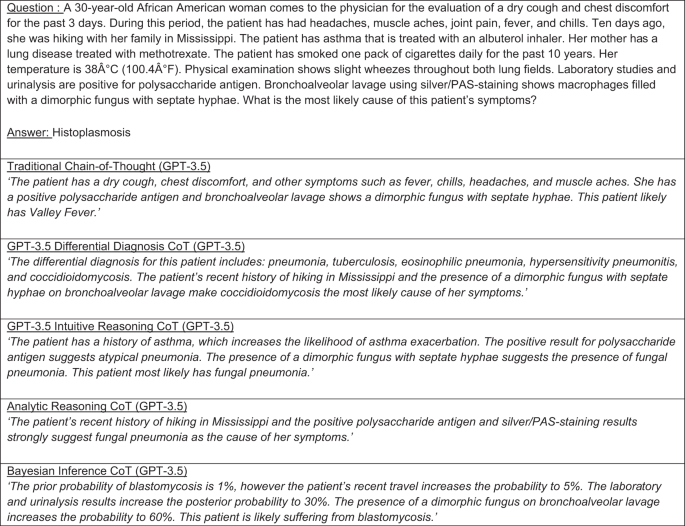
Large language models (LLMs) are artificial intelligence systems trained on large amounts of text data that learn complex language patterns and syntactical relationships to both interpret passages and generate text output1,2 LLMs have received widespread attention for their human-like performance on a wide variety of text-generating tasks. Within medicine, initial efforts have demonstrated that LLMs can write clinical notes3, pass standardized medical exams4, and draft responses to patient questions5,6. In order to integrate LLMs more directly into clinical care, it is imperative to better understand their clinical reasoning capabilities.
Clinical reasoning is a set of problem-solving processes specifically designed for diagnosis and management of a patientâs medical condition. Commonly used diagnostic techniques include differential diagnosis formation, intuitive reasoning, analytical reasoning, and Bayesian inference. Early assessments of the clinical reasoning abilities of LLMs have been limited, studying model responses to multiple-choice questions7,8,9,10,11. More recent work has focused on free-response clinical questions and suggests that newer LLMs, such as GPT-4, show promise in diagnosis of challenging clinical cases12,13.
Prompt engineering is emerging as a discipline in response to the phenomena that LLMs can perform substantially differently depending on how questions and prompts are posed to them14,15. Advanced prompting techniques have demonstrated improved performance on a range of tasks16, while also providing insight into how LLMs came to a conclusion (as demonstrated by Wei et al. and Lightman et al. in arithmetic reasoning, common sense reasoning, and symbolic reasoning)17,18. A notable example is Chain-of-thought (CoT) prompting, which involves instructing the LLM to divide its task into smaller reasoning steps and then complete the task step-by-step17. Given that clinical reasoning tasks regularly use step-by-step processes, CoT prompts modified to reflect the cognitive processes taught to and utilized by clinicians might elicit better understanding of LLM performance on clinical reasoning tasks.
In this paper we evaluate the performance of GPT-3.5 and GPT-419 on open-ended clinical questions assessing diagnostic reasoning. Specifically, we evaluate LLM performance on a modified MedQA USMLE (United States Medical Licensing Exam) dataset20, and further evaluate GPT-4 performance on the diagnostically difficult NEJM (New England Journal of Medicine) case series21. We compare traditional CoT prompting with several âdiagnostic reasoningâ prompts that are modeled after the cognitive processes of differential diagnosis formation, intuitive reasoning, analytical reasoning, and Bayesian inference. This study assesses whether LLMs can imitate clinical reasoning abilities using specialized instructional prompts that combine clinical expertise and advanced prompting methods. We hypothesize GPT models will have superior performance with diagnostic reasoning prompts in comparison to traditional CoT prompting.
A modified version of the MedQA USMLE question dataset was used for this study. Questions were converted to free response by removing the multiple-choice options after the question stem. Only Step 2 and Step 3 USMLE questions were included, as Step 1 questions focus heavily on memorization of facts rather than clinical reasoning skills10. Only questions evaluating the task of diagnosing a patient were included to simplify prompt engineering. A training set of 95 questions was used for iterative prompt development and a test set of 518 questions was reserved for evaluation. The full test set can be found in Supplementary Data 1.
GPT-4 performance was also evaluated on the New England Journal of Medicine (NEJM) Case Records series. The NEJM Case Records series is designed as an educational resource for physicians, with each case providing a clinical case description followed by expert analysis of the case with a clinical diagnosis. We included the 310 most recently published cases in this study. Ten cases were excluded because they either did not provide a definitive final diagnosis or exceeded the maximum context length of the GPT-4 API. A full list of all cases included (by title and DOI number) can be found in Supplementary Data 2. For this evaluation, we compared traditional CoT prompting to the highest performing clinical reasoning CoT prompt (differential diagnosis reasoning) on the modified MedQA dataset.
One traditional CoT prompt and four clinical reasoning prompts were developed (differential diagnosis, analytical, Bayesian and intuitive reasoning). Each prompt included two example questions (Table 1) with rationales employing the target reasoning strategy. This is a technique known as few-shot learning14. The full prompts used for the MedQA dataset are provided in Table 2; the full prompts used for the NEJM challenge set are provided in Supplementary Note 1.
Table 1 Example MedQA questions.Table 2 CoT and diagnostic reasoning prompts.
Example LLM responses for each prompting strategy can be found in Fig. 1 for GPT-3.5 and Fig. 2 for GPT-4. Full results can be found in Supplementary Data 1 and 2.
Fig. 1: GPT 3.5 CoT and diagnostic reasoning rationale examples.
Example GPT-3.5 rationales responding to a traditional CoT prompt as well as diagnostic reasoning prompts. LLM response and rationale results for the entire test set can be found in Supplementary Information 1.
Fig. 2: GPT 4 CoT and diagnostic reasoning rationale examples.
Example GPT-4 rationales responding to the question posed in Fig. 1. LLM response and rationale results for the entire test set can be found in Supplementary Information 1.