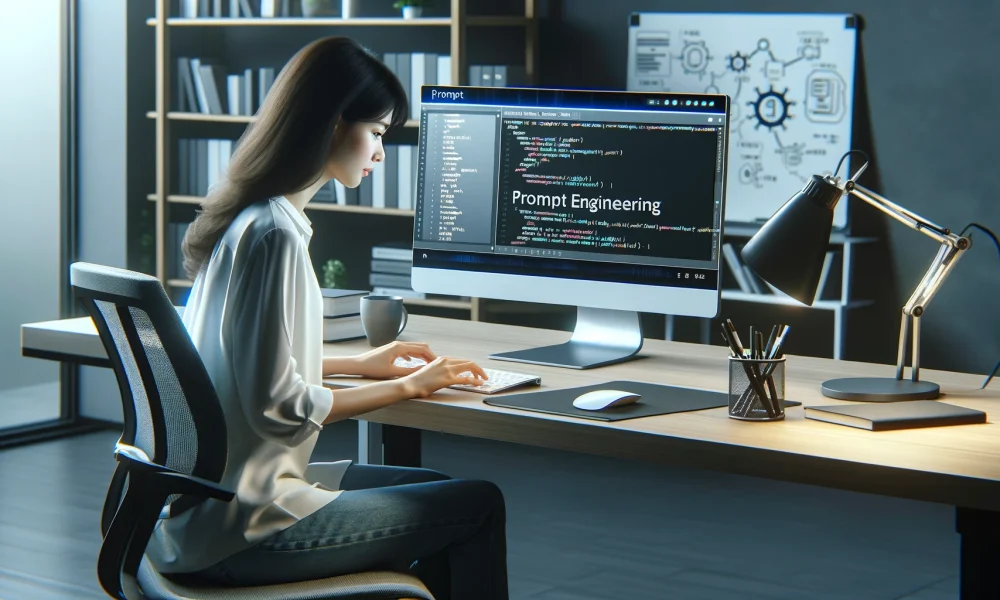
Artificial Intelligence (AI) has witnessed rapid advancements over the past few years, particularly in Natural Language Processing (NLP). From chatbots that simulate human conversation to sophisticated models that can draft essays and compose poetry, AI’s capabilities have grown immensely. These advancements have been driven by significant breakthroughs in deep learning and the availability of large datasets, allowing models to understand and generate human-like text with significant accuracy.
Two key techniques driving these advancements are prompt engineering and few-shot learning. Prompt engineering involves carefully crafting inputs to guide AI models in producing desired outputs, ensuring more relevant and accurate responses. On the other hand, few-shot learning enables models to generalize from a few examples, making it possible for AI to perform well on tasks with limited data. By combining these techniques, the prospects for AI applications have significantly broadened, leading to innovation across diverse domains.
Understanding Prompt Engineering
Crafting effective prompts for AI models is both an art and a science. It involves understanding the model’s capabilities and limitations and predicting how different inputs will be interpreted. A well-designed prompt can make all the difference between a coherent, relevant response and a nonsensical one. For example, a refined prompt with clear instructions significantly enhances the quality of the AI’s output.
Recent advances in prompt engineering have introduced systematic approaches to creating these inputs. Researchers have developed frameworks like prompt tuning, where the prompt is optimized during training. This enables models to learn the most effective prompts for various tasks, resulting in better performance across different applications. Tools like the OpenAI Playground allow users to experiment with prompts and observe real-time effects, making Large Language Models (LLMs) more accessible and powerful.
The Emergence of Few-Shot Learning
Few-shot learning is a technique in which models are trained to perform tasks with few examples. Traditional machine learning models require large amounts of labelled data for high performance. In contrast, few-shot learning models can generalize from a few examples, making them highly versatile and efficient.
Few-shot learning relies on the pre-trained knowledge of large language models. These models, trained on vast amounts of text data, already deeply understand language patterns and structures. When provided with a few examples of a new task, the model can apply its pre-existing knowledge to perform the task effectively.
For instance, if an AI model has been pre-trained on a diverse corpus of text, it can be given just a few examples of a new language translation task and still perform well. This is because the model can draw on its extensive language knowledge to infer the correct translations, even with limited specific examples.
Recent Advances in Few-Shot Learning
Recent research in few-shot learning has focused on improving the efficiency and accuracy of these models. Techniques like meta-learning, where models learn to learn, have shown promise in enhancing few-shot learning capabilities. Meta-learning involves training models on various tasks to adapt quickly to new tasks with minimal data.
Another exciting development is contrastive learning, which helps models distinguish between similar and dissimilar examples. By training models to identify subtle differences between examples, researchers have improved the performance of few-shot learning models across diverse applications.
Data augmentation is another technique that is gaining traction in few-shot learning. By generating synthetic data that mimics real-world examples, models can be exposed to various scenarios, improving their generalization capabilities. Techniques like Generative Adversarial Networks (GANs) and Variational Autoencoders (VAEs) are commonly used.
Finally, self-supervised learning, where models learn to predict parts of their input from other parts, has shown potential in few-shot learning. This technique enables models to use vast amounts of unlabeled data to build robust representations, which can be fine-tuned with minimal labelled examples for specific tasks.
The Case of IBM Watson and Camping World
The following examples highlight the effectiveness of prompt engineering and few-shot learning:
Camping World, a retailer specializing in recreational vehicles and camping gear, faced challenges with customer support due to high inquiry volumes and long wait times. To improve customer engagement and efficiency, they implemented IBM’s Watsonx Assistant.
By refining AI prompts through prompt engineering, such as specific prompts like “Please describe your issue with your recent purchase,” the virtual agent, Arvee, could gather precise information and handle more inquiries accurately. This led to a 40% increase in customer engagement, a 33% improvement in agent efficiency, and average wait times dropping to 33 seconds. Prompt engineering enabled the AI to ask relevant follow-up questions and effectively manage a broader range of inquiries.
The Future of AI Model Enhancement
As AI evolves, prompt engineering and few-shot learning will play increasingly important roles in enhancing model responses. Future AI models will likely incorporate more sophisticated personalization techniques, using both prompt engineering and few-shot learning to understand user preferences and context more deeply. This will enable AI systems to provide highly tailored responses, thereby improving user satisfaction and engagement.
Integrating text, image, and audio data allows AI models to generate comprehensive and context-aware responses. For example, AI models like OpenAI’s GPT-4 and Google’s BERT can analyze and describe images in detail, accurately transcribe spoken language, and generate contextually relevant text across different media formats. Future advancements will likely refine and expand these functionalities, making AI better at handling complex, multimodal data and developing nuanced, highly context-aware responses.
Moreover, as tools for prompt engineering and few-shot learning become more user-friendly, a broader range of individuals and organizations can exploit their power. This democratization will lead to more diverse and innovative applications of AI across various fields.
However, with AI’s increasing capabilities, ethical considerations will become even more important. Ensuring that AI systems are transparent, fair, and aligned with human values will be critical. Techniques like prompt engineering can guide AI models towards ethical behavior, while few-shot learning can help adapt models to diverse cultural contexts and languages, ensuring that AI serves humanity in a responsible and beneficial manner.
The Bottom Line
From prompt engineering to few-shot learning, the techniques for enhancing AI model responses are continually evolving. These advancements are improving the accuracy and relevance of AI-generated content and expanding the potential applications of AI across various domains. As we look to the future, these techniques’ continued development and refinement will undoubtedly lead to even more powerful and versatile AI systems capable of transforming industries and enhancing our everyday lives.