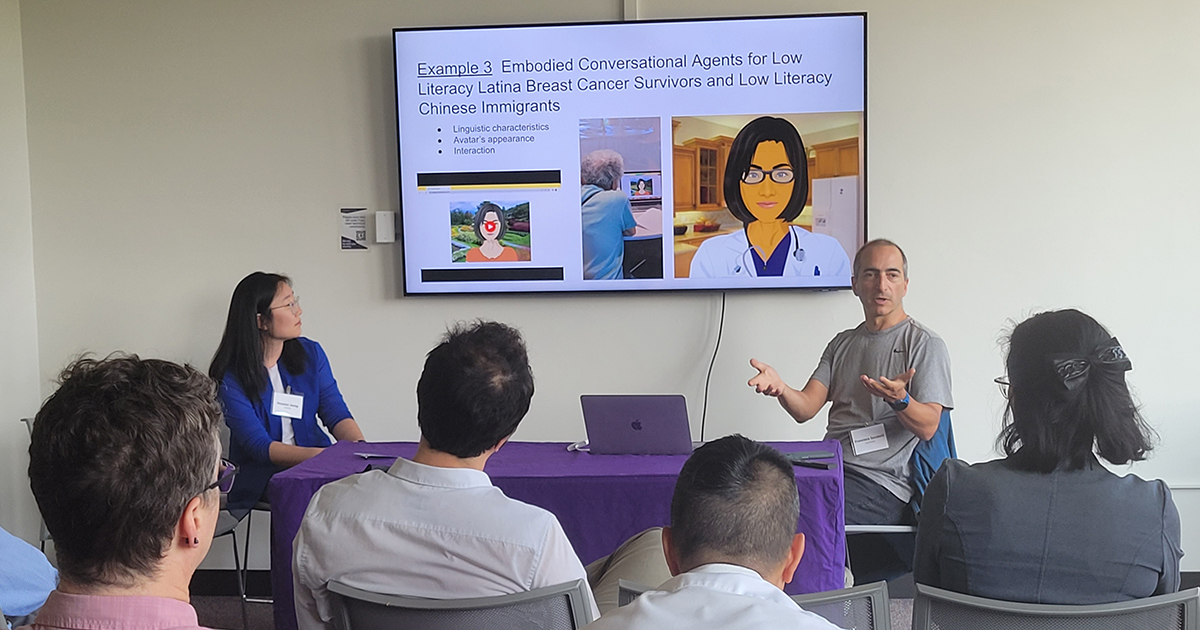
Artificial intelligence (AI) technologies are revolutionizing healthcare, from detecting cancer to helping a paralyzed man walk again. Now, medical professionals are beginning to assess how chatbots like ChatGPT could change the way they work.
Large language models (LLMs) were the focus June 7 of the AI@NU, Institute for Augmented Intelligence in Medicine (I.AIM), and Northwestern Engineering event, “Exploring Opportunities at the Intersection of Healthcare and AI.” Physicians and computer scientists gave three separate demonstrations showing how people can use AI to augment certain tasks, such as organizing patient data.
“When people go to a university, they are excited to meet with the students and the faculty who are conducting novel research,” said Abel Kho, director of I.AIM and the Institute for Public Health and Medicine (IPHAM)’s Center for Health Information Partnerships. “That is why universities are great places for this type of cross-institutional innovation.”
Speakers at the event emphasized an interdisciplinary approach and encouraged participants to connect with others across campus.
“You need problems to challenge you, and if the only problems you have are the ones you think up on your own, those will not be challenging problems,” said Kristian Hammond, Bill and Cathy Osborn Professor of Computer Science at the McCormick School of Engineering and director of the Center for Advancing Safety of Machine Intelligence (CASMI). “You need problems that are real-world and are impactful. If you come up with a solution, maybe you could help save a life.”
Participants were split into three groups to hear every presentation before they reconvened to discuss the benefits and the risks of generative AI.
Demo #1: Augmented Medicine Proof of Concept – Infectious Diseases AI-Assistant
The first demonstration focused on how large language models like ChatGPT can be used to assist infectious disease specialists, who diagnose and manage diseases caused by viruses, bacteria, fungi, and parasites.
