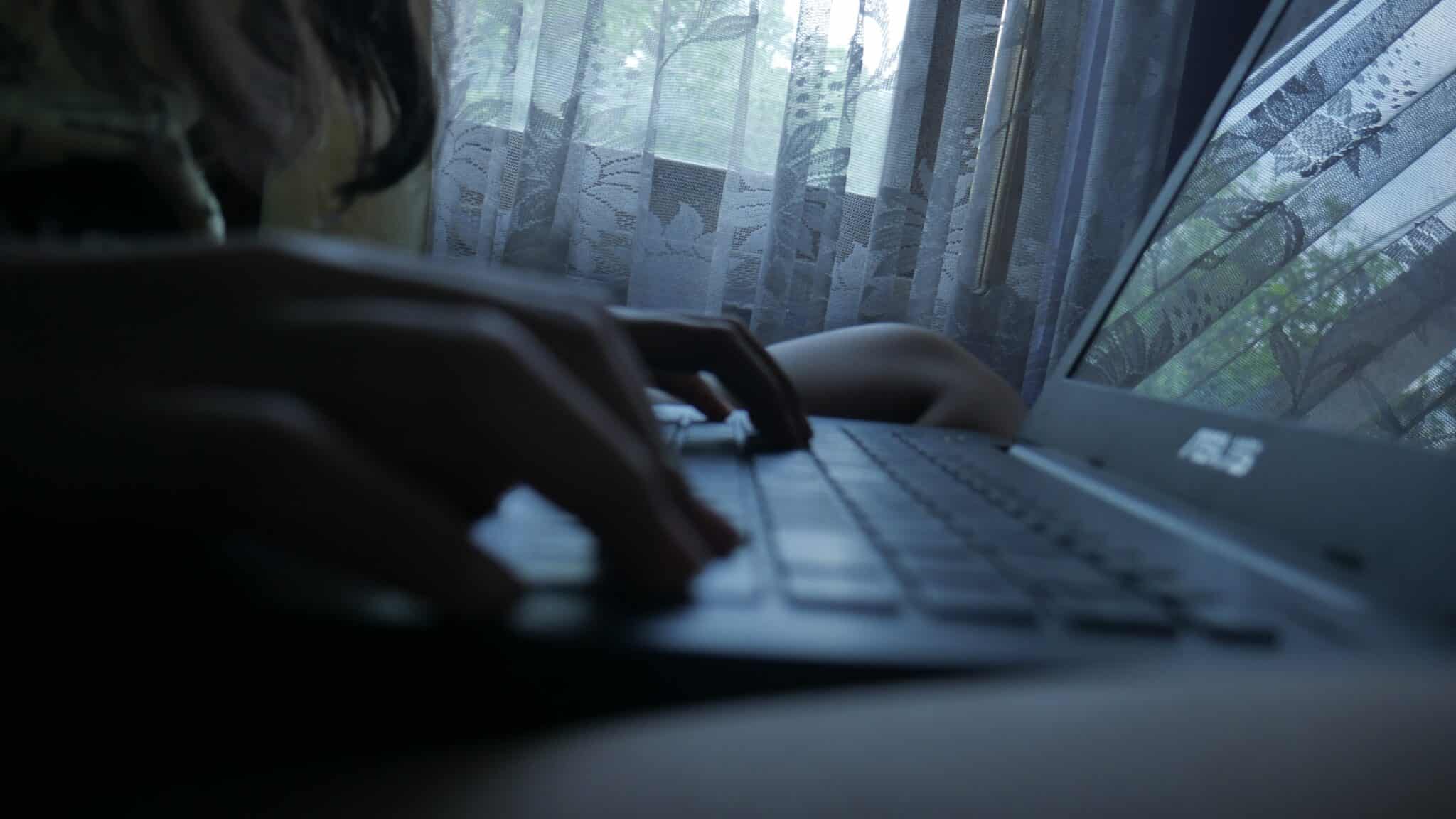
By Ola Ahmad, Chief AI Scientist at Thales
The rise in innovative applications for emerging tech such as artificial intelligence/machine learning (AI/ML) and Large Language Models (LLMs) has also opened the doors to new risks and vulnerabilities. One such vulnerability, called a “prompt injection,” is affecting AI/ML apps and LLMs, and aims to override the model’s existing instructions and elicit unintended responses.
Some quick background: The “prompt” here is the set of instructions that is either built in by developers or inserted by users telling an LLM and its integrated application what to do. On its own, this isn’t a threat, but bad actors can manipulate and inject malicious content into prompts to exploit the model’s operating system. For instance, hackers can trick LLM applications like chatbots or virtual assistants into ignoring system guardrails or forwarding private company documents.
Prompt injection attacks on organizations don’t usually go for user identities. However, if an attacker aims to manipulate the model in ways that could expose personal or confidential information, the consequences could indirectly compromise the user’s identity, risking everyone from intern to CEO.
LLMs and AI/ML apps are being increasingly exploited by hackers to falsify identities and scam people or organizations. With prompt injections, hackers can craft specific prompts to trick the model into giving unauthorized access to or leaking personal data, extracting sensitive personal information, and generating misleading or harmful outputs for phishing or impersonation. Once they have a hold of personal data, attackers can engage in identity theft or fraud, further compromising the user and potentially others dependent on them.
Combating prompt injection attacks
There are several ways an organization can secure its AI model and protect the identity of its users. In addition to using security tools and frameworks, the average organization should follow three best practices: human-in-the-loop verification, explainability, and AI models and techniques for detecting and mitigating suspicious content.
Human-in-the-loop verification
The “human-in-the-loop” concept involves human oversight and intervention of automated processes to mitigate errors, monitor for suspicious activity, ensure accuracy, and maintain ethical standards. AI is still prone to bias and error, not yet at the level of human cognitive abilities, and integrating the human touch helps the organization deliver nuanced solutions and decisions that AI alone can’t fully achieve just yet.
