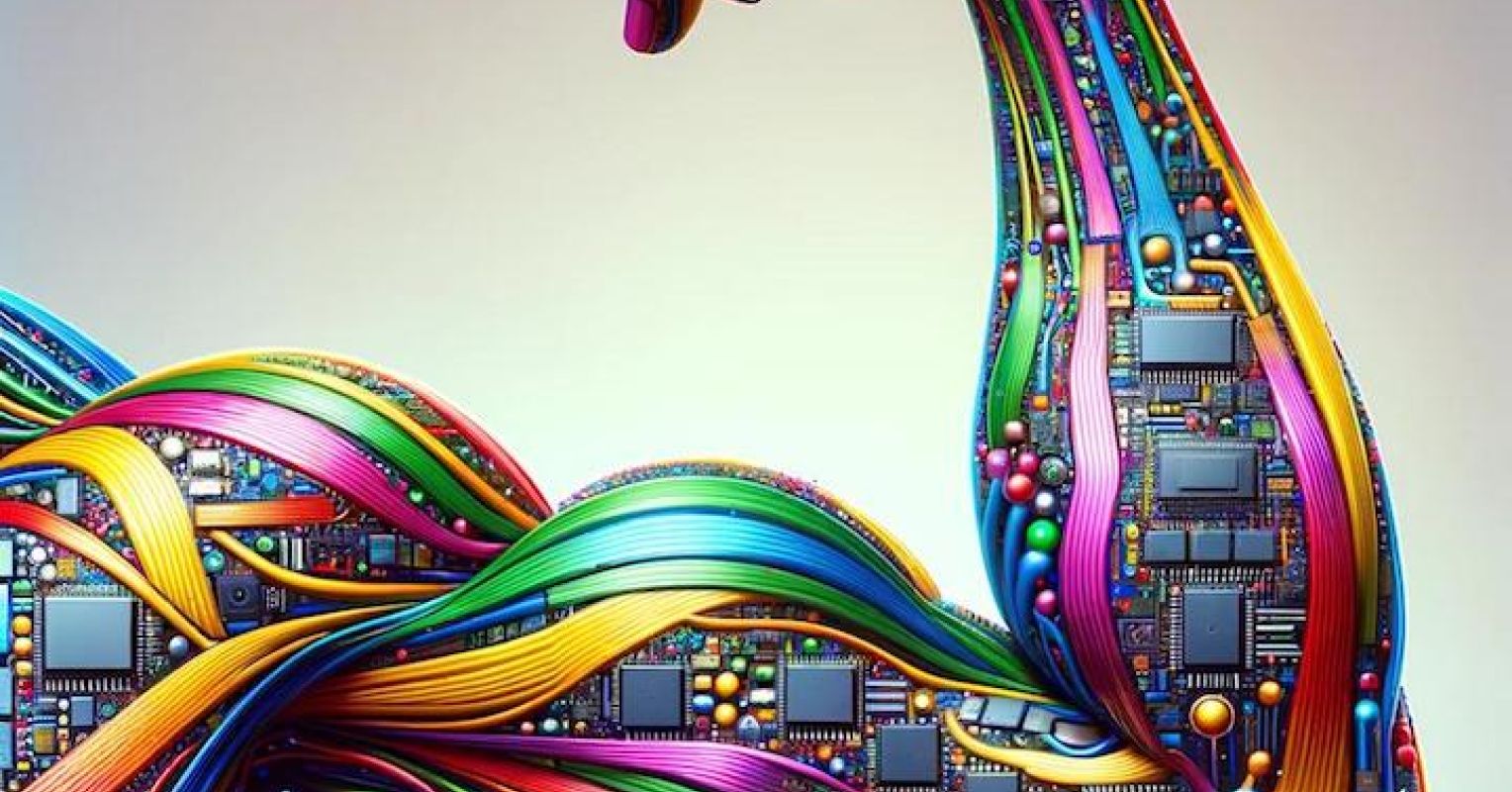
Source: Art: DALL-E/OpenAI
Data and model training are at the heart of the competition with large language models (LLMs). But a compelling narrative is unfolding, one that could very well redefine our approach to crafting artificial intelligence (AI) solutions tailored to specialized domains like finance and medicine.
