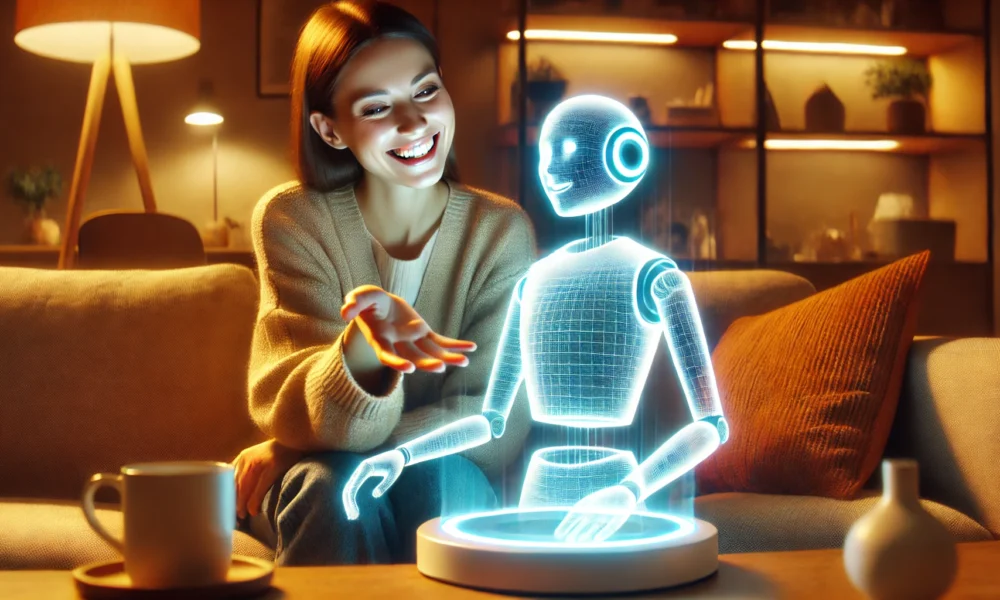
The proliferation of LLMs like OpenAI’s ChatGPT, Meta’s Llama, and Anthropic’s Claude have led to a chatbot for every occasion. There are chatbots for career advice, chatbots that allow you to speak to your future self, and even a chicken chatbot that gives cooking advice.
But these are not the chatbots of ten years ago – back then, they were limited to narrowly preset, rigid “conversations,” often based on a large flow chart with multiple choice or equivalent responses. In essence, they were only slightly more sophisticated than pre-internet IVR telephone menus.
Today’s “chatbots,” on the other hand, are more frequently referring to conversational AI, a tool with much broader capabilities and use cases. And because we now find ourselves in the midst of the generative AI hype cycle, all three of these terms are being used interchangeably. Unfortunately, as a consequence there are many misunderstandings around the risks, use cases, and ROI of investing in conversational AI amongst business leaders, especially in highly regulated industries like finance.
So I’d like to set the record straight on some common misunderstandings around “chatbots,” when what we’re really discussing is conversational AI.
Myth 1: Customers Hate Chatbots
Consumers have been asked for the better part of the last decade whether or not they prefer human agents or chatbots – which is like asking someone if they’d rather have a professional massage or sit in a shopping mall massage chair.
But the debut of ChatGPT in 2022 (along with all the tools that spun from it) turned our perception of a chatbot’s capabilities entirely on its head. As mentioned above, older chatbots operated on scripts, such that any deviation from their prescribed paths often led to confusion and ineffective responses. Unable to understand context and user intent, the answers given were often generic and unhelpful, and they had limited capacity to gather, store, and deliver information.
In contrast, conversational AI engages people in natural conversations that mirror human speech, allowing for a more fluid, intuitive exchange. It demonstrates remarkable flexibility and adaptability to unexpected outcomes. It’s able to understand the context surrounding user intent, detect emotions and respond empathetically.
This deeper level of understanding enables today’s AI to effectively navigate users down logical paths towards their goals. That includes quickly handing customers off to human assistants when necessary. Moreover, conversational AI uses advanced information filters, retrieval mechanisms, and the ability to retain relevant data, significantly enhancing their problem-solving abilities, which makes for a better user experience.