Prompt engineers can use human-in-the-loop approaches to review AI responses and ensure they meet human expectations. Humans can provide feedback and quality control, determining if AI systems are relevant and adapting to new trends and information. Tasks such as editing files, changing settings, or calling APIs typically require human approval to maintain control and increase overall LLM security.
However, using LLMs with human oversight involved makes them less convenient and more labor-intensive. In addition, humans are prone to error, and involving human oversight does not guarantee complete security. Sometimes, the malicious prompts and hack attacks are sophisticated enough that they can slip past human monitoring. For instance, attackers can use social engineering to exploit users into giving away personal information, like their social security or credit card numbers. They can also “prompt” the LLMs or AI/ML applications to release sensitive data used for identification and authentication – and before we know it, the targeted user is a victim of identity theft.
Still, human oversight can help recognize and flag suspicious activity, mitigating the chances of bad actors successfully injecting malicious prompts and compromising an individual’s identity and security.
Explainability
Explainability is the concept that an AI model and its output can be explained in a way that “makes sense” to a human being, making complex AI decisions transparent and trustworthy. Using explainability to combat prompt injection attacks can enhance an organization’s understanding of how models process inputs and generate outputs. Explainability can involve several defense strategies that fall under four approaches:
- Identify: Enterprises can deploy tools that can identify how a model reaches certain responses, detect anomalies or unusual patterns at the input-output level or within the model, and indicate potential attempts at prompt injections. Users should be able to give feedback on model outputs, flagging if they identify unusual responses.
- Educate: This can include providing users with clear guidelines on interacting with the LLM and training various company teams to understand appropriate inputs and outputs so they are more aware and can respond more quickly to potential attacks.
- Analyze: Scrutinizing the inputs that lead to unexpected outputs of the model will determine the causal relationship involved. Regularly auditing the model can also help document its responses and build a dataset for analysis.
- Refine: The analytic insights can be used to adjust training data and strategies to iteratively refine the LLM. The model can also incorporate explainable AI methods to better interpret model decisions and improve robustness against bad prompts.
Explainability gives transparency and clarity into how prompt injection attacks might work so enterprises can strengthen their attack surface. Beyond enhancing security, it also fosters trust in the model’s reliability to keep personal information safe.
AI techniques for prompt injection detection and mitigation
Apart from explainable AI, there are several techniques that companies can employ to enhance their system’s overall security. To get started, they should first identify key objectives and which AI model can fulfill them, before moving toward adoption.
When it comes to securing user identity against malicious prompt injections, the organization can deploy techniques like natural language processing (NLP), anomaly detection, computer vision, and multimodal capabilities to analyze and filter user inputs in real-time, improve identity verification, and flag potentially malicious content based on context and semantics. So, if someone is using fraudulent visual IDs, computer vision may be able to scan, detect, and signal the injection attempt. Furthermore, multimodal models can identify unusual patterns that do not align consistently across different modalities such as text prompts, images, and/or audio, signaling a potential injection attack.
AI techniques like contextual awareness, behavioral analysis, and robust testing can amplify explainability tactics to address suspicious or harmful prompts. Larger, more complex organizations can deploy an ensemble of models and multimodal methods, to evaluate inputs and outputs for a more robust prompt assessment and prompt injection detection.
While these do not guarantee that prompt injection attacks will be eliminated, by integrating these strategies, enterprises everywhere can significantly enhance the resilience of their systems against malicious injections. And by enhancing model robustness and security, enterprises can protect not just the integrity of the model, but also user data and identity.
About the author
Dr. Ola Ahmad is the Chief AI Scientist at the Thales Research and Technology facility in Canada, and an Adjunct professor at Laval University. Her expertise spans across analytical modeling, machine learning/deep learning, trustworthy artificial intelligence, signal processing and computer vision.
Dr. Ahmad earned her Ph.D. in computational modeling and geometry from the École Nationale Supérieure des Mines de Saint-Étienne in France in 2013, where her research focused on the geometry of random fields and probabilistic modeling of stochastic patterns. Following her doctorate, she held postdoctoral positions at several academic institutions including the University of Strasbourg (France), University of Sherbrooke (Canada), and Polytechnique Montreal (Canada), where she further specialized in deep learning and hybrid AI applied to computer vision, sensing and robotics.
In 2018, Dr. Ahmad joined Thales’ Research and Technology team in Canada, where she currently leads the research roadmap for trustworthy AI, spearheading the development of explainable AI, robust machine learning, and frugal/embedded deep learning solutions for autonomous and safety-critical systems.
Article Topics
explainability | injection attacks | prompt injection | research and development | responsible AI | Thales
Latest Biometrics News
Oct 21, 2024, 12:16 pm EDT
The Authenticate 2024 conference hosted by the FIDO Alliance was focused mainly on passkeys; their adoption, benefits and fit with…
Oct 21, 2024, 9:42 am EDT
Bahrain’s Vision 2030 is to become the global leader in digital identity services and a cashless society. The government believes…
Oct 20, 2024, 8:09 pm EDT
More than half a century ago, American psychologist Paul Ekman wanted to know whether he could tell if a person…
Oct 20, 2024, 1:38 pm EDT
Human rights organizations are expressing support for the U.S. government’s plans to introduce new export controls on facial recognition systems…
Oct 20, 2024, 1:18 pm EDT
As part of broader efforts to digitize Cameroon’s civil registration and vital statistics (CRVS) system, the country is launching launch…
Oct 20, 2024, 12:58 pm EDT
Riga’s role in driving digital transformation was highlighted at the recent Latvian-German local government forum held in Berlin. Linda Ozola,…