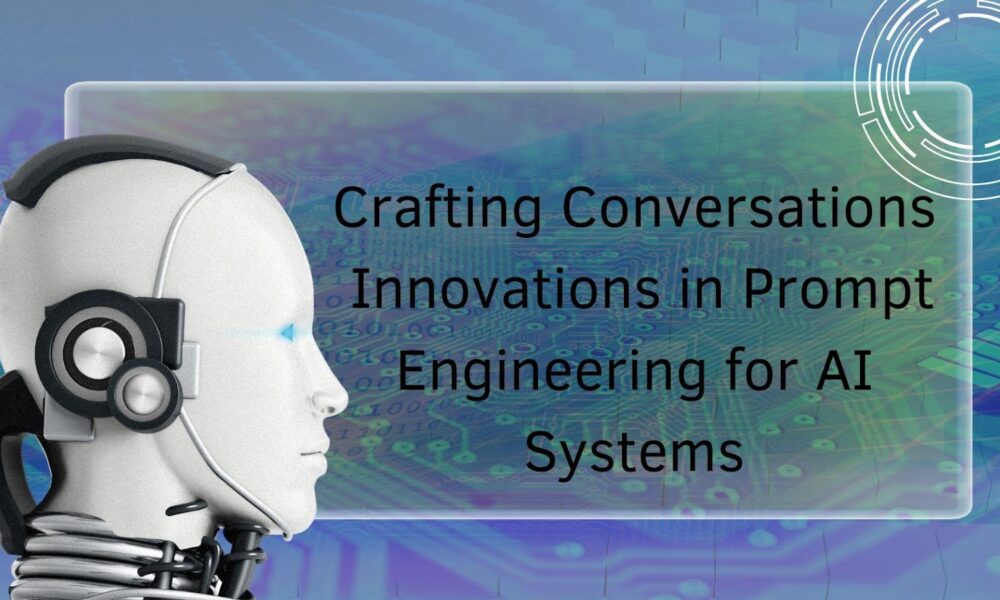
In a rapidly evolving technological landscape, prompt engineering stands at the forefront of advancements in artificial intelligence (AI). This emerging discipline, extensively explored by Panneer Selvam Viswanathan, is transforming how humans interact with large language models (LLMs) by focusing on innovative techniques and applications that provide a roadmap for optimizing AI interactions across diverse domains.
A New Era of AI Interactions
Prompt engineering transforms basic input-output processes into sophisticated, context-aware exchanges. At its core, it emphasizes clarity, contextual framing, and precision in language to guide AI systems effectively. These carefully designed prompts enhance the AI’s ability to generate accurate, relevant, and human-like responses. This innovation not only refines the usability of conversational AI but also addresses challenges like response consistency and contextual relevance in extended conversations.
The Building Blocks of Effective Prompts
Clarity and specificity are paramount in prompt engineering. These factors ensure that language models comprehend user intent without ambiguity. By integrating context—be it background information or domain-specific details—engineers can shape the AI’s responses to be more accurate and purpose-driven.
Instructional phrasing is another vital component, involving strategic use of language that aligns with the AI’s training paradigms. These structured prompts, balanced in length and complexity, reduce computational overhead while maintaining precision. This thoughtful approach enables AI to perform well across simple and complex tasks.
Advanced Techniques Pushing Boundaries
Among the innovations in prompt engineering, techniques such as prompt chaining and few-shot learning stand out. Prompt chaining involves breaking down complex queries into sequential steps, each building on the other, to improve the AI’s reasoning process. This method proves invaluable in scenarios requiring multi-stage problem-solving, like data analysis or creative content generation.
Few-shot learning is another breakthrough, enabling AI to generalize tasks with minimal examples. Carefully curated input-output pairs serve as demonstrations, allowing the model to adapt quickly and efficiently. This approach highlights the growing potential of AI systems to learn on the fly without exhaustive datasets.
Contextual Framing: Enhancing Relevance
Effective communication with AI requires the integration of contextual framing. This technique involves embedding situational and background details into prompts to ensure the AI generates relevant outputs. Whether defining operational parameters or specifying desired outcomes, contextual framing bridges the gap between user intent and machine comprehension.
Scenario development is critical, allowing engineers to craft prompts that align with specific use cases. By iteratively refining context, AI outputs can be tailored to meet the nuanced demands of healthcare, finance, and education sectors.
Tuning AI Through Parameters
Optimizing AI behavior is deeply influenced by fine-tuning parameters such as temperature settings and sampling techniques. Lower temperature values guide the AI toward more deterministic and predictable outputs, ideal for tasks requiring consistency and accuracy. Conversely, higher temperatures introduce randomness, fostering creativity and diverse responses. Sampling methods like top-k filtering, which selects from the top-k most probable tokens, and nucleus sampling, which considers tokens within a cumulative probability threshold, further refine the balance between precision and variability. By leveraging these techniques, developers can customize AI outputs to align with specific task requirements, ensuring optimal performance across a wide range of applications.
Looking Ahead: Automated and Multimodal Innovations
The future of prompt engineering is driven by automation and the integration of multimodal applications. Automated systems for prompt generation leverage machine learning to create, refine, and adapt prompts at scale, enabling efficient and dynamic deployment in various contexts. Multimodal prompt learning further advances this field by incorporating diverse input types, such as text, images, audio, and structured data. This approach unlocks new opportunities for cross-domain interactions, allowing AI systems to interpret and respond to complex, real-world scenarios more effectively. Together, these advancements signal a paradigm shift in AI, enhancing the depth, adaptability, and versatility of machine learning applications.
In conclusion, Panneer Selvam Viswanathan’s insights into prompt engineering underscore its transformative impact on AI interactions. By leveraging advanced techniques like prompt chaining, contextual framing, and parameter optimization, this field is addressing critical challenges and setting new standards for AI reliability and usability. As automation and multimodal capabilities gain traction, prompt engineering is poised to redefine how we communicate with intelligent systems, fostering innovation across industries.