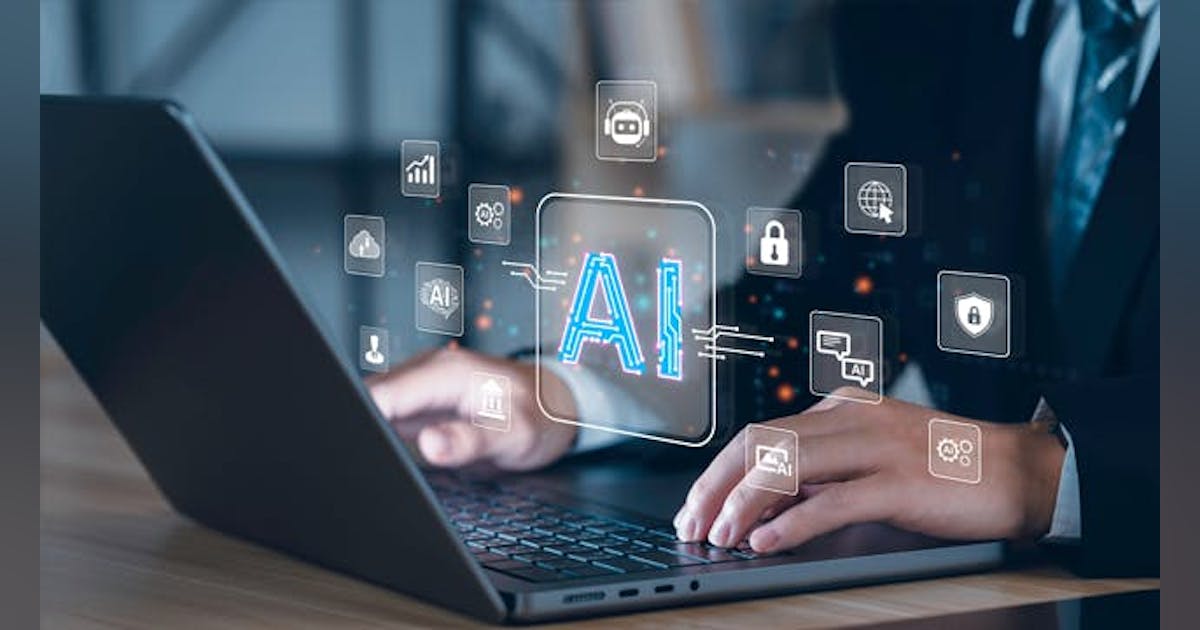
What is prompt-based engineering, and how is AI pivoting in that direction?
Beyond asset performance management, ABB sees potential for GenAI in key focus business areas: sustainability, operational excellence, process performance management, cybersecurity and extended automation.
“Generative AI is reinventing industry,” Ninan says. “The manufacturing industry has been evolving in the last few years to adopt ensemble models, driven through traditional physics-based models integrated with data-driven models. There is a strong transition from analytics AI to prompt-based engineering.”
Applied to industrial design, prompt-based engineering solves for a specific desired result, using AI/ML. “With generative AI (driven by prompt engineering), there is a huge potential for generating some of these outcomes based on the power of large language models,” Ninan says. Prompt-based engineering could be used in the generation of text, images, code, voice or video, and for anomaly detection, predictive maintenance, process optimization, visual data enhancement, quality control or demand forecasting.
Traditionally, he adds, the key driver for automation has been first principles models, or physics-based modeling of isolated equipment in a system, and it required manual intervention and interpretation. “With the adoption of AI/ML, more data-driven models started taking prominence for more complex and non-linear systems. However, AI/ML has its drawbacks such that it used to work for only those datasets for which it was modeled with attributes of anomalous conditions,” Ninan says. Now, the power of industrial AI, he says, is the combination of AI with industrial domain knowledge, or the ensemble approach to modeling.
Data ingestion and modeling
GenAI has the potential to automate repetitive tasks and content creation, such as creating visualizations for asset performance data or potential failures or other deeper data analysis. It can also create personalized user experiences, where large language models, which power generative AI, enable code, image and text generation.
Generative modeling uses deep learning techniques, Ninan says, which are variants of recurrent Neural Networks (RNNs), an artificial neural network commonly used in speech recognition and natural language processing or transformer, a deep learning architecture used in natural language processing, computer vision and audio and multi-modal processing.
GenAI models can help industry automate content creation for marketing, documentation, or customer support. It can also speed up software development and integration by assisting developers with code generation. Generative models like OpenAI’s DALL-E can create images from textual descriptions, which could aid in design, content creation, or data augmentation, Ninan says. “Generative models can generate synthetic data, which is valuable for training ML models when real data is limited or sensitive. This improves model robustness and generalization,” he adds.
Advanced analytics with AI capability enable data ingestion from the edge to the cloud and from multiple systems. AI can help not only collect data from different sources, but contextual, categorize and analyze data from different sources. “For industrial analytics, the majority of data comes from IoT, sensors, instruments and assets. These data could be of various types such as IT, OT, geospatial, engineering, schematic (pdf, jpg, etc.). Data Ingestion refers to the effective way of extracting data from each of these sources into an application context or a storage context,” Ninan says.