Alex Carvalho, infectious disease fellow at Northwestern’s Feinberg School of Medicine, gave ChatGPT information about a real clinical case his team saw: a 39-year-old man who suffered a gunshot wound to the spine and developed a fever and infection. ChatGPT recommended antibiotics immediately, but Carvalho said it’s best to wait to prescribe.
“This is the first time we see it’s not quite ready for gametime, and I still have a job hopefully,” Carvalho said. When he gave ChatGPT more information stating the patient had bullet fragments in his body, the chatbot then said it was best to wait to prescribe antibiotics.
Developer tools such as application programming interfaces (APIs) allow users to better guide ChatGPT. David Liebovitz, co-director of I.AIM and the Center for Medical Education in Digital Healthcare and Data Science, showed how someone can ask ChatGPT to read a PDF before it generates answers based on what’s in the document.
ChatGPT is not reliable for providing citations. It often hallucinates, or confidently presents erroneous information. To avoid this issue, Liebovitz created a template that allowed ChatGPT to provide a link to PubMed, a medical database, when he needed a reference.
“Rather than asking it to provide citations directly, instead ask it to create the link,” Liebovitz said.
Demo #2: Truth-based LLMs
The second presentation demonstrated how, with proper steering, language models can be prompted to produce factual information that is grounded in real-world data. Marko Sterbentz and Cameron Barrie, computer science PhD students who are part of the C3 Lab, configured a system that allows medical practitioners to easily gain insight from emergency department admissions data.
The students created an analytics engine, which generates factual statements about the data. When a user asks the system a question, it runs through the analytics engine before the language model generates a written report.
“We are not trusting the language model to do any calculations,” Barrie said. “We are doing all the analytics within our local system, and then the model is using that information to produce the report.”
“The notion here is that we can take this well-trodden technology, generate all these facts, which then get fed into the language model,” Hammond said. “The language model will provide the structure and the fluency, but the facts themselves are coming from the data.”
Next, the students plan to expand the system’s capabilities, including developing an automated fact-checking method. They are also working to explore other data domains beyond healthcare.
“The ultimate hope with this work is to make it possible to generate well-written documents with highly reliable information that communicates impactful insights about any given data so that people can better utilize data to improve their work and improve the lives of people,” Sterbentz said.
Demo #3: Thinking about LLMs in the Context of Health Disparities
The third demonstration illustrated the benefits of designing robots or avatars specifically for different populations. For example, CASMI visiting researcher Francisco Iacobelli, associate professor of computer science at Northeastern Illinois University, worked with low-literacy Latina breast cancer survivors to build an intelligent tutoring system that helps patients understand their health. He also worked with low-literacy Chinese immigrant patients to design an agent that could have conversations with people in Cantonese.
“How they look affects the interaction,” Iacobelli said. “We had to refine the look with people from the community so that patients would trust the agent and engage visually with the agent.”
Sooyeon Jeong, postdoctoral fellow at the Center for Behavioral Intervention Technologies, helped develop two social robots: one for pediatric patients and another for college students. Both were designed to improve mental health but in different ways. The robot for pediatric patients was a playful teddy bear that a child specialist controlled, and the one for college students led therapeutic exercises.
“Based on the target populations, there are different kinds of language and interactions you have to provide and design around,” Jeong said.
The demonstration concluded with an activity: comparing how ChatGPT and Google Bard can explain lymphedema to a fifth grader. Event participants received different responses from the language models but noted that Bard listed bullet points while ChatGPT provided a metaphor.
The Takeaways
Speakers at the event said it’s important to understand the limitations of language models. Hammond said they are “fluency engines” that are very good with language but not with reasoning.
Some expressed optimism that chatbots will give them more time to connect with patients. If patients use ChatGPT, they may develop a better understanding of their health.
“It may not be perfectly right, but it’s going to prompt conversations,” Kho said. “If you have engaged patients and engaged populations, potentially you raise the bar so that everyone is at a higher level and is effectively communicating in a meaningful way.”