So, it’s not that customers blindly hate chatbots, what they hate is bad service, which previous versions of chatbots were definitely guilty of delivering. Today’s conversational agents are so much more sophisticated that over a quarter of consumers don’t feel confident in their ability to differentiate between human and AI agents, and some even perceive AI chatbots to be better at selected tasks than their human counterparts.
In test pilots, my company has seen AI agents triple lead conversion rates, which is a pretty powerful indication that it’s not about whether or not it’s a bot – it’s about the quality of the job done.
Myth 2: Chatbots are Too Risky
In discussions with business leaders about AI, concerns often arise around hallucinations, data protection, and bias potentially leading to regulatory violations. Though legitimate risks, they can all be mitigated through a few different approaches: fine tuning, Retrieval-Augmented Generation (RAG), and prompt engineering.
Though not available on all LLMs, fine-tuning can specialize a pre-trained model for a specific task or domain, resulting in AI better suited to specific needs. For example, a healthcare company could fine-tune a model to better understand and respond to medical inquiries.
RAG enhances chatbot accuracy by dynamically integrating external knowledge. This allows the chatbot to retrieve up-to-date information from external databases. For instance, a financial services chatbot could use RAG to provide real-time answers about stock prices.
Lastly, prompt engineering optimizes LLMs by crafting prompts that guide the chatbot to produce more accurate or context-aware responses. For example, an e-commerce platform could use tailored prompts to help the chatbot provide personalized product recommendations based on customer preferences and search history.
In addition to using one or more of these approaches, you can also control a conversational AI’s creativity “temperature” to help prevent hallucinations. Setting a lower temperature within the API calls limits the AI to providing more deterministic and consistent responses, especially when combined with a knowledge base that ensures the AI draws from specified, reliable datasets. To further mitigate risks, avoid deploying AI in decision-making roles where bias or misinformation could lead to legal issues.
As for data privacy, ensure that external AI providers comply with regulations, or deploy open-source models on your own infrastructure in order to retain full control over your data, essential for GDPR compliance.
Finally, it’s always wise to invest in professional indemnity insurance that can offer further protection, covering businesses in unlikely scenarios such as attempted litigation. Through these measures, businesses can confidently leverage AI while maintaining brand and customer safety.
Myth 3: Chatbots aren’t ready for complex tasks
After seeing the issues big tech companies are having deploying AI tools, it may feel naive to think an SME would have an easier time. But AI is currently at a stage where the phrase “jack of all trades and master of none” isn’t terribly inaccurate. This is largely because these tools are being asked to perform too many different tasks across environments that are not yet designed for effective AI deployment. In other words, it’s not that they’re not capable, it’s that they’re being asked to figure skate on a pond full of thin, fractured ice.
For example, organizations rife with siloed and/or disorganized data are going to be more prone to AI surfacing outdated, inaccurate, or conflicting information. Ironically, this is a consequence of their complexity! Whereas older chatbots were simply regurgitating basic information in a linear fashion, conversational AI can analyze robust datasets, considering several influential factors at once in order to chart the most appropriate path forward.
Consequently, success with conversational AI is contingent on strict parameters and extremely clear boundaries regarding data sources and tasks. With the right training data and expertly designed prompts, the functionality of conversational AI can extend far beyond the scope of a simple chatbot. For example, it can gather and filter data from customer conversations and use it to automatically update a CRM. This not only streamlines administrative tasks, but also ensures that customer information is consistently accurate and up-to-date. By automating such tasks, businesses can focus more on strategic activities rather than administrative burdens.
If we’re going to continue using the term “chatbot,” it’s imperative that we differentiate between platforms that are incorporating cutting edge conversational AI, and those that are still offering the limited tools of yesterday. In the same way that today the word “phone” more often elicits the image of a touch-screen smartphone than a spiral-corded landline, I believe we’re not far from “chatbot” being replaced by the idea of advanced AI agents rather than clunky multiple-choice avatars.