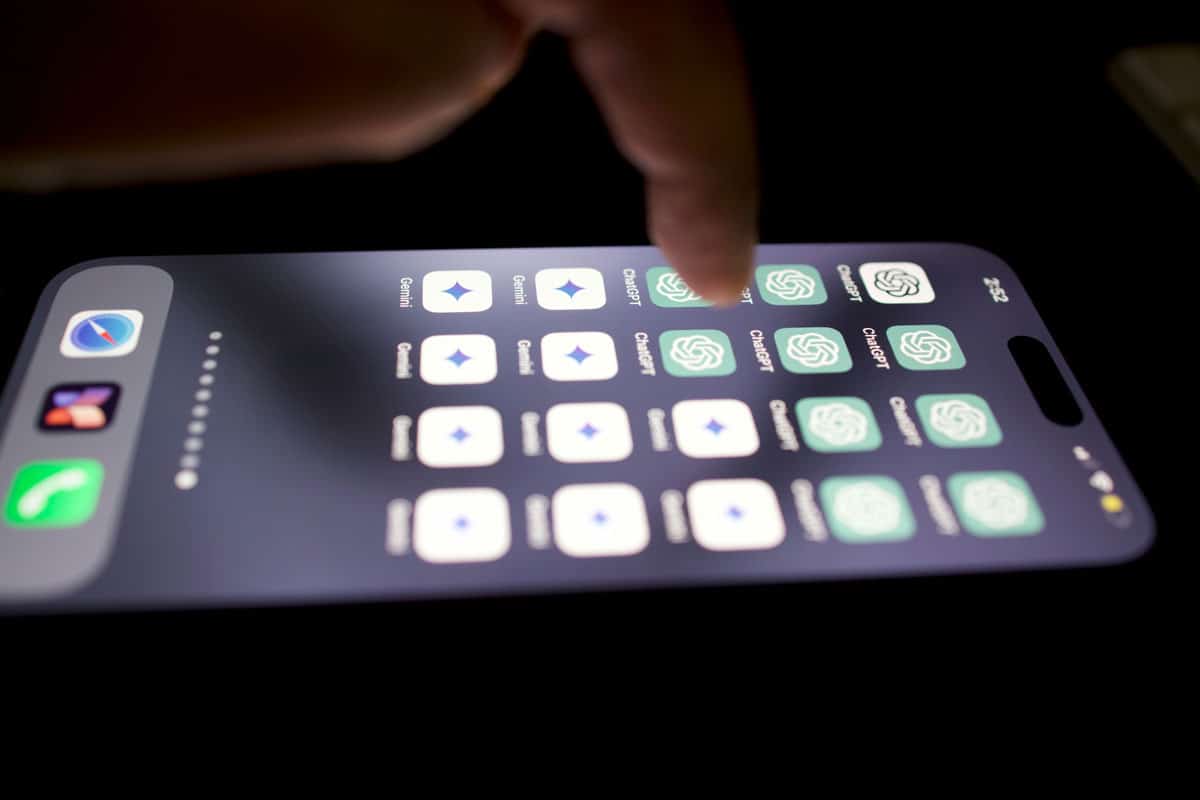
Many international education marketers are now using ChatGPT, whether it’s the free version (GPT-3) or the paid version (GPT-4, which currently costs about US$20 a month). As in other industries, those who use it tend to cite the time savings it allows them in their work – assuming they get the results they are looking for. If results are disappointing, however, using ChatGPT can feel like a waste of time and frustrated users often go back to doing things the way they usually do.
This is where understanding a concept called “prompt engineering” can be extremely useful. Speaking during a recent ICEF webinar, “ChatGPT for Education Marketing: Opportunities, Strategies, and Outcomes,” Philippe Taza, Founder & CEO, HEM Education Marketing Solutions, explained that prompt engineering is “the practice of designing inputs for generative AI tools that will produce optimal outputs.”
How to get helpful results from ChatGPT
A poll of the live audience for the webinar indicated that a discussion of prompt engineering was timely. When asked what they struggle with the most when it comes to ChatGPT, 45% said “crafting the right prompt to get desired responses,” ahead of “understanding the AI’s capabilities and limitations” (27%), “integrating ChatGPT into existing workflows” (24%), and “managing token limitations and consumption” (4%).
One of the most common uses of ChatGPT is to generate text, and as any writer knows, creating text relies on developing ideas and strategising the best way of presenting them. It isn’t an instant process but rather a series of steps. Therefore, it makes sense that ChatGPT needs more than a hurried “I need this and here are the basics” sort of prompt.
Mr Taza says that in his work, he is guided by the iterative process of “Prime, Prompt, Polish.” According to this logic, you begin your work with the AI by priming it (i.e., setting the general groundwork for the AI to consider its task), then you prompt it by giving it all the information it needs to deliver on its task, then you and the AI polish it, which is a bit like a final edit to make it the best it can be.
Mr Taza provided the example of a fictional college in Toronto, Canada. The context provided for the AI was this:
Next in the process is the prompt. Note in the below screenshot that Mr Taza zones in on how important it is to ask the AI if it has what it needs to complete the task.
Join 37,000 subscribers
and stay up to date on International Recruitment
It turns out the AI did, in fact, need a lot more information to do its job well, as the next screenshot shows.
When we think of AI and what we want it to do, often we are looking for time savings. But paradoxically, slowing the AI down a bit (politely) is key to receiving good results, as the next slide shows. (Of course, “slowing down” is relative in this context as the AI will still be working incredibly quickly.)
As the next screenshot shows, the AI is able to deliver good results – and the screenshot after this shows that the prompter then asks it for further help – in a very encouraging way.
After this, the next few slides, which you can view here in the recorded webinar, show what the GPT developed for this task – and then what it was able to do when asked to think a bit more deeply about the subject matter. This is the “polish” phase where it’s the work done by the human and the AI – not just the AI – that drives optimal results. You can see below that the AI crafts some pretty great calls to action – but again, that it wouldn’t have done such a good job without the skill of the prompter.
As Mr Taza notes:
“It’s not always going to happen that You’re not always going to get what you want from the first exchange. You can drill down into sections of what it gave you (e.g., add a new section, provide additional calls to action) and you can see the ‘before’ and ‘after’ and that the “after” is a lot richer.”
For additional background, please see: