exponentially as you start to put together these more complex agentic systems.”
As the value grows with agentic AI, so do the costs – given the infrastructure needed – and complexity, Fernandes notes. Given that the data can come from multiple locations, including the edge, and the workload run on premises are the cloud, there also is the need for flexibility. Those are areas of AI that Red Hat is looking to address, he says.
RHEL AI 1.3 Hits The Market
To that end, Red Hat this week unveiled the latest version of its Linux-based AI platform, Red Hat Enterprise Linux (RHEL) AI, which organizations can use to create, test, and run generative AI models for enterprise workloads. RHEL AI 1.3, which is available now, supports Granite models 3.0, mixture-of-expert LLMs that come in sizes of 2 billion and 8 billion parameters that were introduced by IBM in October and released to the open source community via the Apache 2.0 permissive license.
RHEL AI 1.3 also supports Docling, a community project from IBM Research for parsing common document formats and preparing them for AI training and applications by converting them into other formats like Markdown and JSON. In Red Hat’s latest platform, organizations can convert PDFs into Markdown that can be used with InstructLab, an open source project from IBM for enhancing LLMs.
In addition, the latest platform version is adding support for Gaudi 3, an accelerator and Intel’s response to Nvidia’s highly successful GPUs, including the latest Blackwell family. RHEL AI 1.3 already supports Nvidia and AMD GPUs. RHEL AI also will soon be available via Amazon Web Services’ (AWS’) and Microsoft Azure’s cloud marketplaces.
OpenShift AI, which already supports RHEL AI, is expanding its reach by using vLLM runtimes to support parallelized serving across multiple nodes, which means it can handle multiple requests in real time.
Red Hat’s Collaboration With IBM
The ability to leverage such AI tools as Granite models and InstructLab illustrate the collaboration between Red Hat and parent company IBM in the AI field, fueled in part by Big Blue’s openness to open source, according to Fernandes.
“The partnership we have with IBM Research and IBM’s decision to really embrace open source by opening up the Granite models, open sourcing the InstructLab tools, and the work they’re doing in infrastructure, like Hydra, this Red Hat AI work wouldn’t be possible without that collaboration,” he says.
Red Hat is working with IBM’s research, software, cloud, and other teams, and leveraging its consulting and sales reach. Fernandes points to IBM’s watsonx AI assistant and Red Hat’s AI offerings as an example of the mutual benefits both vendors derive from the collaboration.
“RHEL AI is our Linux-based AI platform for running on individual servers,” Fernandes says. “We then take all that technology and also build it into OpenShift AI, which has that core but also has additional capabilities, because now you’re running in a Kubernetes-based distributed environment, you have more management and MLOps capabilities around that. Then RHEL AI and OpenShift AI get baked into watsonx and watsonx leverages that. There are broader solutions, like watsonx.data and then watsonx.ai, which sort of add above and around.”
The Granite models originated with IBM – it still does the pre-training – and were open sourced, but Red Hat also supports and ships them, he says. Similarly, InstructLab was developed by IBM Research, but that engineering team is now part of Red Hat.
Featuring highlights, analysis, and stories from the week directly from us to your inbox with nothing in between.
Subscribe now