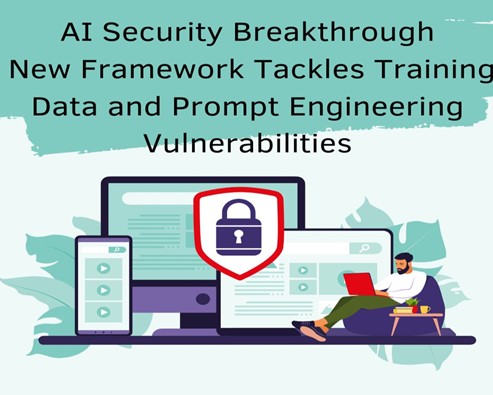
In a significant advancement for AI security, Manojava Bharadwaj Bhagavathula focuses on strengthening generative AI systems against security threats. His framework presents novel approaches to two key challenges in AI security: safeguarding training data integrity and mitigating prompt engineering vulnerabilities. This work comes at a crucial time as organizations increasingly deploy AI systems in mission-critical operations, representing a significant advancement in AI security design.
The Rising Challenge of AI Security
The integration of generative AI into enterprise environments has exposed critical security gaps that traditional cybersecurity measures fail to address. Recent findings reveal that data management vulnerabilities account for 34.5% of security breaches, while prompt engineering exploits contribute to 28.9% of incidents. These statistics highlight the urgent need for specialized security frameworks tailored to AI systems, as organizations face mounting pressure to protect their AI infrastructure while maintaining operational efficiency and innovation capabilities.
A New Paradigm in Data Protection
The research introduces an innovative approach to securing training data, focusing on detecting and preventing sophisticated manipulation attempts. A key discovery shows that even minimal data poisoning – affecting just 0.001% of training tokens – can significantly impact model behavior while evading standard detection methods. The framework implements multi-layered validation protocols that can identify subtle manipulations at both syntactic and semantic levels.
Advanced Prompt Engineering Security
The study presents groundbreaking insights into prompt engineering vulnerabilities, revealing that approximately 37% of carefully crafted adversarial prompts can bypass conventional security filters. To counter this, the framework introduces dynamic response validation mechanisms and context-aware boundary enforcement, significantly improving the detection of malicious prompt patterns.
Quantum-Ready Protection
Looking ahead, the research anticipates the convergence of quantum computing with AI security challenges. The framework incorporates forward-thinking measures to address emerging quantum-enhanced attacks, positioning organizations to defend against future threats. This includes adaptive security protocols that can evolve alongside technological advancements.
Real-time Threat Detection
A major innovation lies in the framework’s real-time monitoring capabilities. The system can analyze patterns in model interactions and system behaviors, providing immediate alerts for potential security breaches. Testing shows this approach can capture up to 91.9% of harmful content with an impressive F1 score of 85.7%.
Resource-Efficient Implementation
The framework addresses practical implementation challenges by optimizing resource usage. While comprehensive security monitoring can increase computational overhead by 15-30%, the system includes intelligent resource allocation mechanisms that help maintain model performance and response times.
Setting New Industry Standards
The research establishes concrete guidelines for implementing AI security measures across organizations. This includes standardized testing procedures, automated security scanning protocols, and clear incident response frameworks. The approach emphasizes the importance of balancing security requirements with operational efficiency.
Future-Proof Security Architecture
The framework’s adaptive nature ensures it can evolve with emerging threats. It incorporates self-healing capabilities and continuous learning mechanisms, allowing security measures to automatically adjust to new attack patterns. This forward-looking design helps organizations stay ahead of evolving security challenges.
The system’s self-healing mechanisms operate on multiple levels, from automatic patch deployment to dynamic security rule updates. When potential vulnerabilities are detected, the framework can automatically implement temporary containment measures while developing long-term solutions.
Practical Impact and Implementation
Organizations implementing these security measures have witnessed remarkable improvements in detecting and preventing AI-related security incidents. The framework seamlessly integrates with existing security infrastructure while offering specialized AI protection. Early adopters have reported substantial reductions in vulnerabilities, with monitoring systems identifying potential threats before they impact operations. The framework has proven valuable in high-stakes environments, successfully scaling from small AI deployments to enterprise-wide systems. Performance data shows organizations using this framework have achieved up to 85% improvement in threat detection rates while reducing false positives. The system excels at distinguishing between legitimate model behavior and security threats, enabling teams to focus on genuine concerns. The framework ensures adaptability to diverse security needs.
In conclusion, Manojava Bharadwaj Bhagavathula’s work represents a significant step forward in securing generative AI systems. The framework’s comprehensive approach to addressing both current and emerging security challenges provides organizations with practical tools for protecting their AI investments while ensuring sustainable long-term security.