The protagonists of our story? The generically trained, such as GPT-4 and Claude 3 Opus, are now being benchmarked against the “age-old” practice of fine-tuning models for domain-specific tasks.
The financial sector, with its intricate jargon and nuanced operations, serves as the perfect arena for this showdown. Traditionally, the path to excellence in financial text analytics involved fine-tuning models with domain-specific data, a method akin to giving a neural network a crash course in finance. However, a study from last year suggests a different story. And with the current rapid progress on “generic” models, this may be very important from performance and financial perspectives.
The Untrained Titans
Imagine, if you will, a world where an AI model not explicitly trained on financial data can navigate the complex labyrinths of financial text analytics, outperforming its fine-tuned counterparts. This isn’t a fragment of sci-fi imagination anymore. GPT-4, with its vast, generalist training, is making this a reality. And that’s just the beginning. Claude 3 Opus is gaining momentum, and the pending launch of GPT-5 further supports this trend.
These models, trained on a diverse array of internet text, have shown an astonishing ability to grasp and perform tasks across various domains, finance included, without the need for additional training. It’s as if they’ve absorbed the internet’s collective knowledge, enabling them to be jacks of all trades and, surprisingly, masters, too.
The Fine-Tuning Conundrum
Fine-tuning has been the go-to for achieving peak performance in domain-specific tasks. By tailoring a model to understand the subtleties of financial language, one could expect it to excel in tasks ranging from sentiment analysis to complex question-answering. However, this approach comes with its own set of challenges. The need for domain-specific datasets, the computational resources for training, and the risk of overfitting to a particular domain are but a few hurdles on this path.
An Empirical Verdict
This 2023 study has tested these models across a variety of financial text analytics tasks, from sentiment analysis to question-answering. The results? ChatGPT and GPT-4 not only held their own but, in many cases, outshone the fine-tuned models. Particularly noteworthy is the GPT-4 performance, which showcases significant improvement over ChatGPT across nearly all financial benchmarks. This leap in capability suggests that as these LLMs evolve, their need for domain-specific fine-tuning may diminish.
Prompting Power
Beyond the raw computational prowess and expansive knowledge of the latest large language models, the art and science of prompting emerge as a pivotal layer in unlocking their full potential. The nuanced craft of prompt engineering transforms the way we harness these digital titans, bridging the gap between human ingenuity and AI’s vast capabilities. This synergy between sophisticated human prompts and the model’s strengths introduces a collaborative dimension to AI interaction, where the precision of the prompt dictates the relevance and depth of the model’s response. As we refine our ability to communicate with these models through prompts, we’re not just leveraging AI; we’re engaging in a dynamic partnership that amplifies our collective intelligence, marking a significant leap forward in our journey with artificial intelligence.
Implications and Musings
What does this mean for the future of AI in specialized domains? Are we approaching a point where the flexibility and general prowess of LLMs could reduce the necessity for fine-tuning? This prospect is both exciting and a bit unsettling. On the one hand, the ability to deploy highly capable AI models without extensive domain-specific training could democratize AI, making powerful tools more accessible across various fields, from finance to medicine. On the other, it raises questions about the future of custom model development and the unique value it offers, particularly in the context of new studies and real-time data.
Technological Muscle Flexing
In this next step in AI’s evolution, the rapid advancements in LLMs stand as a testament to the breakneck speed of progress in the field, edging us ever closer to the tantalizing horizon of artificial general intelligence (AGI). As these models, brimming with the potential of human-like understanding and capability, flex their technological muscles, we find ourselves at the cusp of what could be a defining moment for LLMs. The journey towards AGI, marked by this spectrum of advancements, promises to be transformative, reshaping our interaction with technology and establishing the “power era” of intelligent machines.